AI Native Daily Paper Digest – 20250204
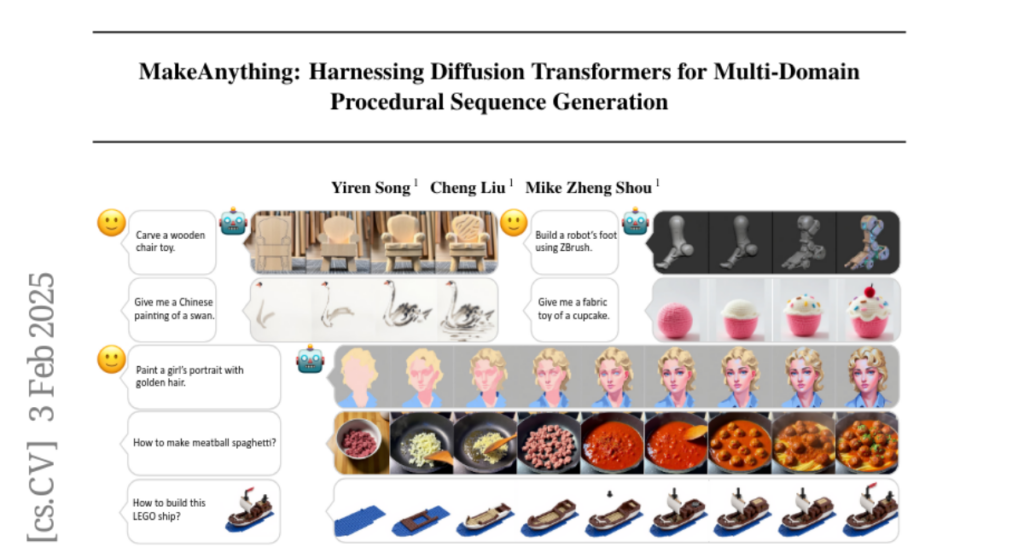
1. The Differences Between Direct Alignment Algorithms are a Blur
π Keywords: Direct Alignment Algorithms, Reinforcement Learning, Supervised Fine-Tuning, Pointwise Objectives
π‘ Category: Reinforcement Learning
π Research Objective:
– To simplify language model alignment by using Direct Alignment Algorithms (DAAs) instead of traditional Reinforcement Learning and Reward Modeling in the context of Reinforcement Learning from Human Feedback.
π οΈ Research Methods:
– DAAs are classified based on ranking losses, rewards used, and whether Supervised Fine-Tuning (SFT) is required. The study incorporated an explicit SFT phase and introduced a beta parameter for preference optimization in one-stage methods.
π¬ Research Conclusions:
– One-stage methods initially underperform compared to two-stage methods; however, with SFT and beta parameter modifications, performance matches two-stage methods, underscoring the importance of careful evaluation of alignment algorithms.
π Paper link: https://huggingface.co/papers/2502.01237
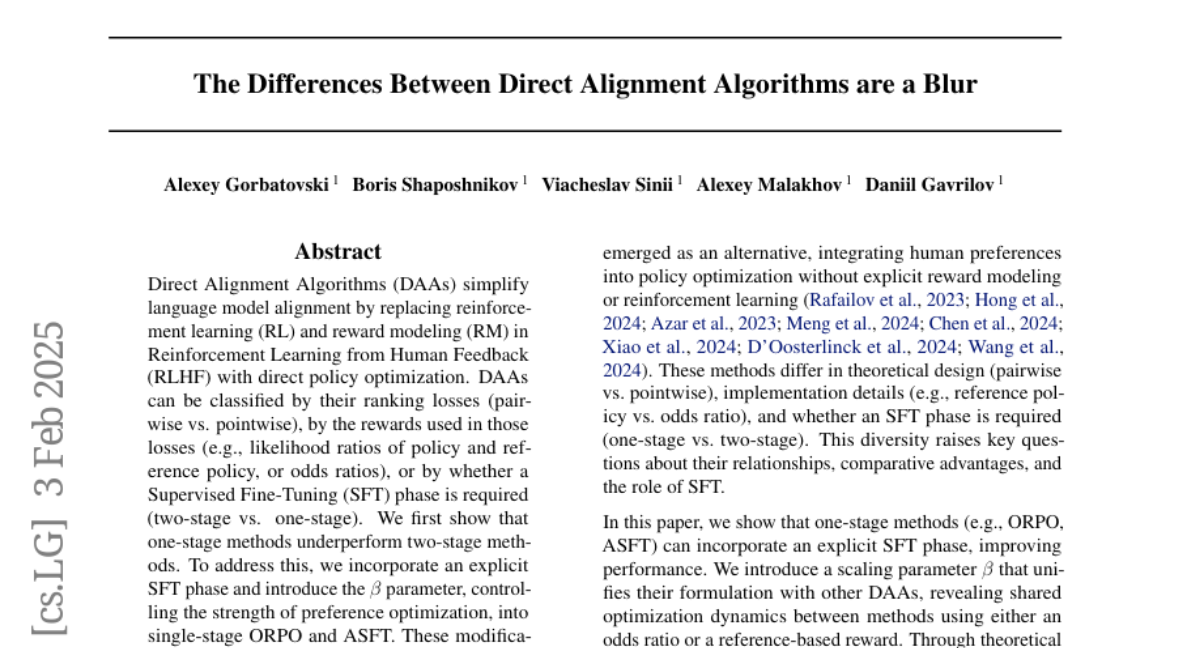
2. OmniHuman-1: Rethinking the Scaling-Up of One-Stage Conditioned Human Animation Models
π Keywords: OmniHuman, Diffusion Transformer, motion generation, realistic video, audio-driven
π‘ Category: Generative Models
π Research Objective:
– Develop OmniHuman, a framework to enhance data scaling in human animation by incorporating motion-related conditions.
π οΈ Research Methods:
– Introduce training principles for mixed motion conditions, along with model architecture and inference strategy improvements.
π¬ Research Conclusions:
– OmniHuman generates more realistic and flexible human videos compared to existing methods and supports multiple driving modalities and portrait contents.
π Paper link: https://huggingface.co/papers/2502.01061
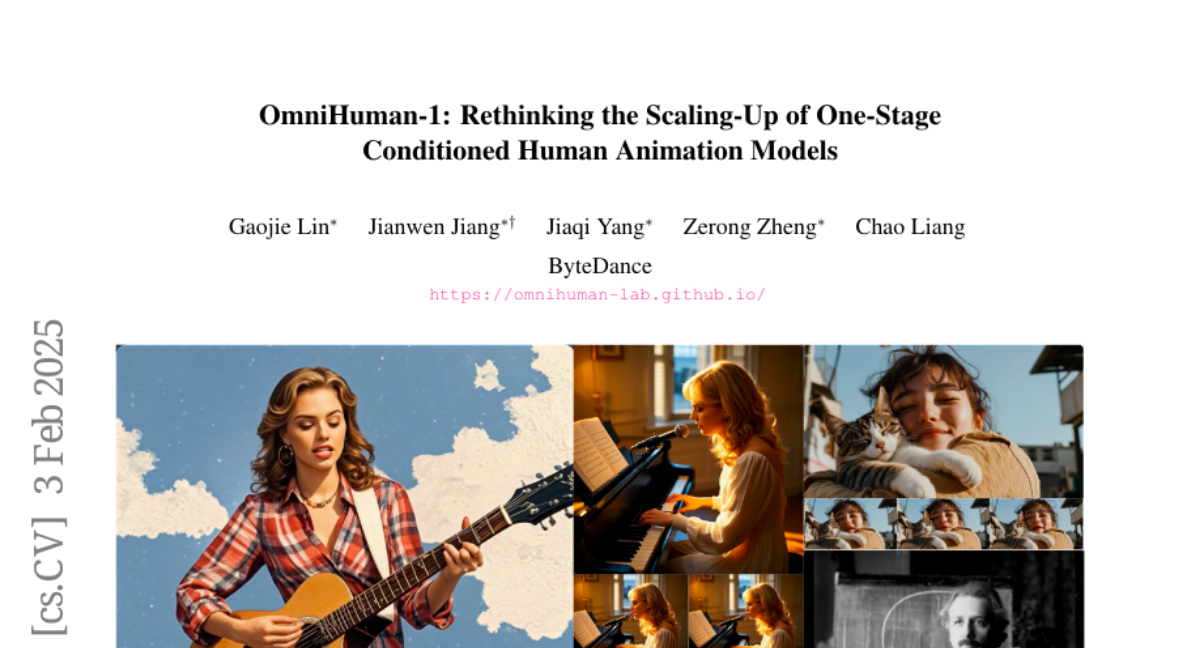
3. Process Reinforcement through Implicit Rewards
π Keywords: Dense Process Rewards, Reinforcement Learning, PRMs, PRIME
π‘ Category: Reinforcement Learning
π Research Objective:
– Investigate the effectiveness of dense process rewards over sparse outcome-level rewards in large language models, particularly for complex multi-step reasoning tasks.
π οΈ Research Methods:
– Develop PRIME (Process Reinforcement through IMplicit rEwards), which supports online updates to process reward models (PRMs) using only policy rollouts and outcome labels.
π¬ Research Conclusions:
– PRIME demonstrates significant improvements over standard SFT models, with a 15.1% average improvement on reasoning benchmarks and surpassing a comparable model with significantly less training data.
π Paper link: https://huggingface.co/papers/2502.01456
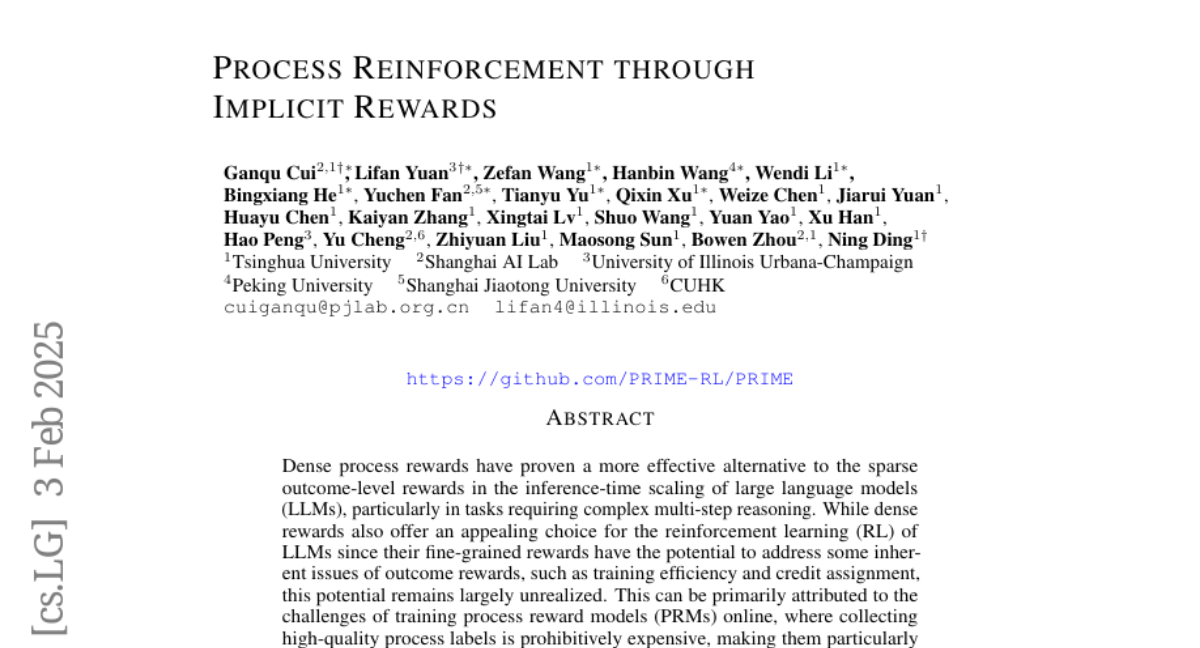
4. Preference Leakage: A Contamination Problem in LLM-as-a-judge
π Keywords: Large Language Models, data annotation, model development, preference leakage
π‘ Category: Natural Language Processing
π Research Objective:
– To investigate preference leakage in LLM-as-a-judge caused by relatedness between synthetic data generators and evaluators.
π οΈ Research Methods:
– Defined three common relatedness scenarios and conducted extensive experiments across multiple LLM models and benchmarks.
π¬ Research Conclusions:
– Identified preference leakage as a pervasive and harder-to-detect issue compared to previously known biases in LLM-as-a-judge paradigms.
π Paper link: https://huggingface.co/papers/2502.01534
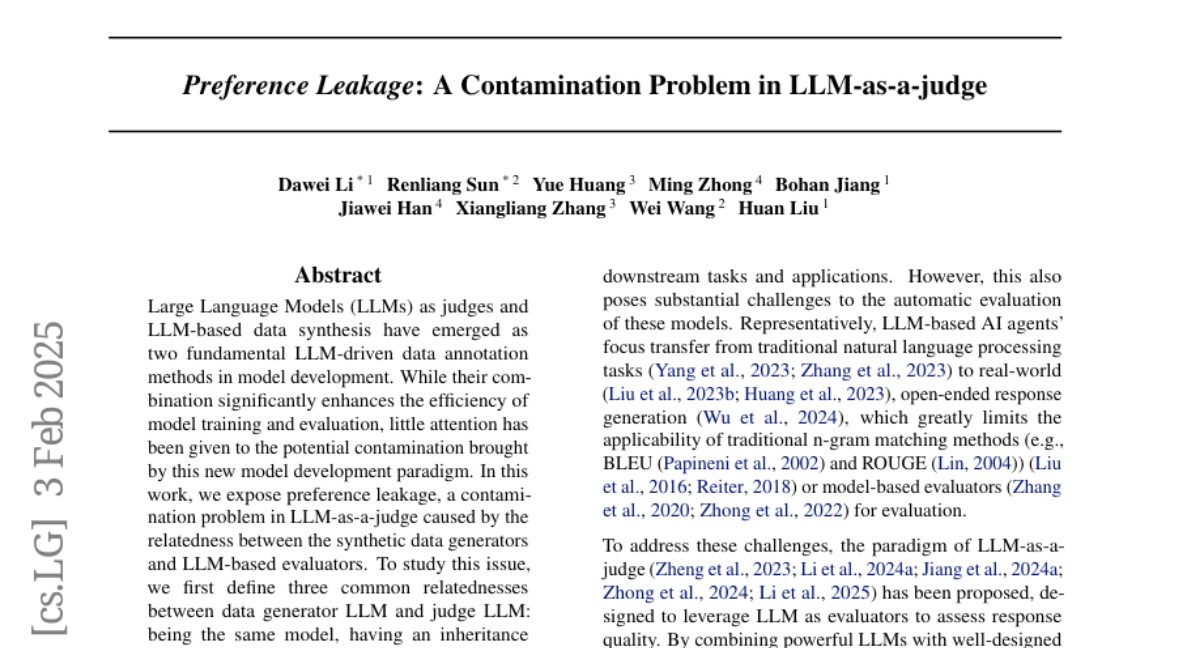
5. SafeRAG: Benchmarking Security in Retrieval-Augmented Generation of Large Language Model
π Keywords: Retrieval-Augmented Generation, Large Language Models, Vulnerability, SafeRAG
π‘ Category: Natural Language Processing
π Research Objective:
– Introduce SafeRAG, a benchmark designed to evaluate the security of the Retrieval-Augmented Generation (RAG) paradigm.
π οΈ Research Methods:
– Classify attack tasks into silver noise, inter-context conflict, soft ad, and white Denial-of-Service.
– Construct a security evaluation dataset named SafeRAG manually for each identified task.
– Simulate various attack scenarios using the SafeRAG dataset.
π¬ Research Conclusions:
– RAG components exhibit significant vulnerability to various attack tasks, impacting the service quality by bypassing existing security measures.
π Paper link: https://huggingface.co/papers/2501.18636
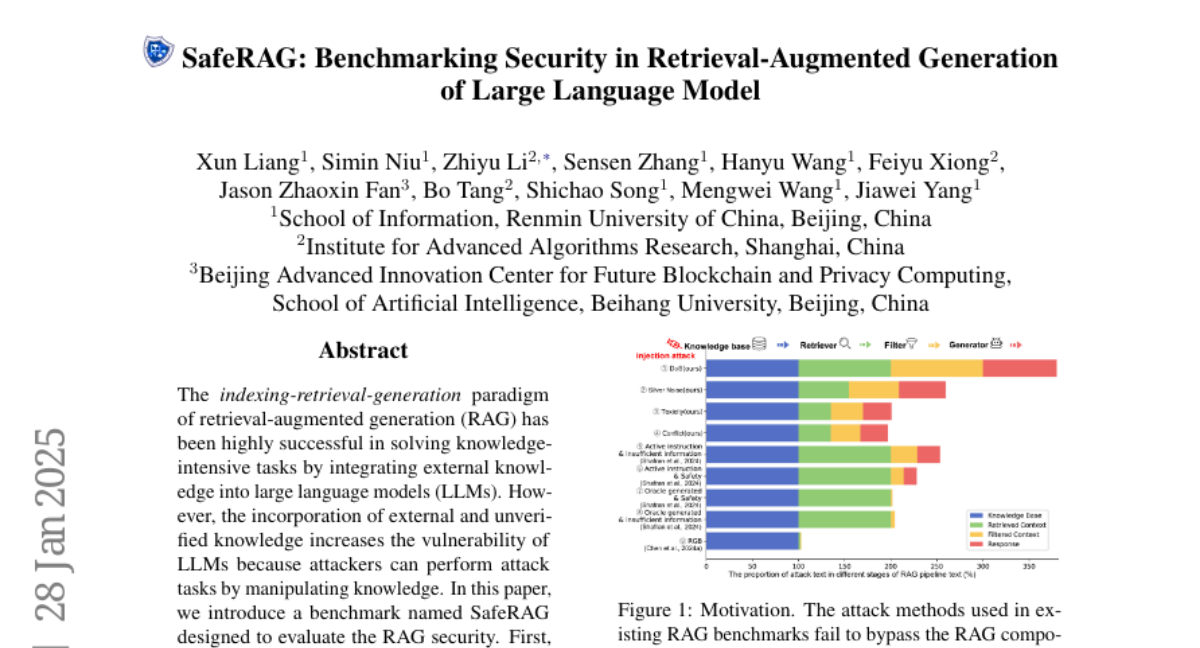
6. AlignVLM: Bridging Vision and Language Latent Spaces for Multimodal Understanding
π Keywords: Vision-Language Models, Semantic Similarity, Multimodal Alignment
π‘ Category: Multi-Modal Learning
π Research Objective:
– Introduce AlignVLM to improve alignment between visual features and language embeddings in vision-language models.
π οΈ Research Methods:
– Propose a method that maps visual features to a weighted average of LLM text embeddings, leveraging linguistic priors.
π¬ Research Conclusions:
– AlignVLM demonstrates state-of-the-art performance and enhanced robustness for document understanding tasks.
π Paper link: https://huggingface.co/papers/2502.01341
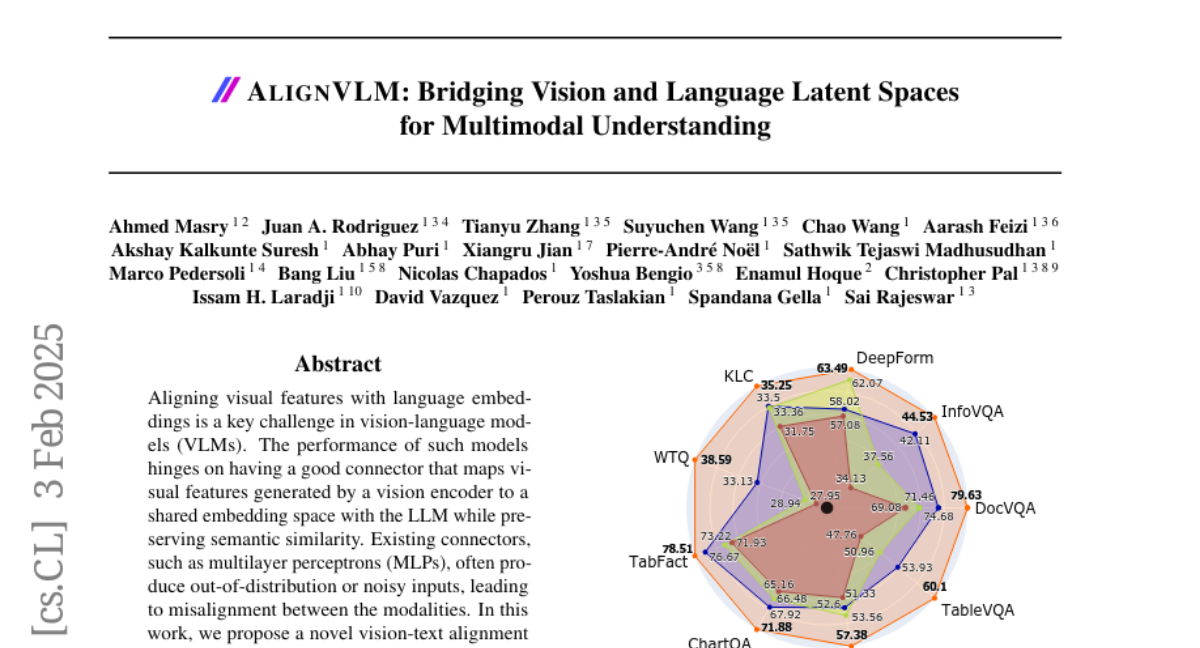
7. SliderSpace: Decomposing the Visual Capabilities of Diffusion Models
π Keywords: SliderSpace, diffusion models, compositional control, concept decomposition, artistic style
π‘ Category: Generative Models
π Research Objective:
– The objective is to develop SliderSpace, a framework that automatically decomposes visual capabilities of diffusion models into controllable and understandable directions, enabling more intuitive and diverse manipulations.
π οΈ Research Methods:
– SliderSpace discovers multiple interpretable directions from a single text prompt and trains each as a low-rank adaptor, allowing for compositional control and new possibilities in the model’s latent space through extensive experiments on diffusion models.
π¬ Research Conclusions:
– SliderSpace effectively decomposes the visual structure of model knowledge, offering insights into latent capabilities and producing more diverse and useful variations compared to traditional methods, as validated through quantitative evaluation and user studies.
π Paper link: https://huggingface.co/papers/2502.01639
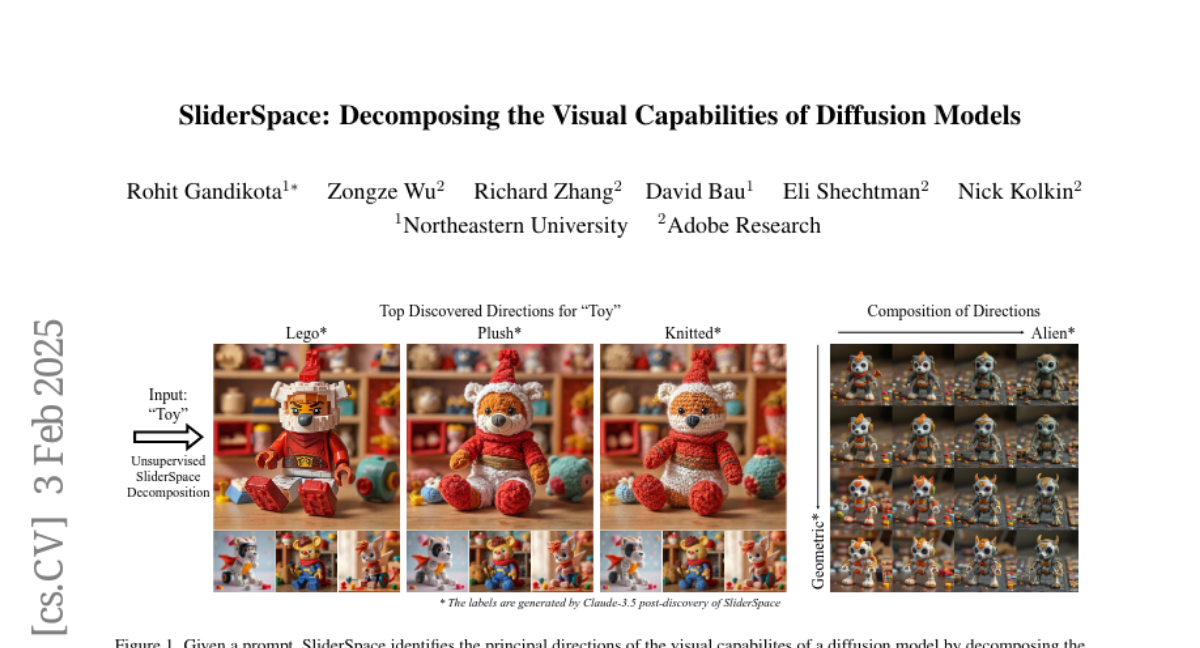
8. MM-IQ: Benchmarking Human-Like Abstraction and Reasoning in Multimodal Models
π Keywords: IQ Testing, Cognitive Capabilities, Multi-Modal Systems, Benchmark, Reasoning
π‘ Category: Multi-Modal Learning
π Research Objective:
– Propose MM-IQ, a comprehensive framework to evaluate core cognitive competencies in multimodal AI systems.
π οΈ Research Methods:
– Developed 2,710 test items across 8 reasoning paradigms for a systematic evaluation of multimodal models.
π¬ Research Conclusions:
– Identified significant limitations in current architectures as they perform only slightly better than random, emphasizing the need for major advancements to improve AI reasoning capacities.
π Paper link: https://huggingface.co/papers/2502.00698
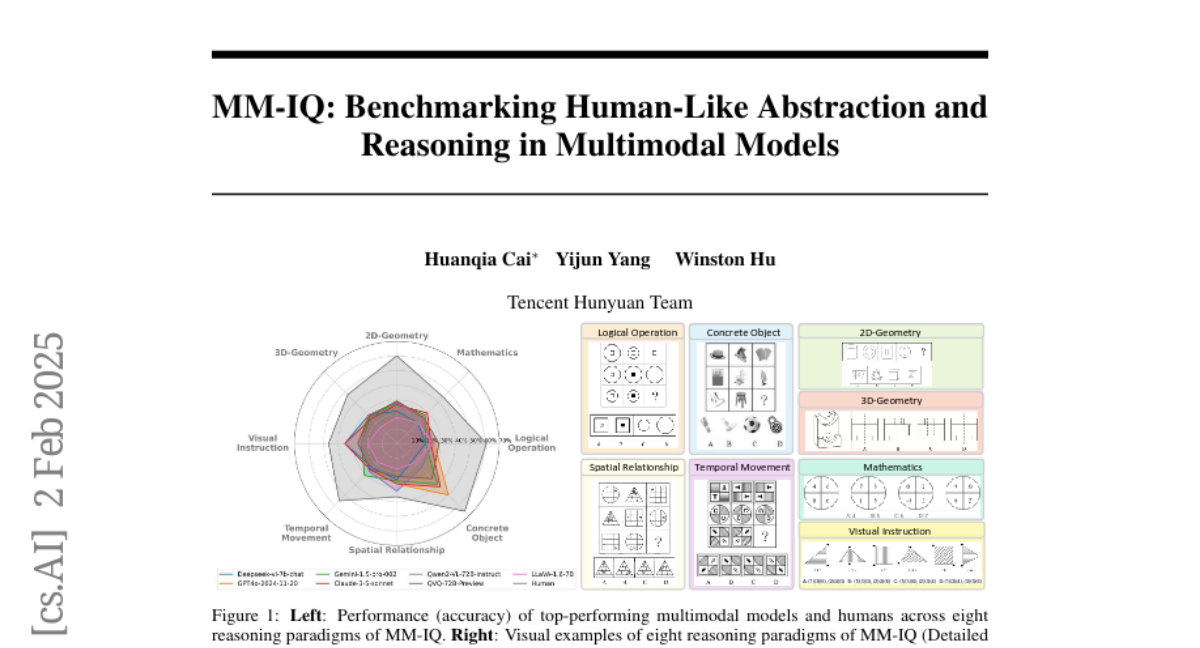
9. AIN: The Arabic INclusive Large Multimodal Model
π Keywords: Large Multimodal Models, AIN, Arabic Language, Generative AI, AI Native
π‘ Category: Multi-Modal Learning
π Research Objective:
– The study aims to address the gap in Arabic LLMs by introducing AIN, an Arabic Inclusive Multimodal Model, which performs strongly across diverse domains in both Arabic and English.
π οΈ Research Methods:
– AIN was developed using 3.6 million high-quality Arabic-English multimodal data samples to achieve state-of-the-art performance in multilingual settings.
π¬ Research Conclusions:
– AIN demonstrates superior performance on the CAMEL-Bench benchmark, outperforming established models like GPT-4o by an absolute gain of 3.4% over eight domains and 38 sub-domains, positioning it as a crucial tool for empowering Arabic speakers with advanced AI technologies.
π Paper link: https://huggingface.co/papers/2502.00094
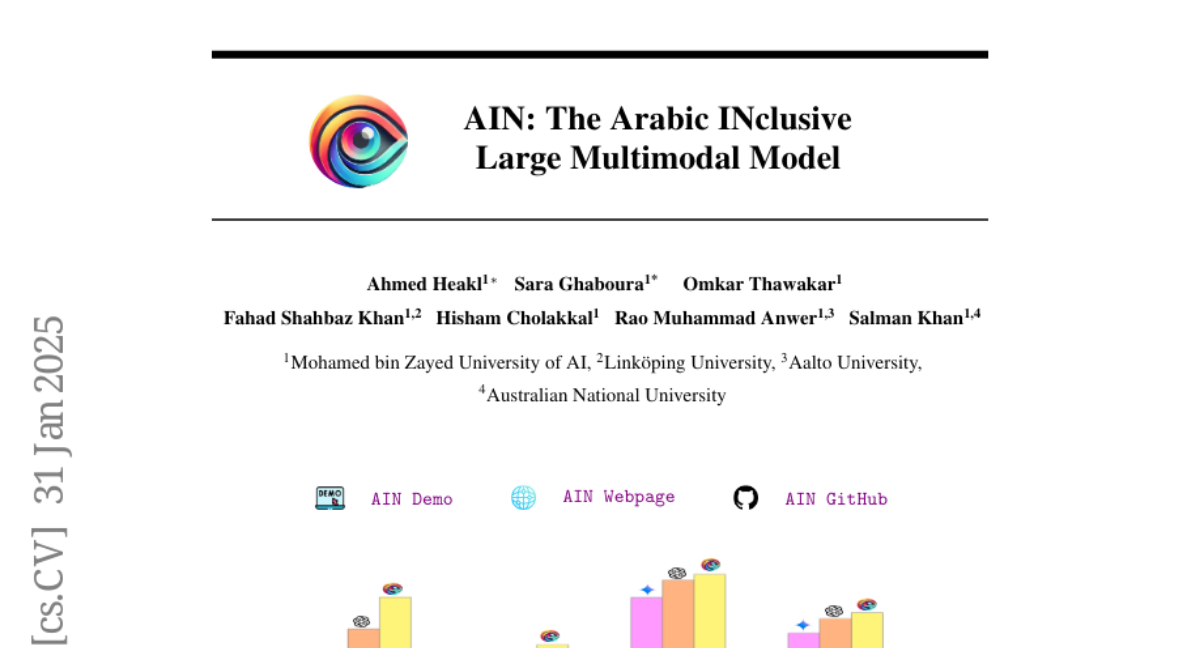
10. FastKV: KV Cache Compression for Fast Long-Context Processing with Token-Selective Propagation
π Keywords: FastKV, KV cache compression, latency, long-context sequences, Generative Models
π‘ Category: Generative Models
π Research Objective:
– The objective of the research is to introduce FastKV, a key-value (KV) cache compression method that enhances latency for long-context sequences in large language models.
π οΈ Research Methods:
– FastKV employs a Token-Selective Propagation (TSP) approach to maintain full context information in initial layers and selectively propagate this information in deeper layers. It also uses grouped-query attention (GQA)-aware KV cache compression for improved memory and computational efficiency.
π¬ Research Conclusions:
– FastKV demonstrates significant improvement, achieving 2.00 times enhancement in time-to-first-token (TTFT) and 1.40 times improvement in throughput compared to the previous state-of-the-art method, HeadKV, while maintaining accuracy on long-context benchmarks.
π Paper link: https://huggingface.co/papers/2502.01068
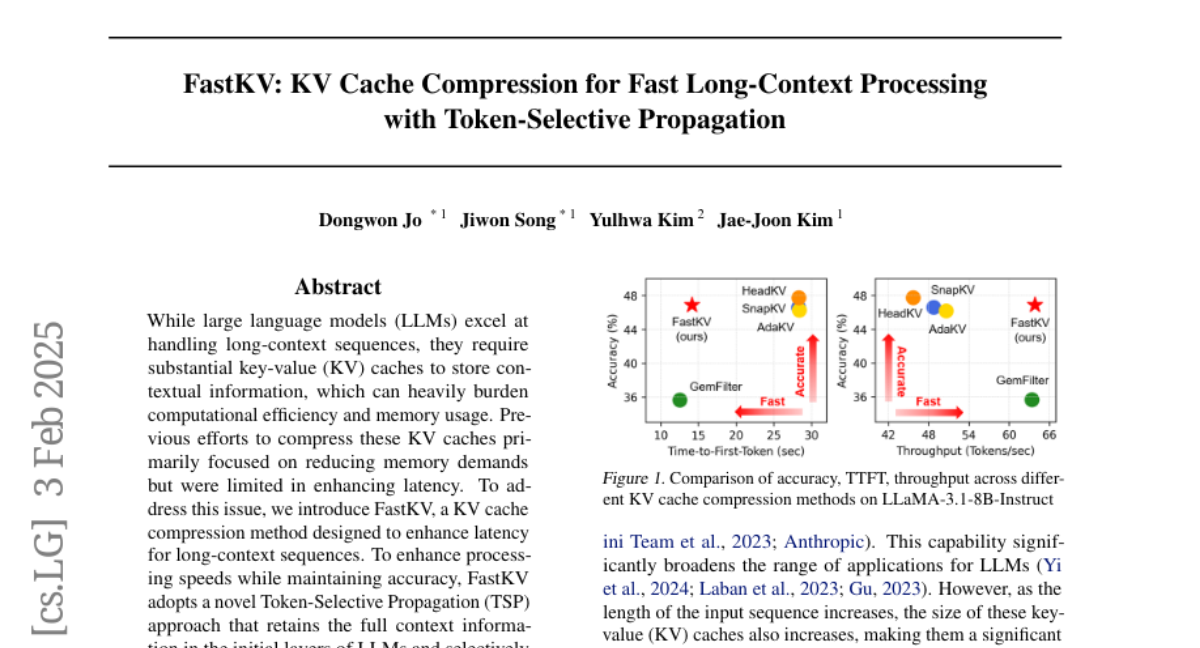
11. Scaling Embedding Layers in Language Models
π Keywords: SCONE, N-gram Embedding, Language Model, Inference Speed, Contextualized Representation
π‘ Category: Natural Language Processing
π Research Objective:
– The paper aims to enhance the performance of language models by extending input embedding layers without increasing decoding costs.
π οΈ Research Methods:
– SCONE introduces embeddings for frequent n-grams, using a separate model for learning during training. These embeddings are precomputed for inference and stored in off-accelerator memory to minimize speed impact.
π¬ Research Conclusions:
– SCONE successfully allows for new scaling strategies in language models, significantly outperforming a 1.9B parameter baseline while maintaining fixed inference-time FLOPS.
π Paper link: https://huggingface.co/papers/2502.01637
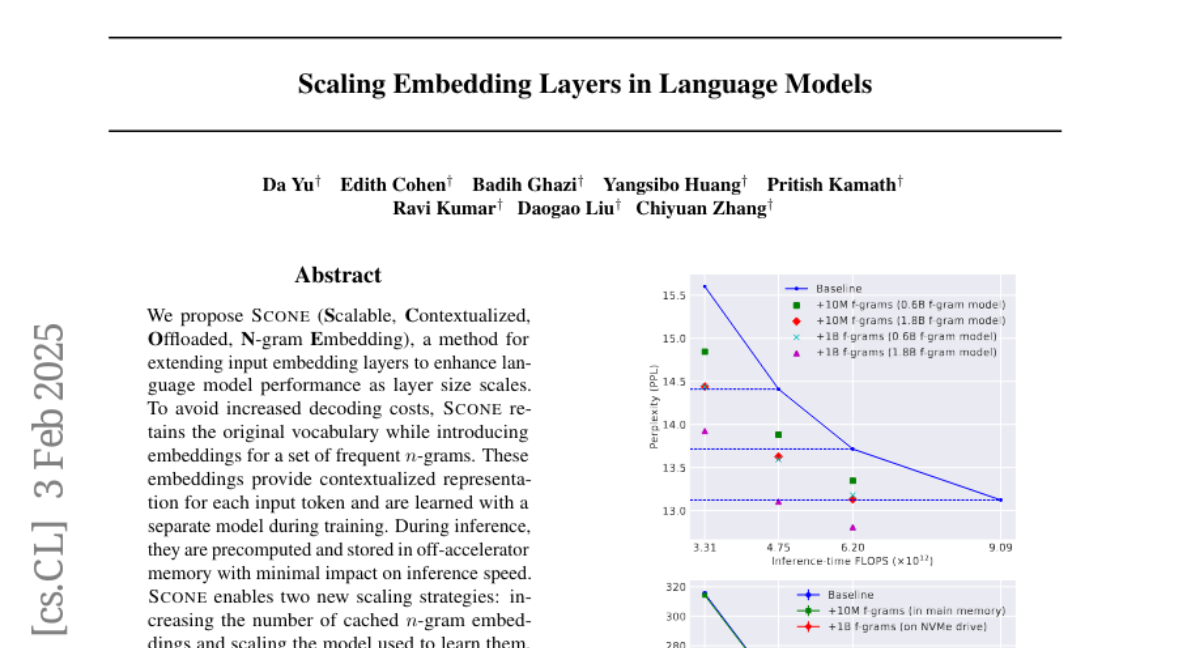
12. ZebraLogic: On the Scaling Limits of LLMs for Logical Reasoning
π Keywords: logical reasoning, large language models, curse of complexity, non-monotonic reasoning, scalability
π‘ Category: Knowledge Representation and Reasoning
π Research Objective:
– The study aims to investigate the logical reasoning capabilities and scalability of large language models (LLMs) through the use of ZebraLogic, a comprehensive evaluation framework.
π οΈ Research Methods:
– Introduced ZebraLogic, which assesses LLM performance on logic grid puzzles derived from constraint satisfaction problems, allowing for controlled and quantifiable complexity analysis.
π¬ Research Conclusions:
– The research identifies a significant decline in accuracy of LLMs as problem complexity increases, highlighting a fundamental limitation referred to as the curse of complexity. Despite using larger models and increased inference-time computation, this limitation persists. The study explores potential strategies for improvement, such as Best-of-N sampling and self-verification prompts.
π Paper link: https://huggingface.co/papers/2502.01100
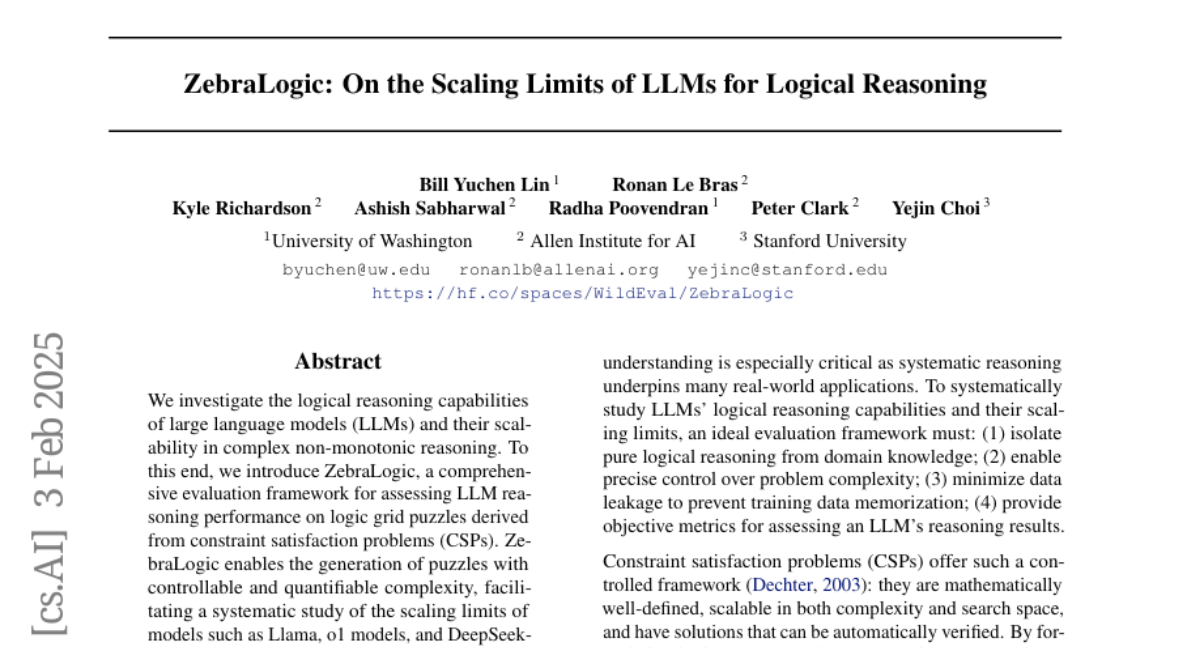
13. DeepRAG: Thinking to Retrieval Step by Step for Large Language Models
π Keywords: Large Language Models, Retrieval-Augmented Generation, Markov Decision Process, Retrieval Efficiency
π‘ Category: Natural Language Processing
π Research Objective:
– To propose DeepRAG, a framework that models retrieval-augmented reasoning as a Markov Decision Process for strategic and adaptive retrieval.
π οΈ Research Methods:
– Implementation of DeepRAG that iteratively decomposes queries to dynamically choose between retrieving external knowledge or relying on parametric reasoning.
π¬ Research Conclusions:
– DeepRAG improves retrieval efficiency and answer accuracy by 21.99%, optimizing retrieval-augmented reasoning.
π Paper link: https://huggingface.co/papers/2502.01142
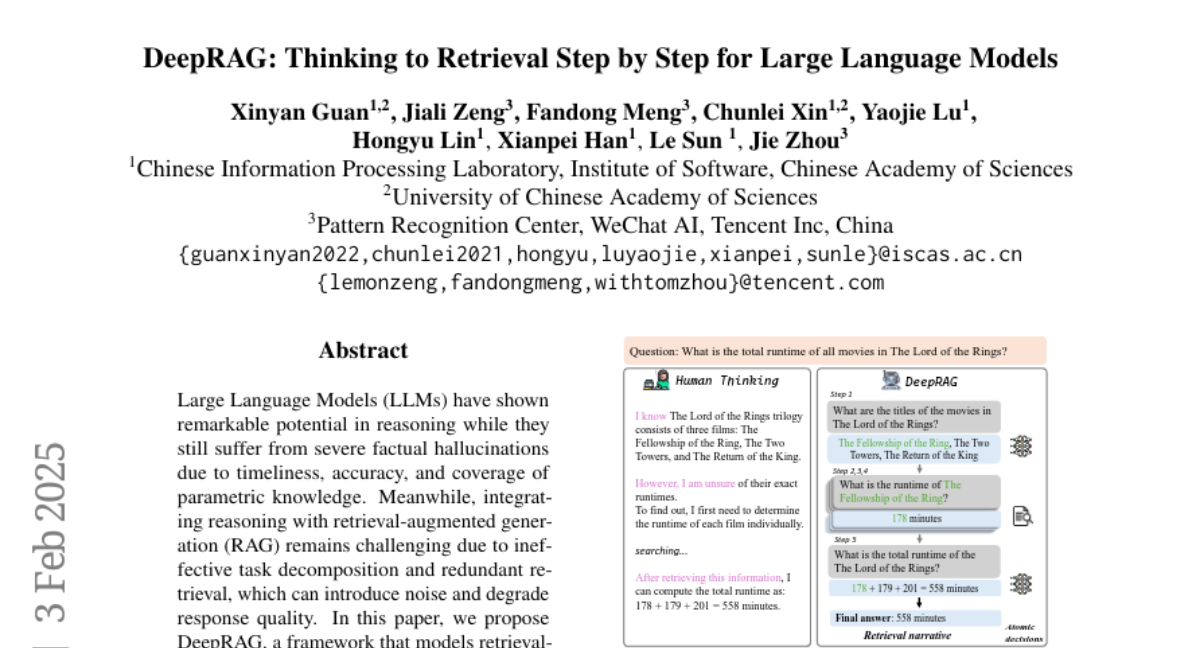
14. Improving Transformer World Models for Data-Efficient RL
π Keywords: Model-Based RL, Craftax-classic benchmark, deep exploration, generalization, long-term reasoning
π‘ Category: Reinforcement Learning
π Research Objective:
– To achieve a new state of the art performance in model-based reinforcement learning on the Craftax-classic benchmark, surpassing both existing models and human performance.
π οΈ Research Methods:
– Developed a novel policy architecture combining CNNs and RNNs to establish a model-free baseline and introduced improvements including “Dyna with warmup”, “nearest neighbor tokenizer”, and “block teacher forcing” to enhance MBRL efficiency.
π¬ Research Conclusions:
– The proposed model-based RL approach exceeded human performance in the Craftax-classic benchmark with a 67.4% reward after 1M environment steps, outperforming DreamerV3’s 53.2%.
π Paper link: https://huggingface.co/papers/2502.01591
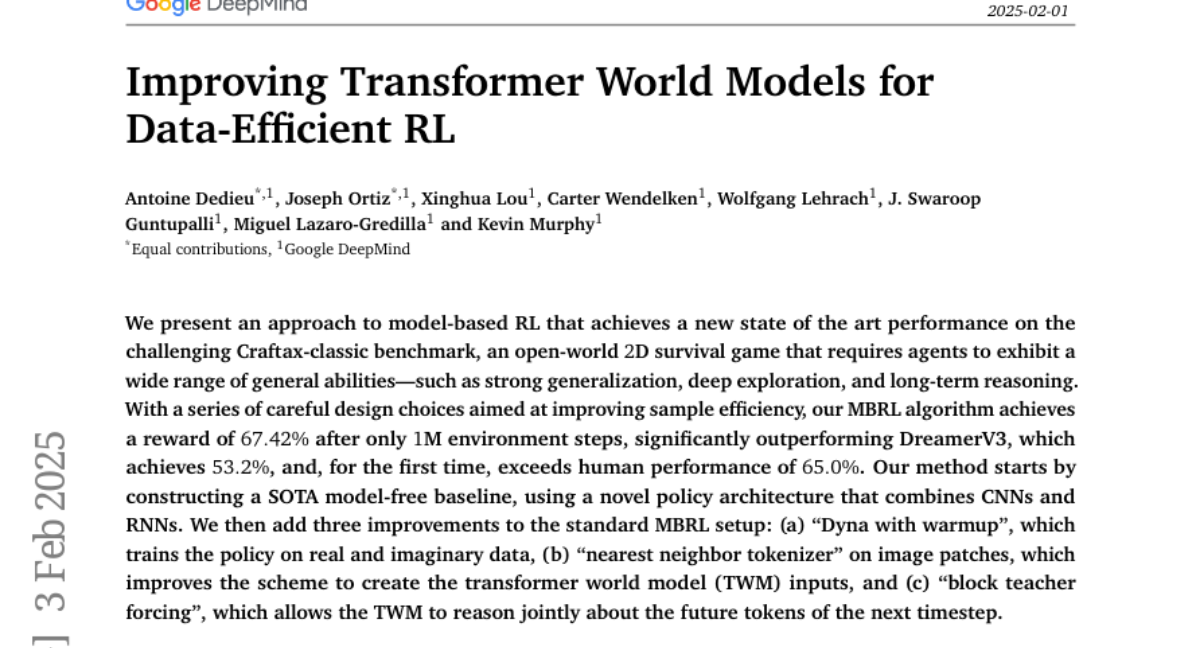
15. The Jumping Reasoning Curve? Tracking the Evolution of Reasoning Performance in GPT-[n] and o-[n] Models on Multimodal Puzzles
π Keywords: OpenAI, Large Language Models, Multimodal Tasks, Reasoning Capabilities, Computational Cost
π‘ Category: Multi-Modal Learning
π Research Objective:
– The study aims to evaluate the evolution of reasoning capabilities in large language models, specifically focusing on their performance in challenging multimodal tasks.
π οΈ Research Methods:
– The research involves tracking the development of GPT-[n] and o-[n] series models, emphasizing their ability to solve complex puzzles that require a combination of visual perception and abstract reasoning.
π¬ Research Conclusions:
– Results indicate an improvement in reasoning capabilities across model iterations. However, even with significant advancements, the models still face challenges in solving simple multimodal puzzles that require abstract reasoning, raising efficiency concerns due to computational costs.
π Paper link: https://huggingface.co/papers/2502.01081
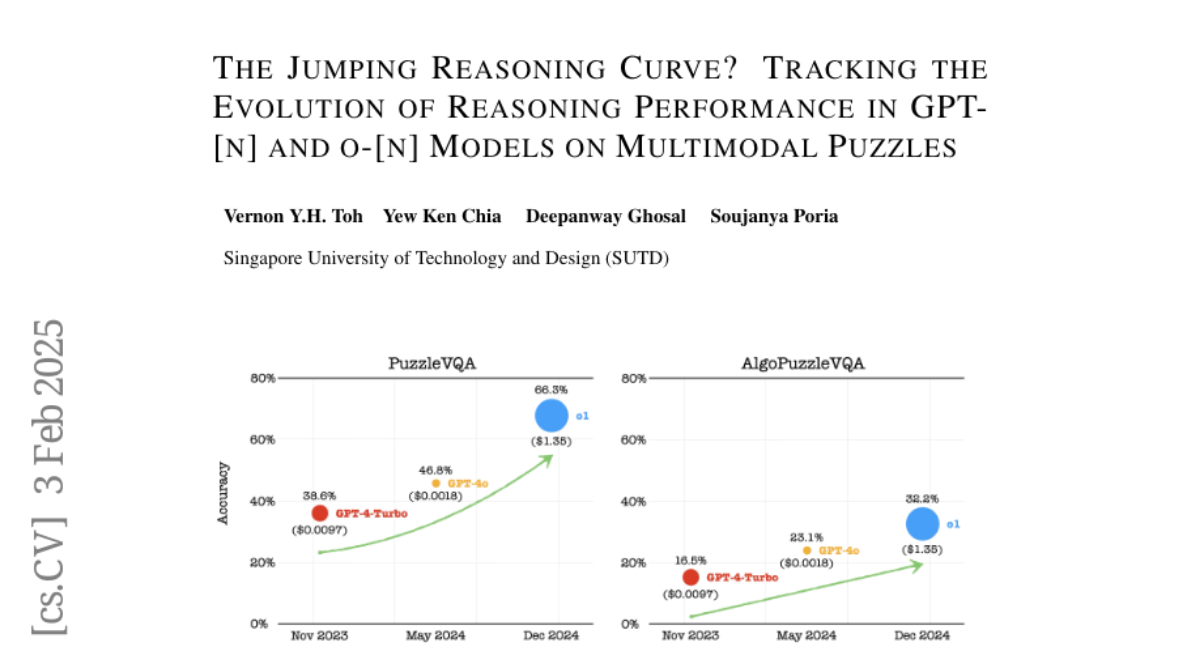
16. Improved Training Technique for Latent Consistency Models
π Keywords: Consistency models, Latent space, Outliers, Diffusion loss, Optimal transport
π‘ Category: Generative Models
π Research Objective:
– To enhance the performance of consistency models in the latent space for large-scale text-to-image and video generation tasks.
π οΈ Research Methods:
– Replacing Pseudo-Huber losses with Cauchy losses to mitigate outlier impact.
– Introducing diffusion loss at early timesteps and using optimal transport coupling.
– Implementing an adaptive scaling-c scheduler and Non-scaling LayerNorm in the architecture.
π¬ Research Conclusions:
– Successfully trained latent consistency models capable of high-quality sampling with one or two steps, significantly closing the performance gap with diffusion models.
π Paper link: https://huggingface.co/papers/2502.01441
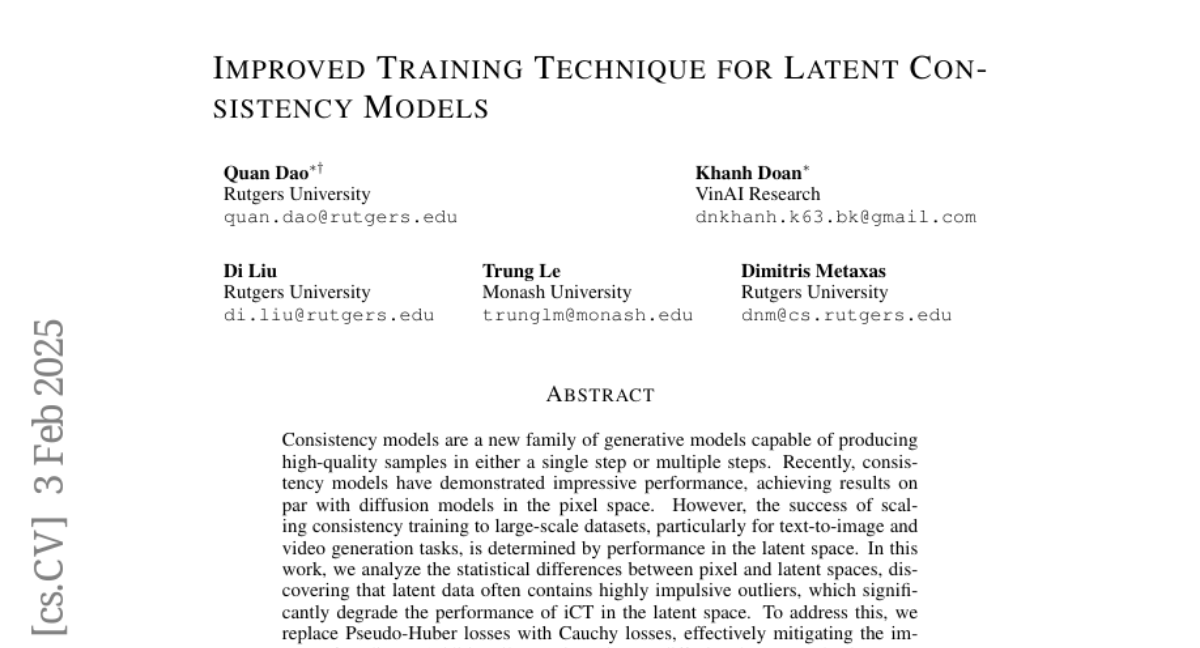
17. Almost Surely Safe Alignment of Large Language Models at Inference-Time
π Keywords: Language Models, Alignment, Safety, Inference-Time, Constrained Markov Decision Process
π‘ Category: Natural Language Processing
π Research Objective:
– Introduce a novel inference-time alignment approach to ensure language models generate safe responses with high probability.
π οΈ Research Methods:
– Frame safe response generation as a constrained Markov decision process within the model’s latent space.
– Propose InferenceGuard to implement safety alignment without altering model weights.
π¬ Research Conclusions:
– InferenceGuard effectively balances safety and task performance, outperforming existing methods in generating safe and aligned responses.
π Paper link: https://huggingface.co/papers/2502.01208
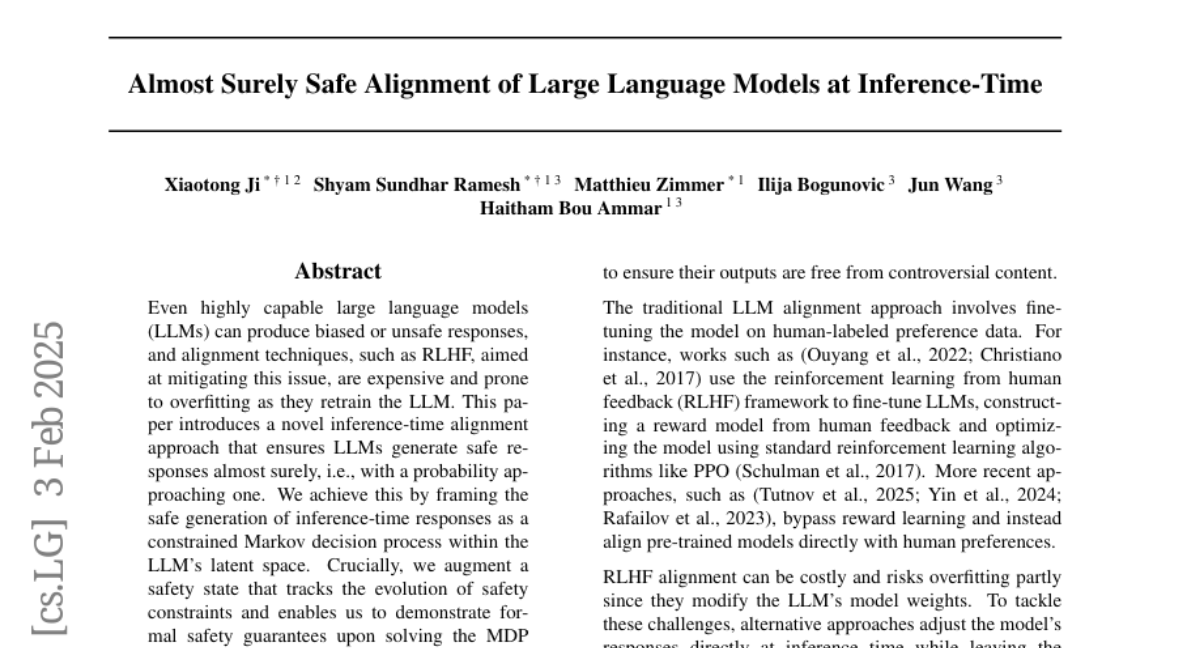
18. PhD Knowledge Not Required: A Reasoning Challenge for Large Language Models
π Keywords: General Knowledge, Reasoning Models, Capability Gaps, Inference-Time Technique
π‘ Category: Knowledge Representation and Reasoning
π Research Objective:
– The paper presents a new benchmark based on the NPR Sunday Puzzle Challenge to test general knowledge.
π οΈ Research Methods:
– The benchmark evaluates reasoning models’ performance, offering insights into capability gaps not evident in benchmarks for specialized knowledge.
π¬ Research Conclusions:
– OpenAI o1 outperforms other models in reasoning tasks, while DeepSeek R1 exhibits various failure modes like giving up or displaying uncertainty, indicating the need for new inference-time techniques to improve accuracy and completion.
π Paper link: https://huggingface.co/papers/2502.01584
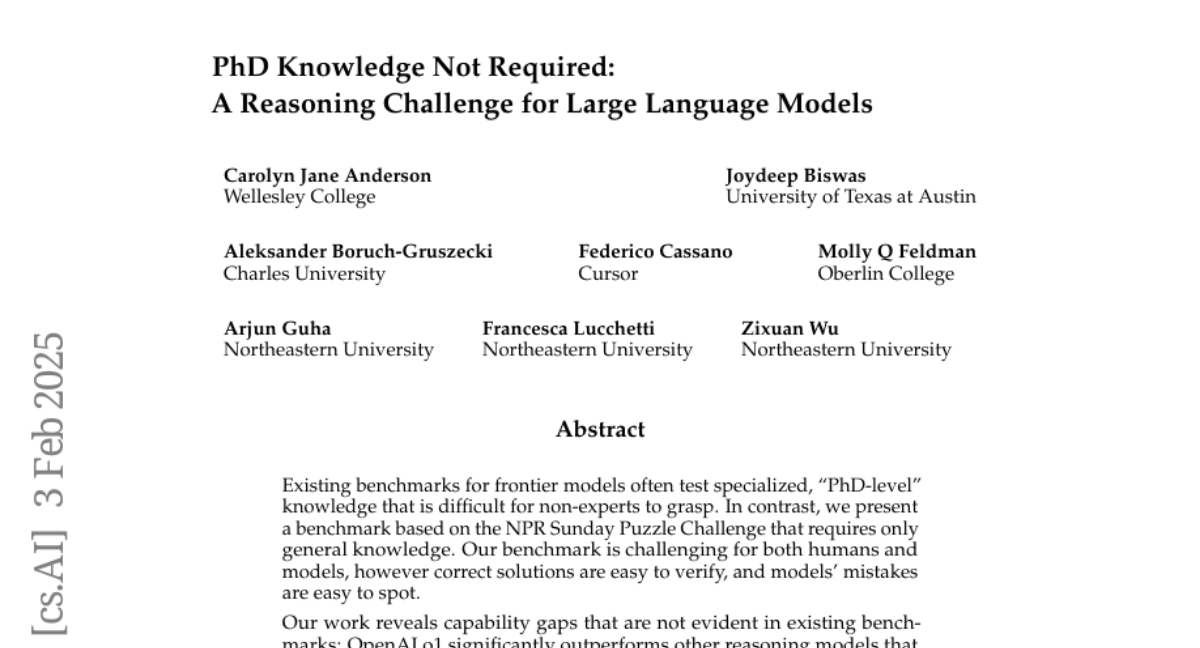
19. Lifelong Sequential Knowledge Editing without Model Degradation
π Keywords: Knowledge Editing, Norm-Growth, Overfitting, ENCORE
π‘ Category: Machine Learning
π Research Objective:
– The research aims to investigate the degradation of models during large-scale sequential knowledge edits and to develop a method to maintain model performance.
π οΈ Research Methods:
– The study involves analyzing locate-then-edit methods, identifying issues with overfitting and norm-growth, and introducing ENCORE, a method that incorporates early stopping and norm-constrained robust editing.
π¬ Research Conclusions:
– The introduction of ENCORE significantly reduces overfitting and norm-growth, supporting up to 10,000 sequential edits without compromising performance, and offers increased efficiency compared to MEMIT and AlphaEdit.
π Paper link: https://huggingface.co/papers/2502.01636
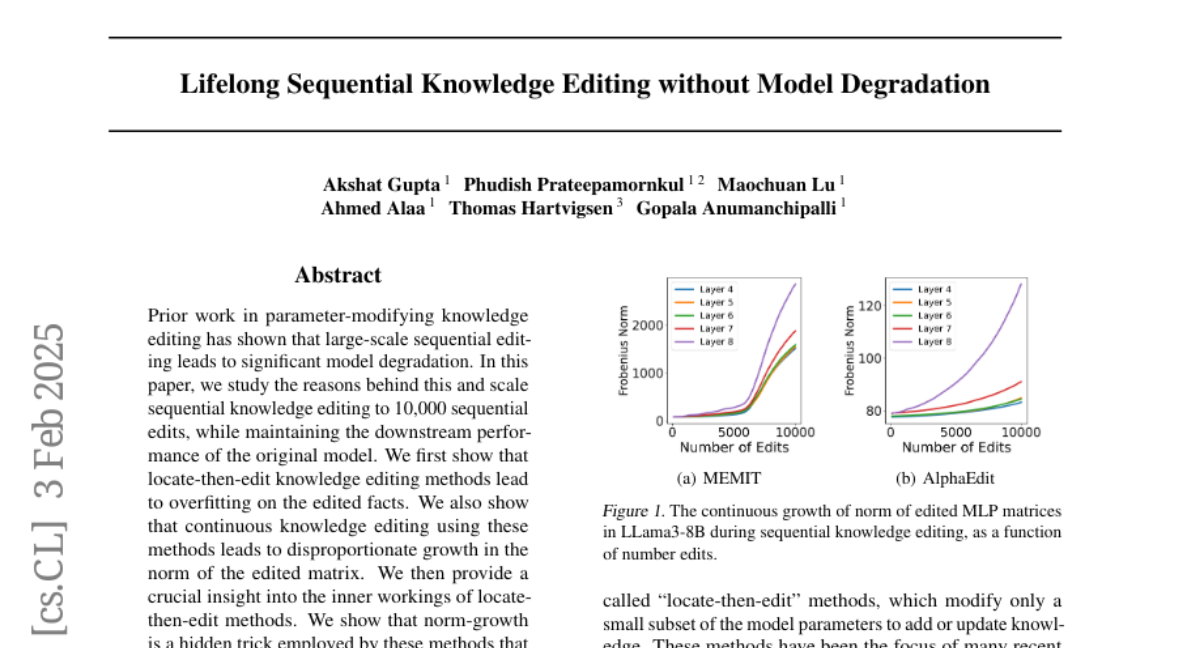
20. RandLoRA: Full-rank parameter-efficient fine-tuning of large models
π Keywords: Low-Rank Adaptation, RandLoRA, trainable parameters, full-rank updates, vision-language tasks
π‘ Category: Machine Learning
π Research Objective:
– The paper examines whether the performance gap in Low-Rank Adaptation (LoRA) is due to reduced trainable parameters or rank deficiency and introduces RandLoRA to address this.
π οΈ Research Methods:
– Introduces a new method, RandLoRA, which employs full-rank updates using learned linear combinations of low-rank, non-trainable random matrices, optimizing with diagonal scaling matrices.
π¬ Research Conclusions:
– Demonstrates that full-rank updates in RandLoRA are effective, significantly reducing the performance gap across vision, language, and especially vision-language tasks compared to standard fine-tuning.
π Paper link: https://huggingface.co/papers/2502.00987
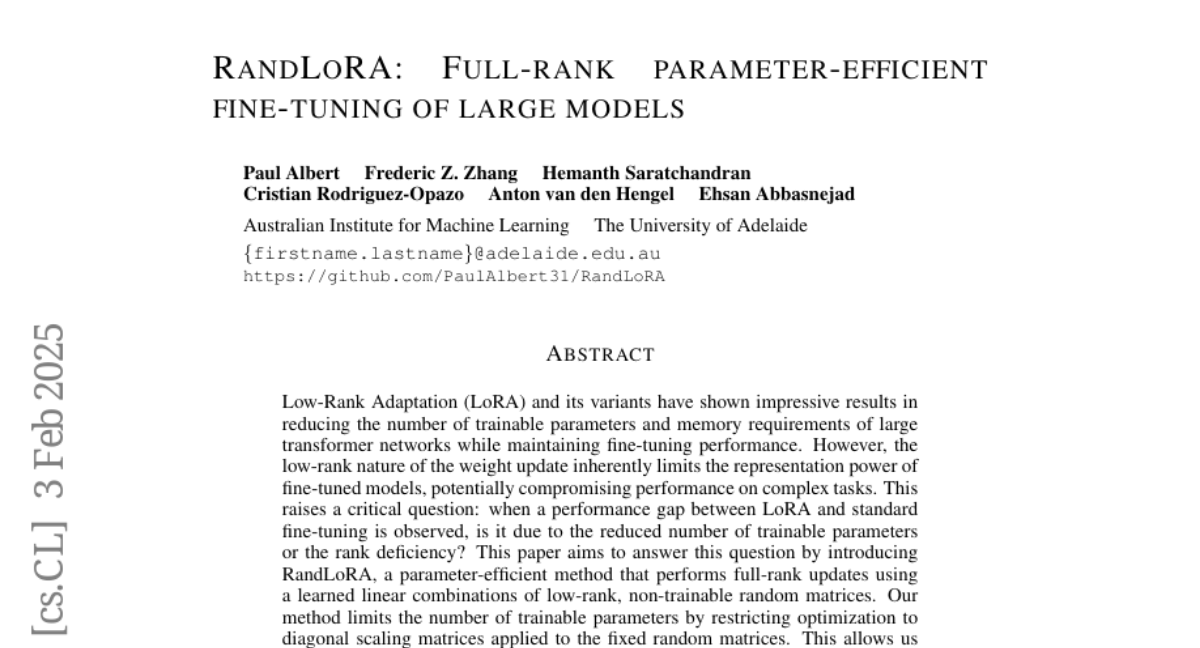
21. A Study on the Performance of U-Net Modifications in Retroperitoneal Tumor Segmentation
π Keywords: Automatic Segmentation, U-Net, Vision Transformer, xLSTM, ViLU-Net
π‘ Category: AI in Healthcare
π Research Objective:
– Address challenges in retroperitoneal tumor segmentation due to irregular shapes and high computational demands.
π οΈ Research Methods:
– Evaluate U-Net and its enhancements using elements like CNN, Vision Transformer, Mamba State Space Model, and Extended Long-Short Term Memory on various datasets.
π¬ Research Conclusions:
– The proposed ViLU-Net model with Vi-blocks improves segmentation efficiency, highlighting the effectiveness of xLSTM in reducing computational resources. The code is available on GitHub.
π Paper link: https://huggingface.co/papers/2502.00314
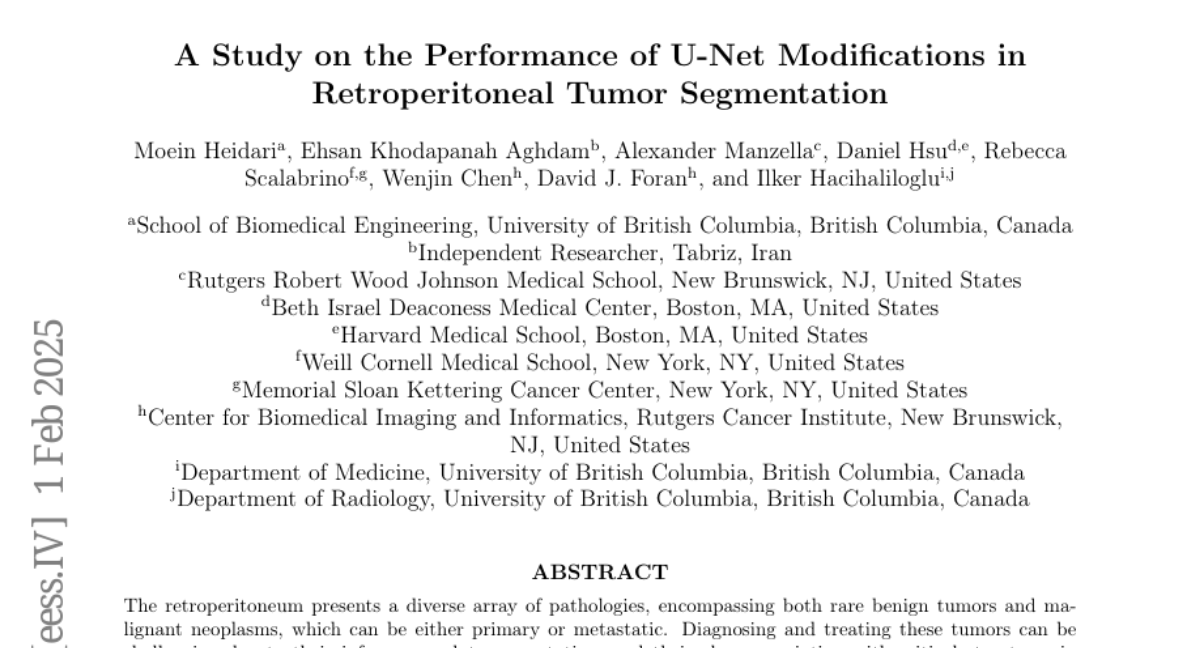
22. Learning to Generate Unit Tests for Automated Debugging
π Keywords: Unit Tests, Debugging, Automated Test Generation, Large Language Model, UTDebug
π‘ Category: AI Systems and Tools
π Research Objective:
– To address the trade-off in unit test generation by teaching LLMs to produce error-revealing inputs with correct outputs using the UTGen system.
π οΈ Research Methods:
– Integration of UTGen into a debugging pipeline, UTDebug, which uses generated tests to improve the debugging process and avoid overfitting by validating and back-tracking edits.
π¬ Research Conclusions:
– UTGen outperforms traditional test generation methods, and when combined with UTDebug, enhances pass@1 accuracy significantly on benchmark datasets.
π Paper link: https://huggingface.co/papers/2502.01619
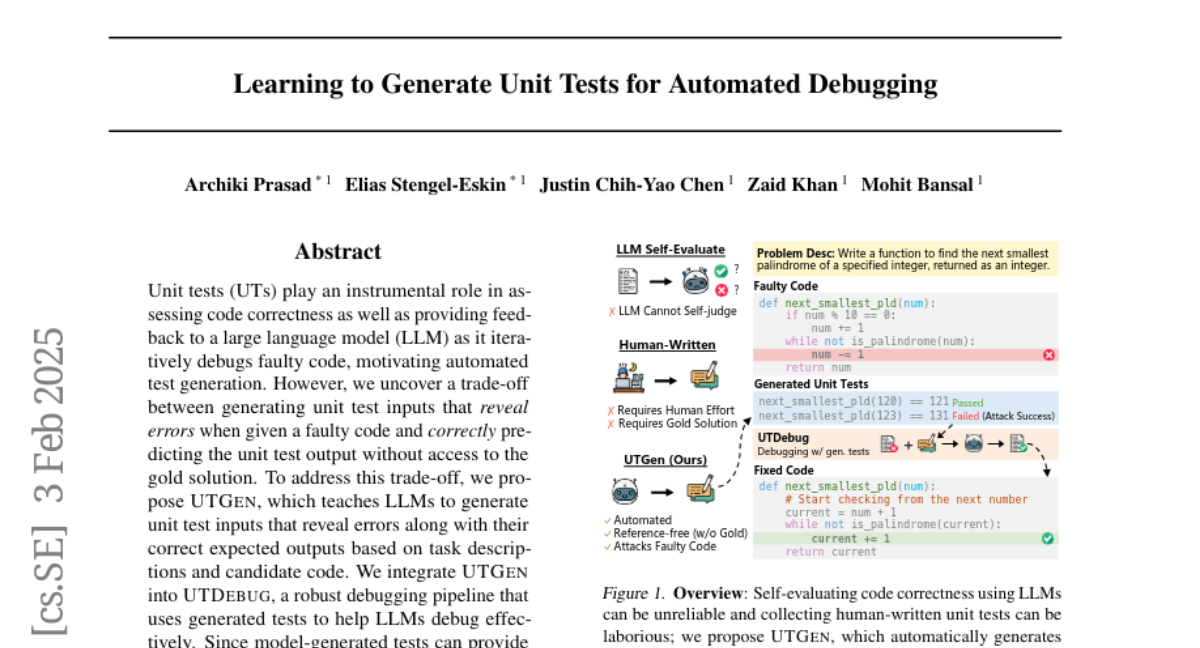
23. MakeAnything: Harnessing Diffusion Transformers for Multi-Domain Procedural Sequence Generation
π Keywords: AI Native, procedural generation, multi-domain dataset, diffusion transformer, image generation
π‘ Category: Generative Models
π Research Objective:
– The study aims to overcome three key challenges in AI-generated procedural tutorials, focusing on multi-task datasets, logical continuity, and domain generalization.
π οΈ Research Methods:
– The researchers proposed a multi-domain dataset encompassing 21 tasks and developed the MakeAnything framework using the diffusion transformer and asymmetric low-rank adaptation for improved image generation.
π¬ Research Conclusions:
– MakeAnything surpasses existing methods in procedural generation tasks, establishing new performance benchmarks through extensive experiments.
π Paper link: https://huggingface.co/papers/2502.01572
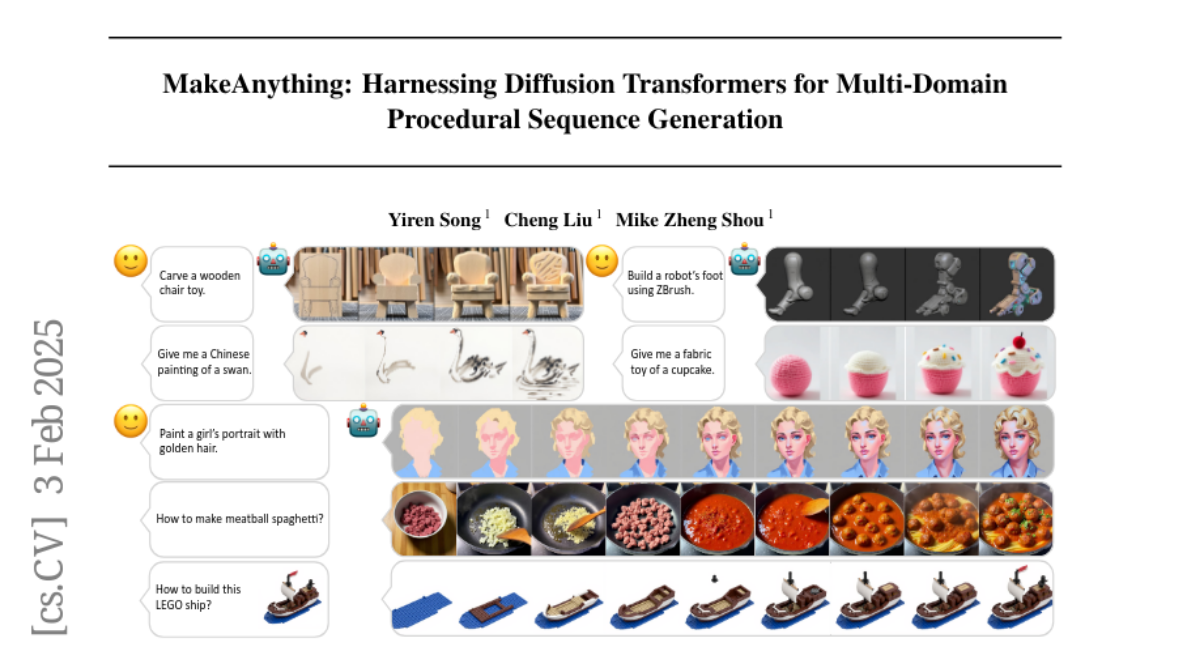
24. Current Pathology Foundation Models are unrobust to Medical Center Differences
π Keywords: Pathology Foundation Models, Robustness, Confounding Features, Cancer-Type Classification
π‘ Category: AI in Healthcare
π Research Objective:
– Introduce the Robustness Index to measure whether pathology foundation models focus on biological features over confounding features like medical center signatures.
π οΈ Research Methods:
– Evaluation of ten publicly available pathology FMs and a quantitative approach to measure the impact of medical center differences on model prediction performance.
π¬ Research Conclusions:
– Current models largely represent medical center characteristics, with only one model achieving a robustness index greater than one, indicating a slight dominance of biological features over confounding ones.
– Classification errors are specifically attributable to confounding features, and FM embedding spaces are more organized by medical centers than biological factors.
π Paper link: https://huggingface.co/papers/2501.18055
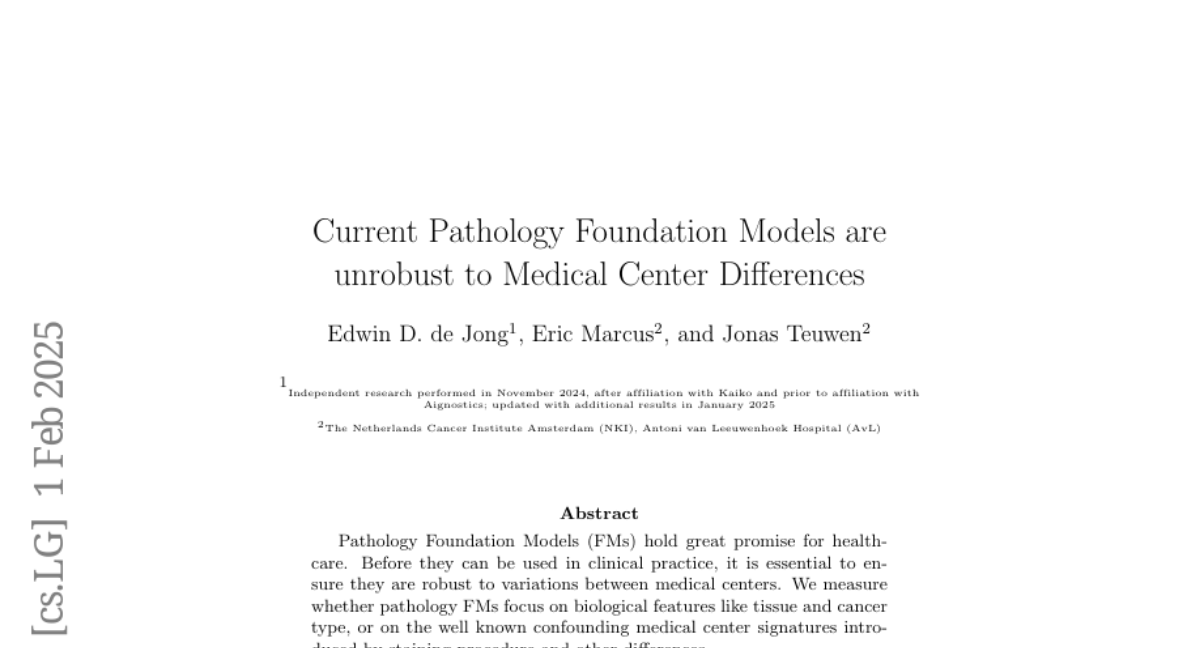
25. Language Models Prefer What They Know: Relative Confidence Estimation via Confidence Preferences
π Keywords: Language models, Confidence estimation, Confidence scores, Relative confidence, Uncertainty
π‘ Category: Natural Language Processing
π Research Objective:
– To improve the reliability of confidence estimates in Language models by shifting from absolute to relative confidence estimation.
π οΈ Research Methods:
– Employed relative confidence estimation where questions are matched against each other, allowing the model to make comparative confidence judgments. Used rank aggregation methods like Elo rating and Bradley-Terry to compute confidence scores.
π¬ Research Conclusions:
– Relative confidence estimation outperforms absolute confidence estimation by providing more reliable confidence scores, showing an average gain of 3.5% in selective classification AUC and a 1.7% gain over self-consistency methods across extensive testing with five state-of-the-art LMs on various challenging tasks.
π Paper link: https://huggingface.co/papers/2502.01126
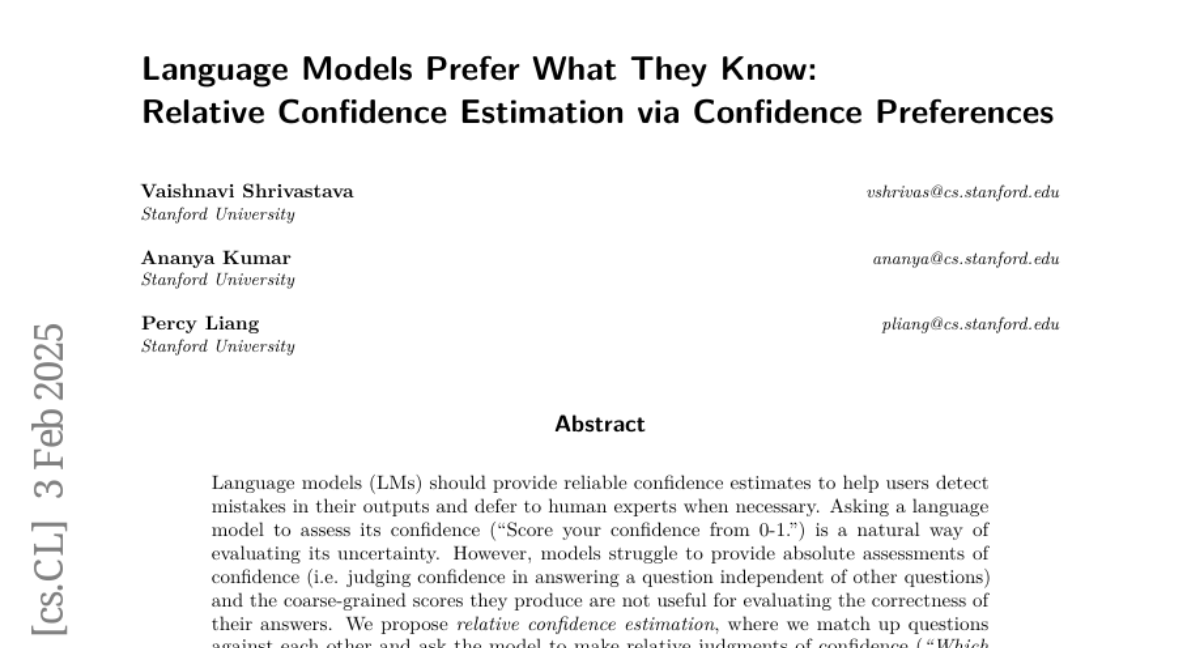