Winning Strategies for the High-Stakes AI Arena: Unveiling an Innovative AI Research and Development Planning Framework and Matrix
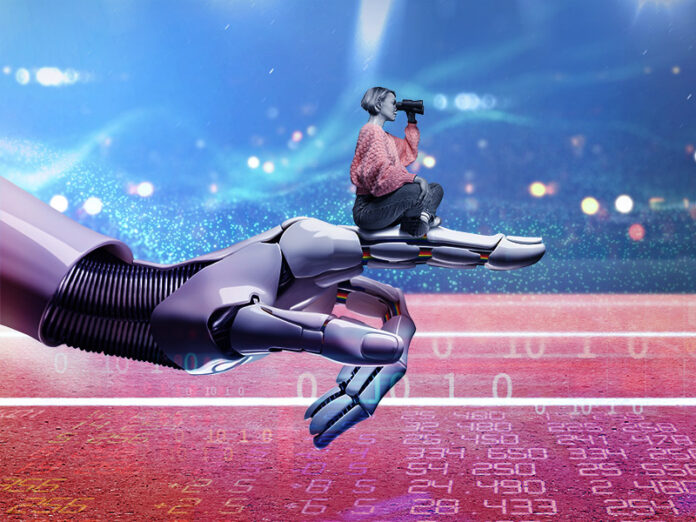
Image Credit | The European Business Review
This article is featured on The European Business Review and is written by Aurélie Jean, Mark Esposito, Terence Tse and Danny Goh.
Artificial intelligence and its rapid emergence have proven to be a double-edged sword. While its benefits are numerous, it also has the potential to bring grave consequences. In this essay, four experts from the fields of technology, AI, and digital transformation provide a framework for making the most of AI with low risks.
Today’s rapid emergence of AI for research and development (R&D) has opened up a myriad of use cases across different industries. We stand to gain the benefits of AI in general, and with the right level of project planning, we can deliver high business performance improvement. These are exciting times for AI. These are also times of contradiction: every story covering unprecedented successes is matched by other articles of concern over threats of unintended consequences. We want to develop AI to improve our lives. But we also want to protect ourselves from the bad things.
So, how can we build better tools to live better lives and use our tools to prevent life’s catastrophes? In the modern world, the very best time to do that is in research and development planning. We need ways to guide our innovative thinking and planning. Here, we are offering one such possibility.
The Recursive “More 3P” AI Transformation Framework
The definition of a recursive algorithm continuously seeks to break down the problem that the software is being asked to solve into smaller instances of the same problem. Instead of a solution to the problem, our proposed “More 3P” AI Transformation Framework intends to be a comprehensive disciplined approach to solution development.
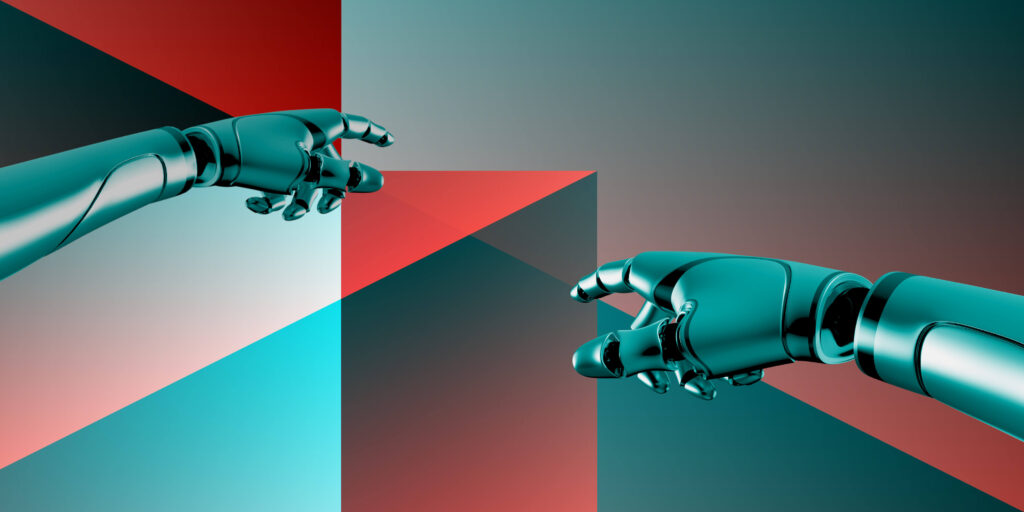
The “3P” refers to the three-dimensional realms considered in the R&D guidance: Prediction, Personalization, and Precision.
Prediction:
Predictive data analytics help ensure that organizations can, and in near-real-time, forecast conditions, trends, outcomes and behaviors more accurately, leading to informed proactive planning and decision-making. When considering how to apply AI in any given field, predictive simulations come first to mind. Indeed, by capturing the signals and patterns within the underlying mechanisms and logic of past events or a given phenomenon, algorithms can anticipate (or predict) a similar event in the near or long-term future.
In past decades, ever-better processing and collection of data have enabled real-time gathering and automated curation of datasets that can be used to train AI.
Of note is that one could pay attention to the model’s capability to predict unique, singular, or new events resulting from the simulations, either autonomously or with human subject matter experts (SMEs) oversight in the given field or industry sector.
Such “predictives” can also help identify threat risk potential hidden – for example technology discrimination and environmental impact – within the opacity of the algorithms driving the simulations, again with SME human oversight.
Personalization:
Personalization, on the other hand, indicates a potential for customizing services, products, and experiences that are tailored to individual users (consumers, customers, clients, and/or value-chain partners) based on demographics, preferences, and behavioral data to enhance product or service provision, satisfaction, and loyalty.
In past decades, ever-better processing and collection of data have enabled real-time gathering and automated curation of datasets that can be used to train AI. Additionally, up-leveling advances made on AI models make possible highly personalized recommendations or actual modifications of the delivery of products and services in general. Such increased capacity for personalization brings new business opportunities in improved design, production and delivery of more personalized products and services to customers.
How to Embrace the Recursive 3P AI Transformation Framework Approach
We expect More Predictive, More Personalized and More Precise business, work or production processes by employing formal design and systems thinking approaches that fully anticipate and consider AI transformation opportunities.
A use-case-driven approach should be used when adopting the 3P Frame by asking the following questions: What are the business transformation opportunities envisioned? What are the problems that can be anticipated? What are the threat risks?
We offer the More 3P AI Transformation Matrix, as depicted in Figure 1, which presents a list of all tasks for each of the three Ps. Here are the steps to building and using an AI Transformation planning matrix:
1. List all the tasks in which AI is already being, will be or can be applied;
2. For each task, list how this task can become more precise and/or more predictive, and/or more personalized;
3. Identify tasks that have substantially similar or common ways to more of each P dimension;
4. Identify tasks that have the most influence on precision, prediction and personalization to identify the tasks that will bring the most to the business;
5. Reiterate this matrix over time while moving forward to the AI transformation of the target process;
6. Tell the stories of what went right, what went wrong, and what came next.
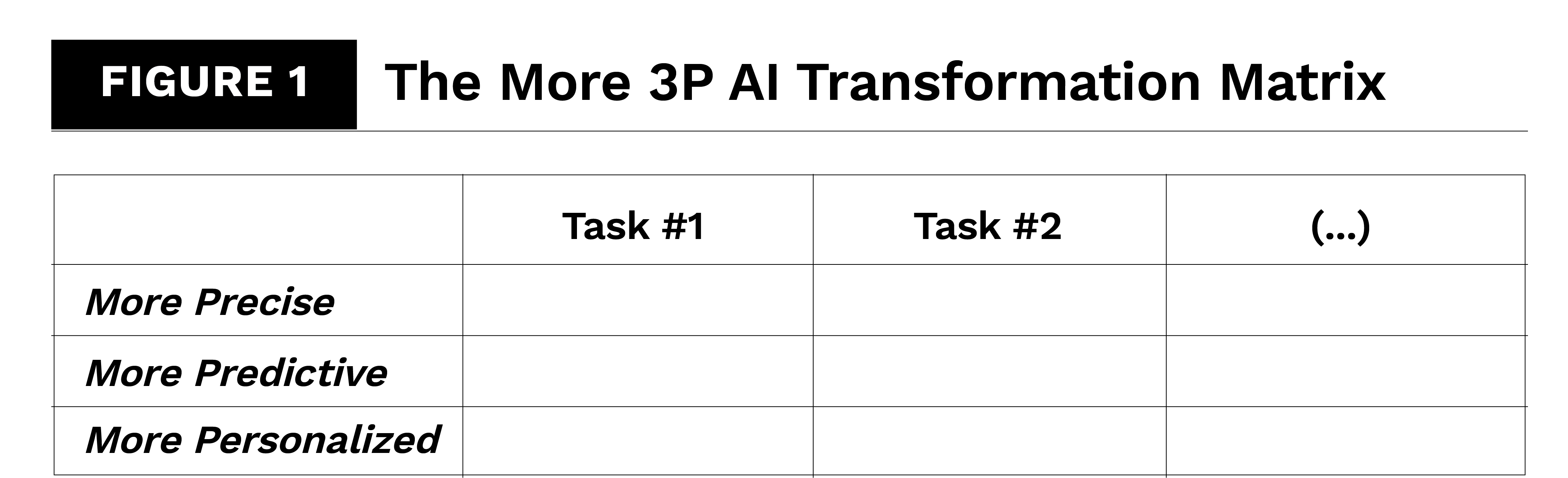
During the matrix planning process, it is useful to remember that this is simply an R&D guidance mechanism for model development. The simpler, the better. This enables development teams to actively and iteratively apply The More 3P Matrix to make ever-increasingly smarter, and more effective planning decisions.
The Promise of Business-Transforming Performance and Return on Investment
A simple framework and matrix means it can be more rigorously applied. In its simplicity, thoughtful detail, and rigor lies its power as an important planning tool. “More” performance improvement within the context of the 3P Framework Matrix may also mean improvement in planning for abeyance and mitigation of downside risk instances such as technology discrimination, for example, techno-racism, and algorithmic biases.
This is made possible by measuring the “more” quotient levels in each of the 3P realms and their evolutionary stages over time. Indeed, being more precise, more personalized and more predictive over baseline measures enforces project concepts as a matter of science and engineering.
The More 3P AI Transformation Framework is a proactive approach to continuous process improvement that voluntarily compels the full and willing participation of designers, developers (both data scientists and process engineers), and business-side leaders.
Technology development teams and business leaders must collaborate. They need to collaborate at every stage to design, develop, test, and operationalize. The underlying machine learning operations and/or generative artificial intelligence operations, as applied to the algorithmic models being developed, require business leadership to help ensure that the model’s aims, in terms of “More 3P AI,” meet all design criteria represented in the matrix.
If not, the systems may not run correctly with a resultant drag on business performance and return on investment for the company as a consequence. Poor governance may well result in the unintended consequences of imprecise (loose) AI model development. The More 3P AI Matrix, at its best application, prevents that.
Forward-Leaning
The More 3P AI Transformation Framework is a proactive approach to continuous process improvement that voluntarily compels the full and willing participation of designers, developers (both data scientists and process engineers), and business-side leaders.
This matrix must be planned and implemented with no-option fidelity as a “mission critical” matter to ensure these software systems meet stringent performance guidelines, specifications, and necessary policy and governance guardrails, all geared toward achieving true AI-led business transformation.
The More 3P AI Framework Matrix should likely lead the development of a business case for the proposed AI project models. This may apply particularly in mature corporate software development organizations. What makes it to the matrix and is fully listed and described will inform the business case criteria. For the AI start-up or early-stage venture, the 3P Framework Matrix may represent a clear, easy-to-understand way to introduce to all stakeholders what the business of the business is.
When pursuing AI Transformation within an organization, it’s useful to envision it through this Framework lens as it demonstrates how the organization can empower itself to take the best advantage of AI. The faster this can be fully articulated and demonstrated to leadership, the faster competitive advantage can be attained.
Furthermore, delineating how the business can evolve to become a More 3P organization within the business case cum an applied 3P AI Transformation Framework during the AI transformation provides a straightforward narrative of AI’s impact. This storyline goes beyond just the expected growth, profitability and efficiency performance gains. This narrative powerfully conveys AI’s business value, resonating with all stakeholders—employees, customers, value chain partners, and investors—by showcasing tangible business operations and enhancements to outcomes.
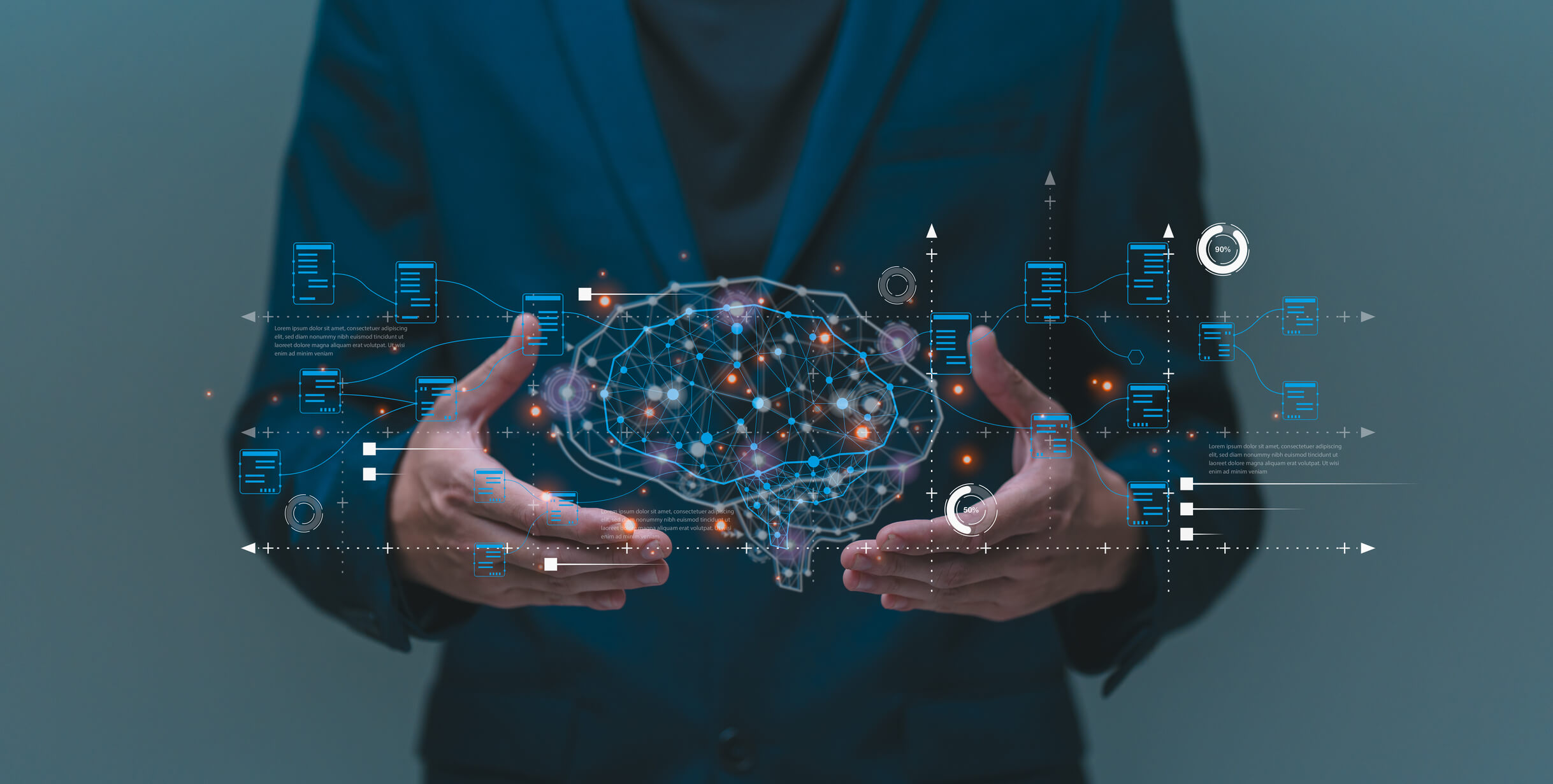
Embedding Effective Governance
Demonstrating near bullet-proof effective algorithmic governance in the 3P AI Transformation Framework is crucial as it addresses various challenges, such as data privacy, sovereignty, ethical considerations and guardrails, and regulatory compliance. By integrating algorithmic governance, the organization can mitigate risks associated with AI while optimizing its benefits. This governance should be dynamic, evolving with changing AI capabilities and societal norms to ensure that the transformation is innovative, continuously responsible, and sustainable.
Conclusion
A well-considered AI transformation course, viewed through the “3P” lens can confer substantial advantages for organizations. By rigorously applying 3P AI Transformation Framework strategies, organizations can realize significantly enhanced operational effectiveness and efficiencies, business performance improvement, ROI and competitive advantages – all governed by robust recursive algorithmic development and management practices that safeguard against potential risks.
Aurélie Jean, PhD, computational scientist, entrepreneur and author. Aurélie Jean has close to 20 years of experience in computational science applied to a broad range of disciplines. After 11 years of academic research, Aurélie is now running two companies, including a deep tech AI startup in the early detection of breast cancer. She is the author of several bestseller non-fiction titles on algorithmic science, as well as a columnist on science and technology. Aurélie is teaching algorithmic science in executive education and is a research fellow at the Hult Business School and The Digital Economist. She is also an investor and a board member of several companies in the United States and in France.
Dr. Mark Esposito is a professor of economics and public policy with appointments at Hult Int’l Business School and Harvard University. He equally serves as an Adjunct Professor of Public Policy at Georgetown University’s McDonough School of Business.
At Harvard, he serves as a social scientist with affiliations at Harvard Kennedy School’s Center for International Development; Harvard University’s Institute for Quantitative Social Science (IQSS); the Davis Center for Eurasian Studies and he is an incoming faculty affiliate of the Berkman Klein Center for Internet and Society at Harvard.
He co-founded the Machine Learning research firm, Nexus FrontierTech and the EdTech venture, The Circular Economy Alliance. He has equally co-founded The Chart ThinkTank and The AI Native Foundation. He was ranked by Thinkers50 in 2016 as one of the 30 rising business thinkers in the world and got shortlisted for the Breakthrough Award in 2019 and for the Strategy Award in 2023. He holds a doctoral degree from Ecole des Ponts Paris Tech and lives and works across Boston, Geneva, and Dubai.
Terence Tse is a globally recognized educator, author, and speaker. He is a Professor of Finance at Hult International Business School and co-founder of Nexus FrontierTech, an AI company. He is also a visiting professor at ESCP Business School and Cotrugli Business School. His latest co-authored book, The Great Remobilization: Strategies and Designs for a Smarter Global Future, was nominated for the 2023 Thinkers50 Strategy Award. Terence co-authored two Amazon bestsellers, The AI Republic and Understanding How the Future Unfolds. The DRIVE framework from the latter earned a nomination for the Thinkers50’s CK Prahalad Breakthrough Idea Award. Terence also authored Corporate Finance: The Basics, now in its second edition. He has appeared on numerous media platforms as well as ran workshops and consulted for global brands. He holds a doctoral degree from the Cambridge Judge Business School and has a background in investment banking and consulting.
Danny Goh is a serial entrepreneur and educator with a significant impact in the fields of innovation, sustainability, and digital transformation. He currently holds the position of Entrepreneurship Expert at the Saïd Business School, University of Oxford, and serves as a Senior Fellow at the Centre for Policy and Competitiveness at the École des Ponts Business School. Additionally, Danny lectures on entrepreneurship and digital transformation at various universities, including ESCP and Ecole des Ponts Business School, and provides his expertise as an advisor and judge for various technology start-ups and accelerators, including Microsoft Accelerator, Startupbootcamp IoT, and LBS Launchpad.
Danny is also a sought-after speaker, having presented at prominent conferences such as TEDx, UK High Commission events and BCGx. He co-authored the international best-seller on Amazon, The AI Republic: Building the Nexus Between Humans and Intelligent Automation, alongside Dr. Mark Esposito and Dr. Terence Tse. He is also a co-founder of the AI Native Foundation and The Chart Thinktank, a bipartisan non-profit organisation based in London. These organisations collaborate with global entities, including the United Nations and various governments, to advance the use of technology in the fourth industrial revolution.
As the founder and CEO of Nexus FrontierTech, Danny leads an AI research lab that leverages computer vision and NLP technologies to create digital transformation solutions for businesses across all sectors. The lab has successfully assisted Fortune’s top 100 enterprises like HSBC, UBS, MUFG and Toyota, as well as government bodies in the UK and Singapore, in integrating AI into their operations, thereby driving business growth and maintaining a competitive edge in an ever-evolving digital landscape.