Three questions for businesses before they integrate AI in their operations
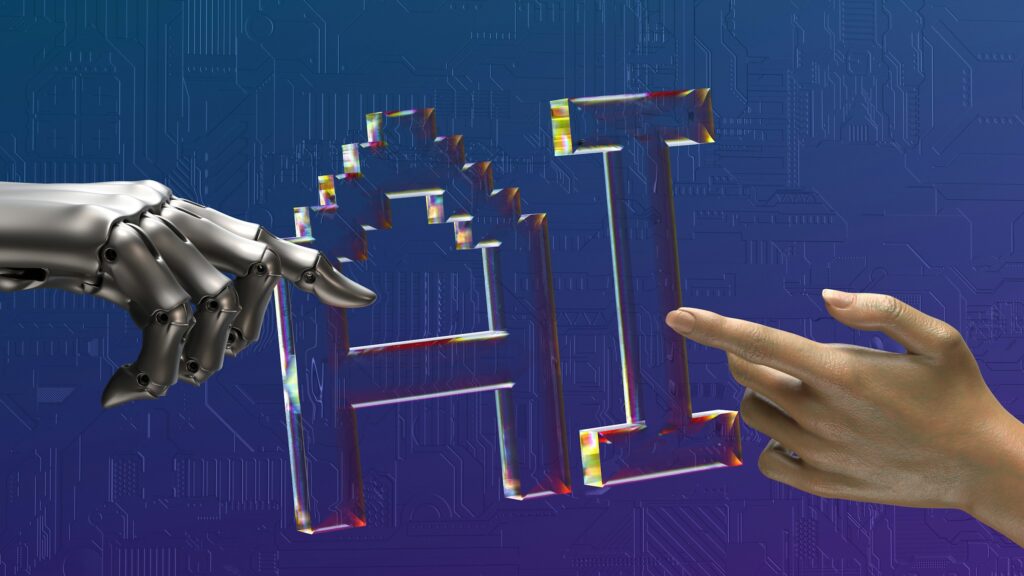
This article is featured on LSE Business Review, and is written by Terence Tse, David Carle, and Danny Goh.
With the hype around large language models such as ChatGPT, many firms are rushing to adopt the technology and integrate it in their operations. However, businesses should be cautious. Not all AI is created equal. Terence Tse, David Carle and Danny Goh pose three questions to guide companies in their AI adoption decisions.
To stay ahead of the competition, you have integrated artificial intelligence (AI) into your company. However, are you sure you have truly harnessed technology’s potential, or have you inadvertently stumbled into empty hype? Have you got the AI right or, more importantly, the right AI?
Considering the potential hazards that come with AI integration, we encourage businesses to answer three important questions prior to embracing this technology. By contemplating these questions in advance, companies can gain valuable insights into the potential advantages and drawbacks of implementing AI.
Is output accuracy a priority?
In the realm of “traditional AI”, or non-ChatGPT-type AI, accuracy serves as a beacon that guides the creation of effective AI solutions. However, with generative AI, typified by its ability to mimic human-like creativity, accuracy as a performance evaluation metric has seemingly lost its vital role. It is easy to fall for the allure of generative AI’s human-like touch, causing us to prioritise experiential value over strict accuracy.
By way of comparison, this is similar to desiring a string of dates without valuing the quality of the interactions. However, such preference can be risky, considering that generative AI has a reputation for generating deceptive content called hallucinations. This makes AI outputs unreliable, which can lead to wasted time and resources and expose companies to reputational and financial hazards.
From our front-row seats working with our clients, we have devised three methods to mitigate the hallucination problem. One method involves implementing a so-called “large language model (LLM) adaptor”, which relies on the use of multiple AI models rather than solely depending on a single one. This specific adaptor ensures effortless compatibility between different LLMs and empowers these models to select the route that will deliver the best accuracy and performance.
Incidentally, this also serves as a back-up plan. If, for instance, one of the LLMs has suddenly stopped working, the AI solution can continue to function on the feed from other models.
Another method is to implement a technique called “retrieved augmented generation”. This option involves imposing certain restrictions on the generative AI model that can lead to more precise output, while also mitigating any instances of distorted information.
The third approach is to obtain the ability to track the origins of data.
Can your AI system trace the origins of data?
Picture a scenario where the HR team in your company recruits individuals just relying on the credentials they assert, neglecting to verify their CVs. The team completely ignores objective evaluations and makes recruitment decisions solely based on personal preferences. Would you perceive it as odd, if not outright unjust? Yet, it is how we embrace generative AI. We place trust, sometimes without question, on the output of generative AI.
While we may not have a foolproof solution for problem, we can implement measures to guarantee the AI’s information is sourced reliably. For instance, there is a huge difference between a model that generates a figure and a model that produces the same figure along with the details of the data origin: the name of the report, the page number and the exact location on the page where the data sit.
Data traceability is no fantasy. In our previous client works, we have been able to trace the original materials 100 per cent of the time. We strongly believe that only this way we can instil full confidence in our clients to depend and rely on generative AI.
Is the data secured?
In AI integration, safeguarding client data is a non-negotiable priority. Companies need to be mindful of the risks of relinquishing control over sensitive data. This is often due to the fact that many AI vendors currently require their clients to use cloud-based solutions. This can be dangerous as it means that not only the client’s data, but often the data of the client’s client will also have to sit in the cloud. This can put all the parties involved at unnecessary risk.
A much better approach is to deploy AI solutions using clients’ on-premises data centres instead. However, this may introduce more complex development and implementation processes. Yet, we believe the long-term benefits far outweigh the short-term troubles. To make the solution development part easier and faster, it is possible to train, develop and test many AI models using just small or even synthetic datasets and then migrate them to on-premises systems of the clients.
While embracing AI promises unprecedented opportunities, careful considerations are paramount. Not all AI are created equal, and navigating the business AI landscape demands caution. By asking the right questions and prioritising accuracy, data traceability, and data security, companies can chart a course toward AI integration that is both safe and sound.
About the Author
Terence Tse: a professor of finance at Hult International Business School.
David Carle: US Managing Director at Nexus Frontier Tech.
Danny Goh: a partner and commercial director of Nexus Frontier Tech, a co-founder of the technology group Innovatube and an expert with the Entrepreneurship Centre at Said Business School, University of Oxford.