AI Native Daily Paper Digest – 20250130
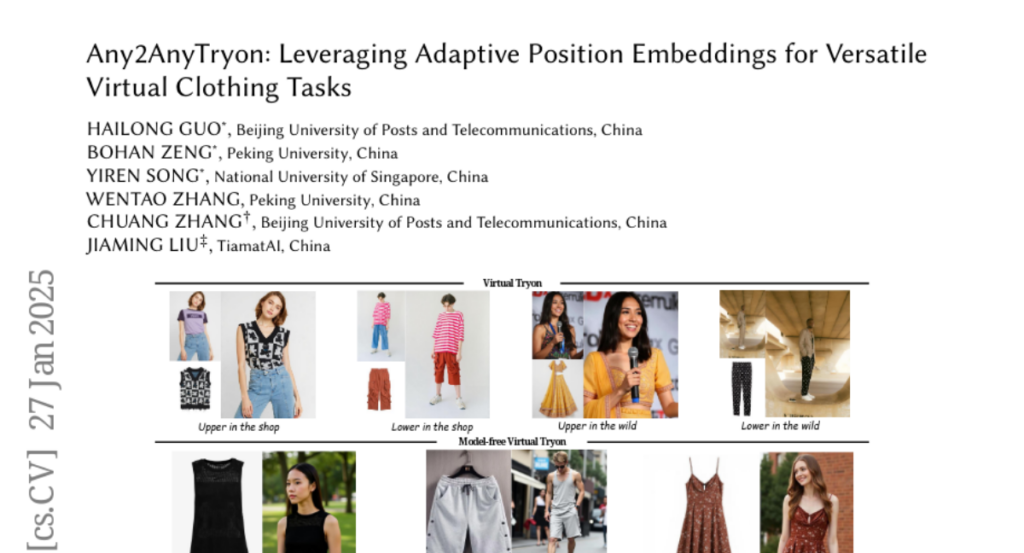
1. Critique Fine-Tuning: Learning to Critique is More Effective than Learning to Imitate
π Keywords: Supervised Fine-Tuning (SFT), Critique Fine-Tuning (CFT), GPT-4o, Qwen2.5-Math-CFT, Reasoning
π‘ Category: Natural Language Processing
π Research Objective:
– The study aims to challenge the existing paradigm of Supervised Fine-Tuning (SFT) by proposing a novel Critique Fine-Tuning (CFT) method for language models, inspired by human learning processes.
π οΈ Research Methods:
– The researchers constructed a 50K-sample dataset using WebInstruct and GPT-4o, where inputs include queries and noisy responses, and outputs are critiques. They tested CFT on various math benchmarks with models like Qwen2.5 and DeepSeek-Math, comparing its performance to SFT.
π¬ Research Conclusions:
– Critique Fine-Tuning (CFT) demonstrated a consistent 4-10% improvement over SFT in math benchmarks, and the Qwen2.5-Math-CFT model performed on par with or better than models trained on much larger datasets. The study confirms the robustness of CFT to the noise source and critique model, advocating that critique-based training enhances reasoning in language models.
π Paper link: https://huggingface.co/papers/2501.17703
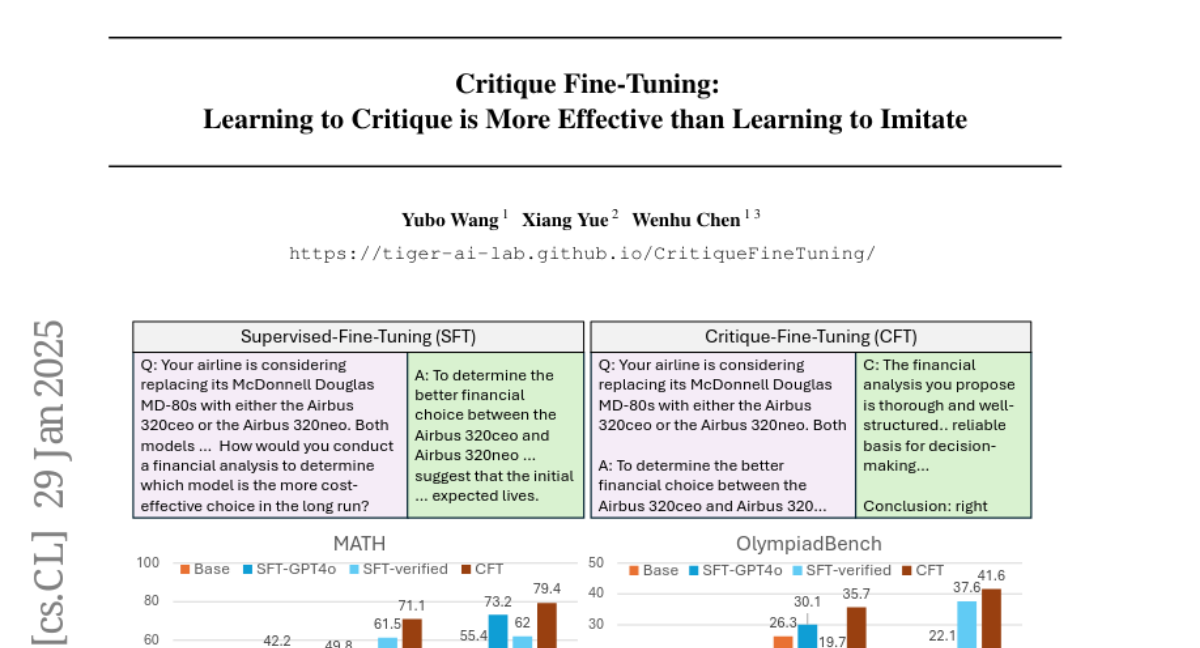
2. Atla Selene Mini: A General Purpose Evaluation Model
π Keywords: Small Language Model-as-a-Judge, Atla Selene Mini, Generative Model, HuggingFace, AI Native
π‘ Category: Generative Models
π Research Objective:
– To introduce Atla Selene Mini, a state-of-the-art small language model-as-a-judge (SLMJ) that excels in evaluating performance across diverse benchmarks.
π οΈ Research Methods:
– Developed a data curation strategy using synthetically generated critiques and dataset filtering. Trained the model with direct preference optimization (DPO) and supervised fine-tuning (SFT) loss.
π¬ Research Conclusions:
– Atla Selene Mini outperforms existing SLMJs and GPT-4o-mini in performance across 11 benchmarks. It shows improved zero-shot agreement with human evaluations in financial and medical datasets and is robust to prompt format variations.
π Paper link: https://huggingface.co/papers/2501.17195
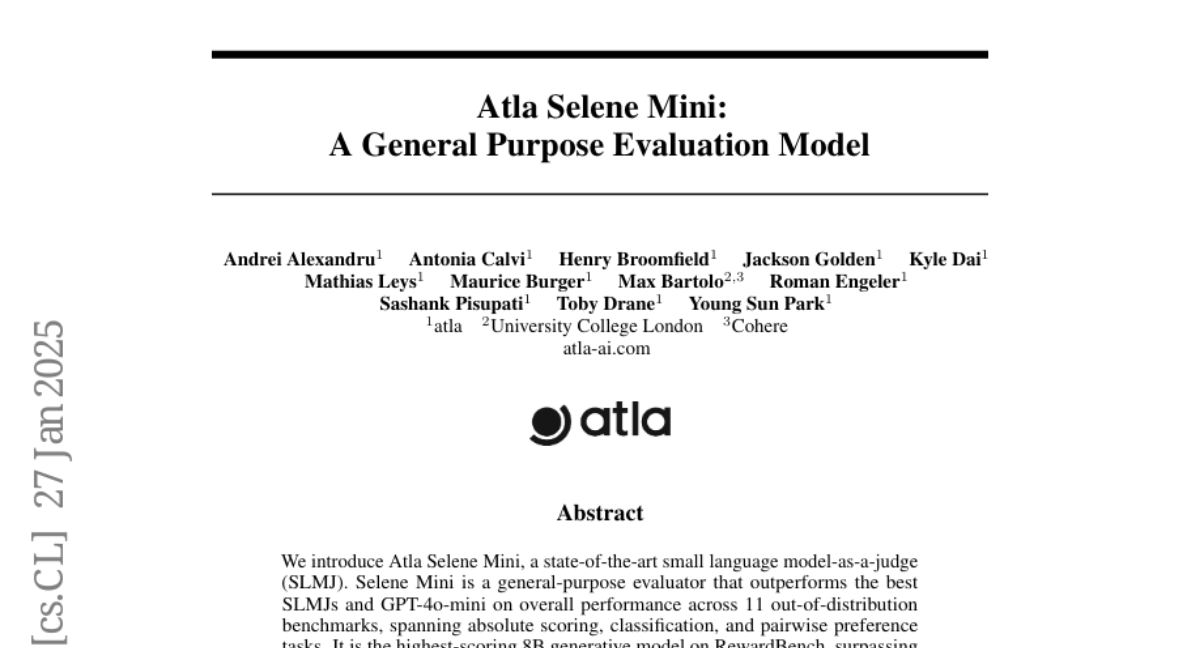
3. Exploring the sustainable scaling of AI dilemma: A projective study of corporations’ AI environmental impacts
π Keywords: Large Language Models, environmental impact, Generative AI, net-zero, AI electricity use
π‘ Category: AI Ethics and Fairness
π Research Objective:
– To develop a methodology for estimating the environmental impact of a company’s AI portfolio that provides actionable insights without needing extensive expertise in AI and Life-Cycle Assessment (LCA).
π οΈ Research Methods:
– Analysis includes modeling AI energy consumption, considering factors like AI usage increase, hardware efficiency, and electricity mix changes in accordance with IPCC scenarios.
π¬ Research Conclusions:
– Large generative AI models can consume up to 4600x more energy than traditional models, predicting a potential electricity use increase by a factor of 24.4 by 2030 under high adoption scenarios. Actions across the AI value chain are necessary to mitigate impacts effectively, promoting unified environmental assessment frameworks and transparency.
π Paper link: https://huggingface.co/papers/2501.14334
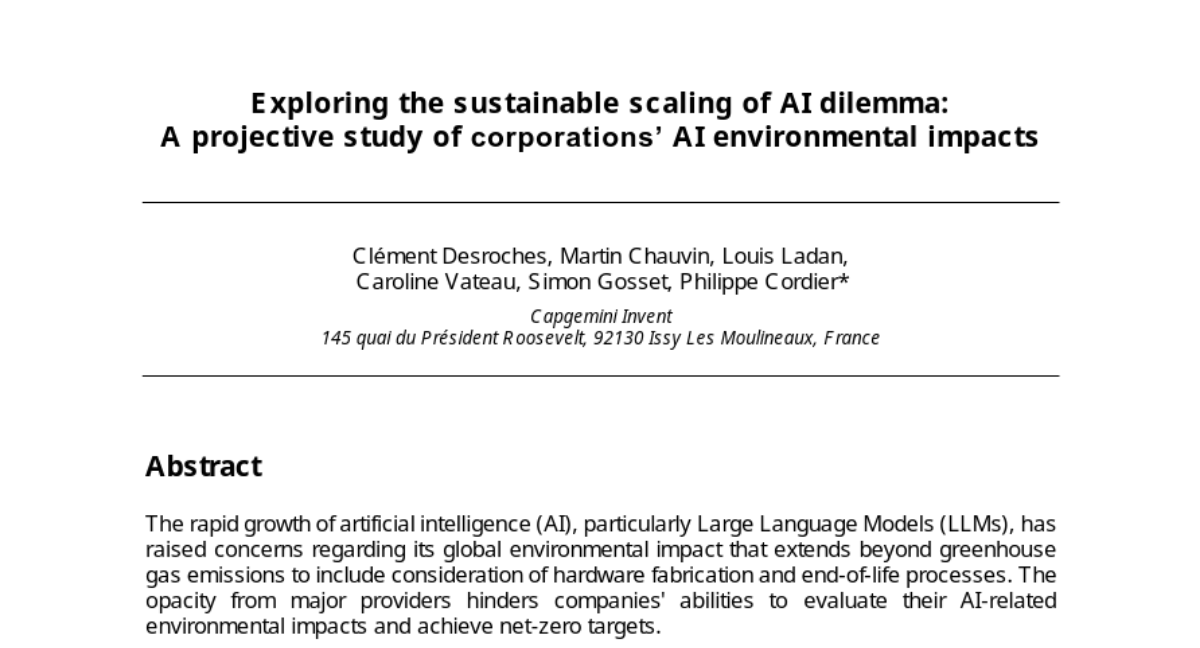
4. Early External Safety Testing of OpenAI’s o3-mini: Insights from the Pre-Deployment Evaluation
π Keywords: Large Language Models, Privacy, Safety Testing, ASTRAL
π‘ Category: AI Ethics and Fairness
π Research Objective:
– To ensure the responsible deployment of Large Language Models (LLMs) by assessing their safety through thorough testing.
π οΈ Research Methods:
– Employed ASTRAL tool to automatically generate and execute 10,080 unsafe test inputs on OpenAI’s o3-mini LLM during its early beta phase.
π¬ Research Conclusions:
– Identified 87 actual instances of unsafe LLM behavior, emphasizing the importance of pre-deployment safety testing.
π Paper link: https://huggingface.co/papers/2501.17749
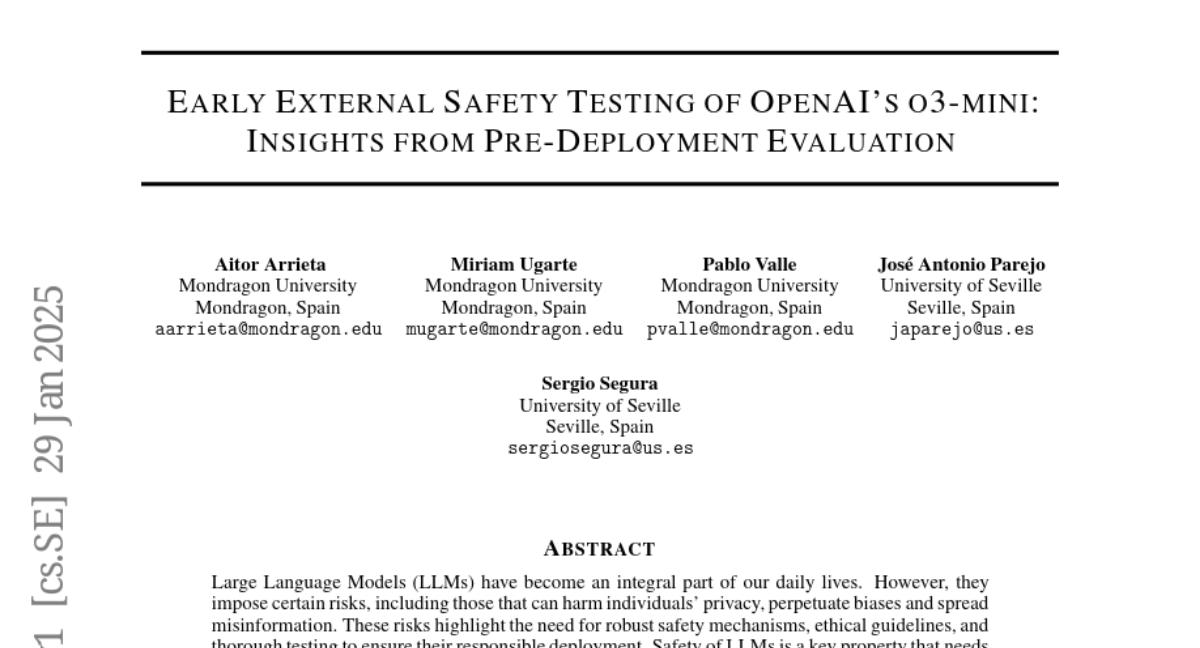
5. Any2AnyTryon: Leveraging Adaptive Position Embeddings for Versatile Virtual Clothing Tasks
π Keywords: Image-based Virtual Try-On, Data Scarcity, Generalization, Any2AnyTryon, LAION-Garment
π‘ Category: Generative Models
π Research Objective:
– The research aims to address the challenge of data scarcity in Image-based Virtual Try-On (VTON) and enhance the generalization and controllability of VTON generation.
π οΈ Research Methods:
– Introduced Any2AnyTryon, leveraging different textual instructions and model garment images to eliminate reliance on traditional conditions like masks and poses.
– Constructed the LAION-Garment dataset and used adaptive position embedding to improve VTON image generation across various input sizes and categories.
π¬ Research Conclusions:
– Any2AnyTryon demonstrated enhanced flexibility, controllability, and improved quality in virtual try-on generation compared to existing methods.
π Paper link: https://huggingface.co/papers/2501.15891
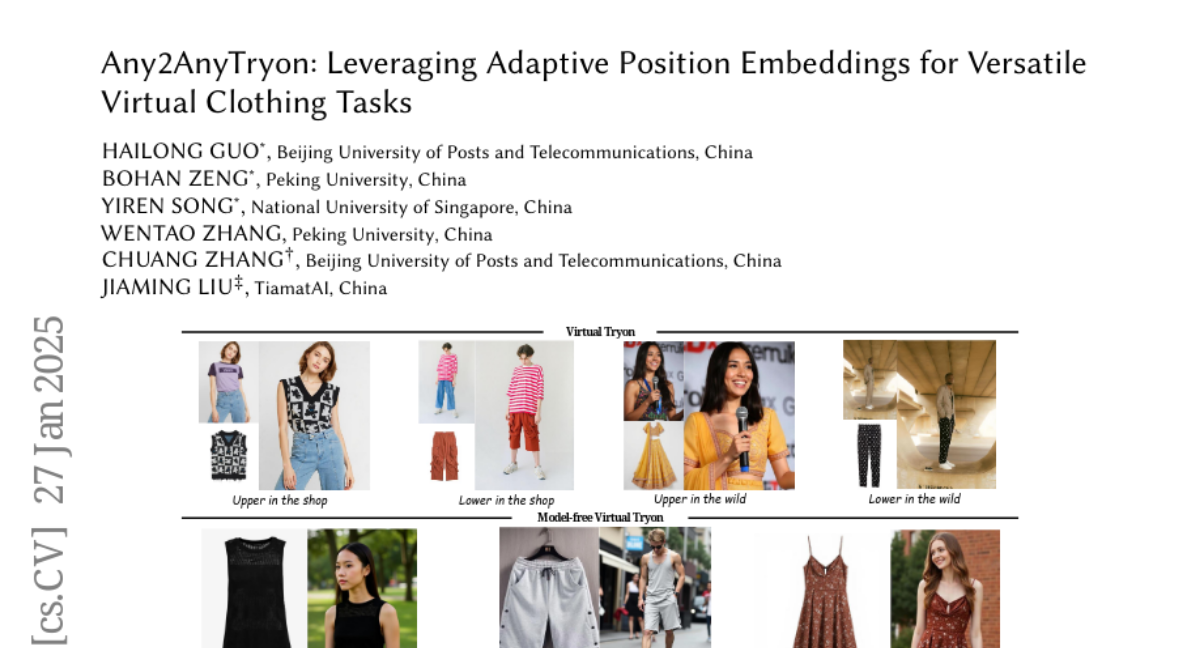
6. Virus: Harmful Fine-tuning Attack for Large Language Models Bypassing Guardrail Moderation
π Keywords: Large Language Models, fine-tuning attacks, guardrail, Virus, safety alignment
π‘ Category: Natural Language Processing
π Research Objective:
– This research aims to investigate the vulnerability of Large Language Models to fine-tuning attacks and examine the effectiveness of guardrail moderation in filtering harmful samples.
π οΈ Research Methods:
– The authors developed a novel attack method, named Virus, which modifies harmful data to bypass guardrail moderation. Experiments were conducted to test the method’s capability of evading detection.
π¬ Research Conclusions:
– The study concludes that relying solely on guardrail moderation is inadequate for preventing harmful fine-tuning attacks, as Virus demonstrated a high leakage ratio and enhanced attack performance, highlighting inherent safety issues in pre-trained LLMs.
π Paper link: https://huggingface.co/papers/2501.17433
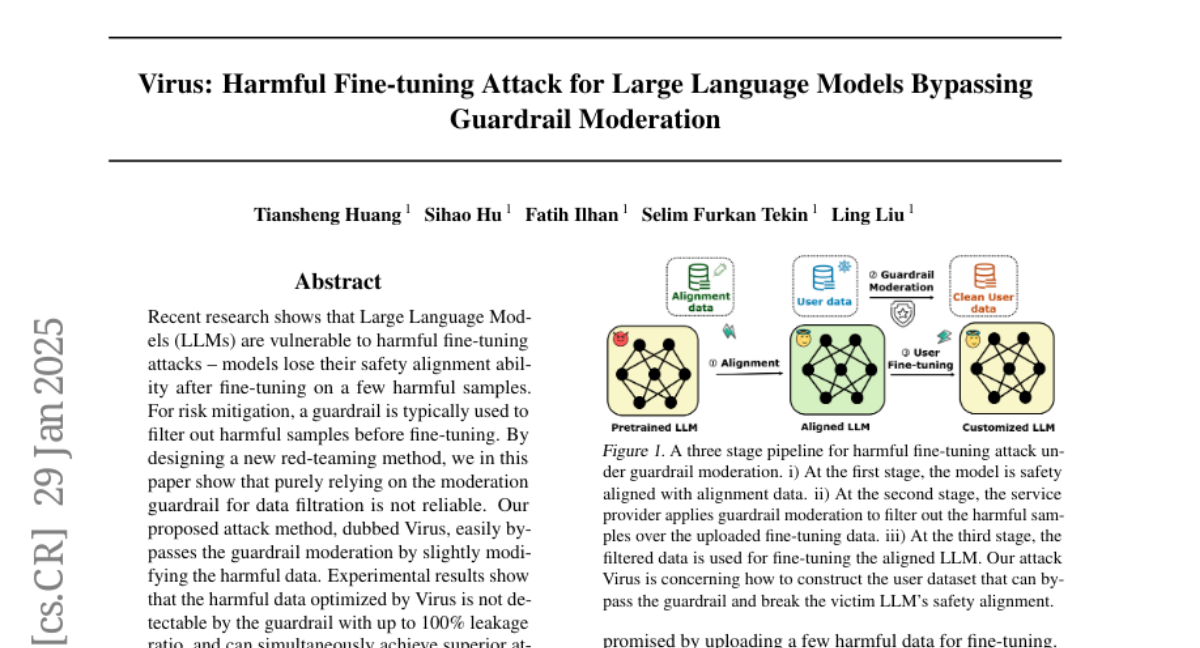
7. People who frequently use ChatGPT for writing tasks are accurate and robust detectors of AI-generated text
π Keywords: LLMs, AI-generated text, human detection, AI vocabulary, annotated dataset
π‘ Category: Natural Language Processing
π Research Objective:
– The study investigates human ability to detect AI-generated text from commercial LLMs like GPT-4o and Claude.
π οΈ Research Methods:
– Annotators were hired to review 300 non-fiction articles, categorize them, and explain their decisions.
π¬ Research Conclusions:
– Annotators familiar with LLMs excelled in detection, correctly classifying nearly all articles without specialized training. Complex text phenomena were identified beyond lexical clues, showcasing the limitations of automatic detectors.
π Paper link: https://huggingface.co/papers/2501.15654
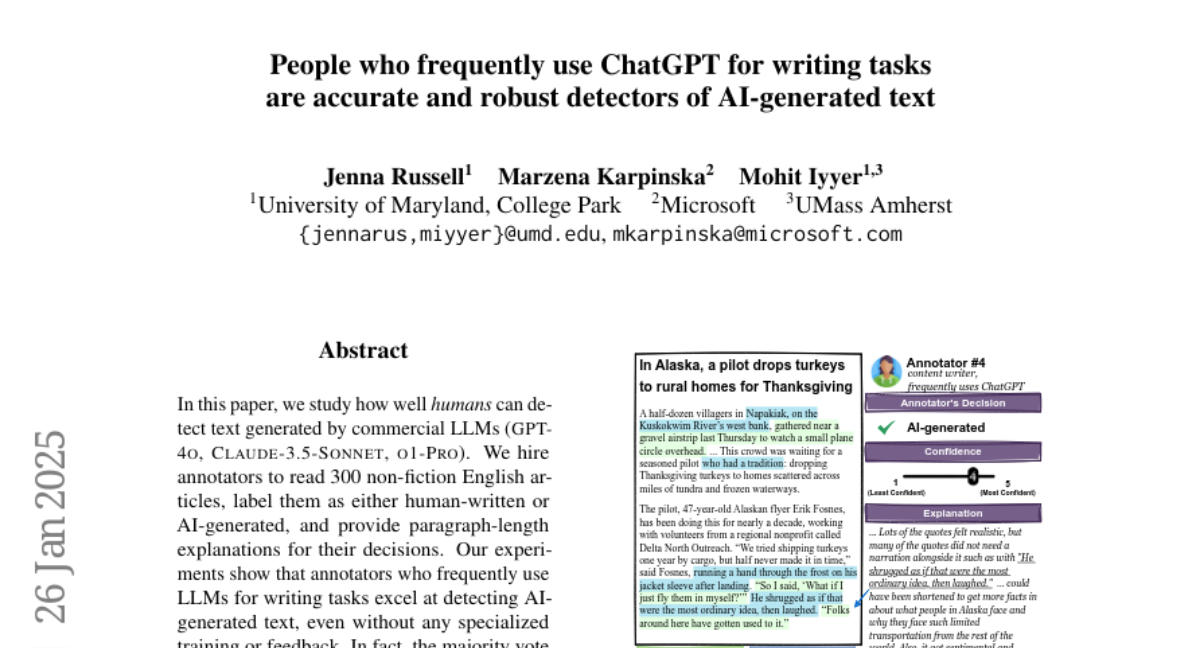