AI Native Daily Paper Digest – 20241212
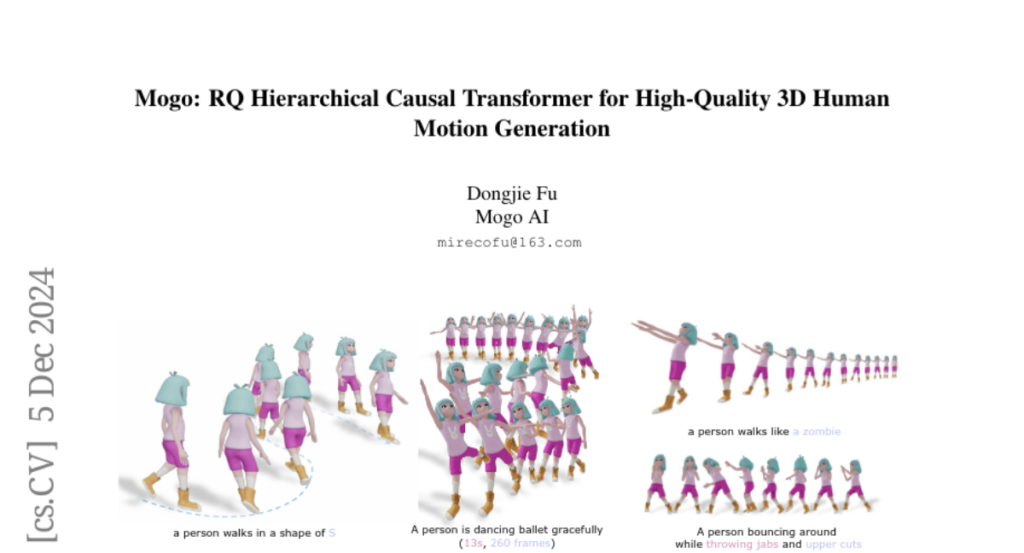
1. SynCamMaster: Synchronizing Multi-Camera Video Generation from Diverse Viewpoints
π Keywords: video diffusion models, 3D consistency, virtual filming, 6 DoF, SynCamVideo-Dataset
π‘ Category: Computer Vision
π Research Objective:
– Explore the potential of video diffusion models to maintain dynamic consistency across various viewpoints, focusing on open-world video generation with arbitrary viewpoints.
π οΈ Research Methods:
– Introduce a plug-and-play module to enhance a pre-trained text-to-video model for consistent multi-camera video generation, utilizing a multi-view synchronization module and a hybrid training scheme with multi-camera images and monocular videos.
π¬ Research Conclusions:
– Successfully developed a method that allows for multi-view synchronized video generation, releasing a dataset named SynCamVideo-Dataset, enabling novel viewpoint re-rendering.
π Paper link: https://huggingface.co/papers/2412.07760
2. LAION-SG: An Enhanced Large-Scale Dataset for Training Complex Image-Text Models with Structural Annotations
π Keywords: Text-to-Image Generation, LAION-SG, Scene Graphs, SDXL-SG, Compositional Image Generation
π‘ Category: Generative Models
π Research Objective:
– To improve compositional image generation through precise inter-object relationship annotations in datasets.
π οΈ Research Methods:
– Construction of the LAION-SG dataset with structural annotations of scene graphs and training the SDXL-SG model using this dataset.
π¬ Research Conclusions:
– Models trained on LAION-SG demonstrate significant performance improvements in complex scene generation, and a new benchmark, CompSG-Bench, was introduced to evaluate models in compositional image generation.
π Paper link: https://huggingface.co/papers/2412.08580
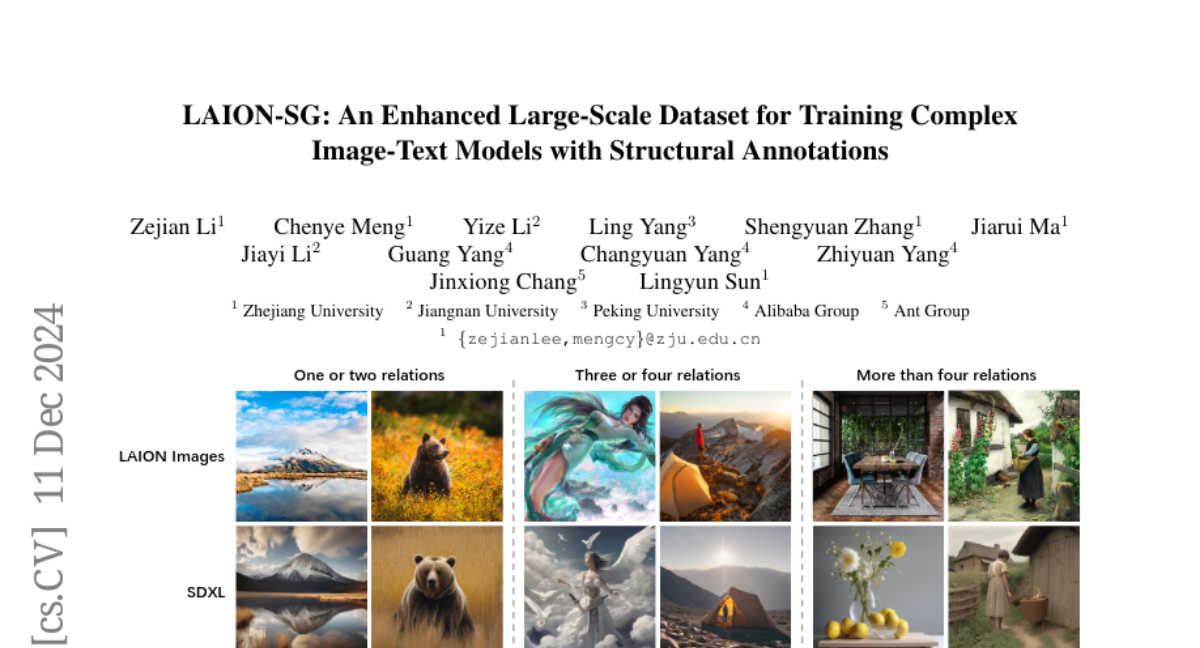
3. POINTS1.5: Building a Vision-Language Model towards Real World Applications
π Keywords: Vision-language models, Dynamic high resolution, Bilingual support, Filtering methods
π‘ Category: Multi-Modal Learning
π Research Objective:
– Introduce a new vision-language model, POINTS1.5, to excel in real-world applications.
π οΈ Research Methods:
– Incorporate a NaViT-style vision encoder for handling images of any resolution.
– Add bilingual capability, with a focus on enhancing performance in Chinese through comprehensive image data collection and annotation.
– Implement and evaluate filtering methods to enhance visual instruction tuning datasets.
π¬ Research Conclusions:
– POINTS1.5 shows significant performance improvements over its predecessor and ranks first on the OpenCompass leaderboard for models with fewer than 10 billion parameters.
π Paper link: https://huggingface.co/papers/2412.08443
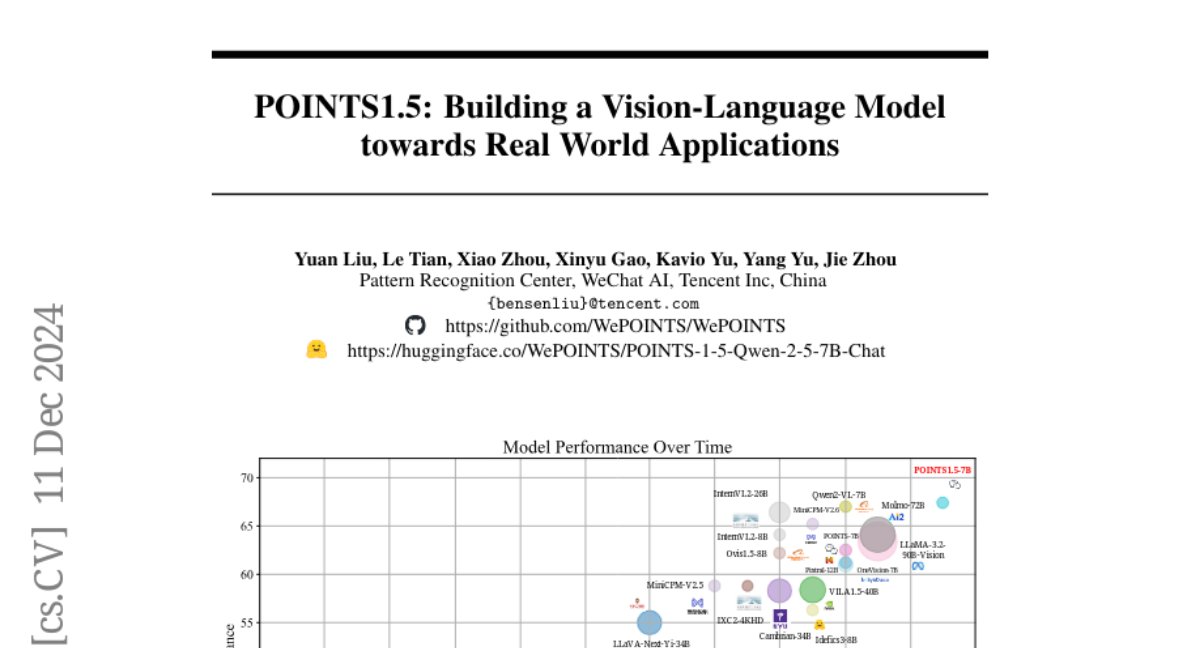
4. Learning Flow Fields in Attention for Controllable Person Image Generation
π Keywords: Controllable person image generation, flow fields in attention, fine-grained textural details, diffusion-based baseline
π‘ Category: Generative Models
π Research Objective:
– To enable controllable person image generation with precise appearance or pose control using reference images.
π οΈ Research Methods:
– Introduction of a novel approach called learning flow fields in attention (Leffa) which uses a regularization loss in the attention map of a diffusion-based model to guide correct reference matching.
π¬ Research Conclusions:
– Leffa outperforms previous methods, effectively reducing distortion in fine-grained textural details while maintaining high-quality images, and can enhance the performance of other diffusion models.
π Paper link: https://huggingface.co/papers/2412.08486
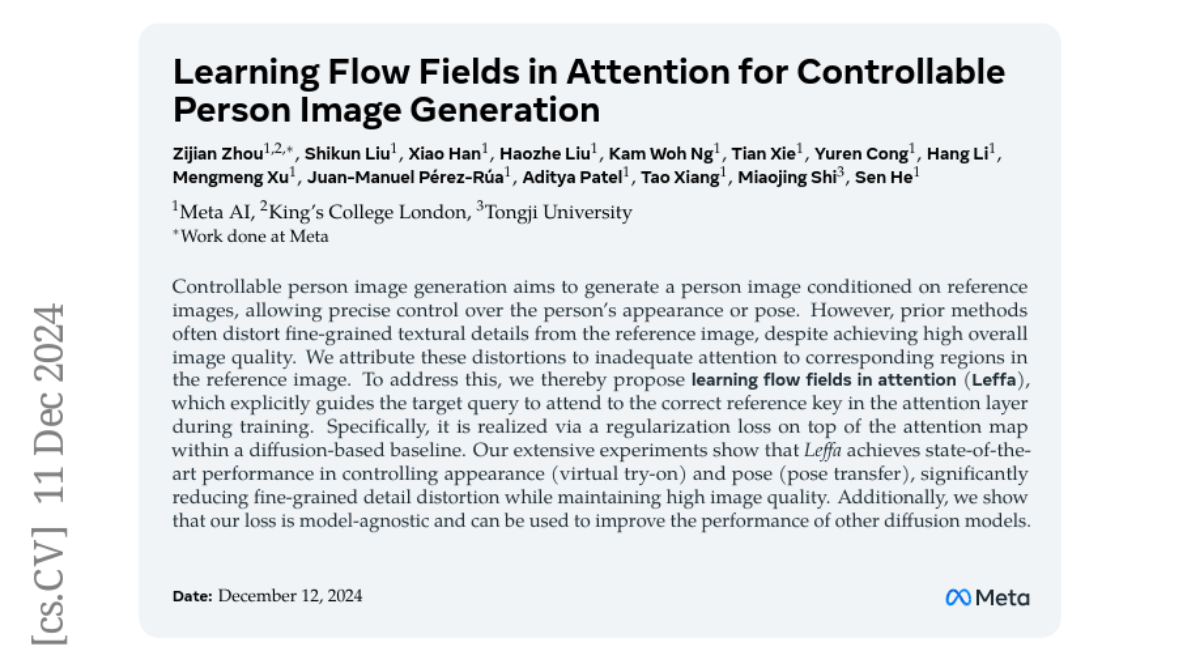
5. StyleMaster: Stylize Your Video with Artistic Generation and Translation
π Keywords: Style control, Video generation, Texture features, Global style extraction, StyleMaster
π‘ Category: Generative Models
π Research Objective:
– The study aims to enhance style control in video generation models by addressing content leakage and improving style transfer fidelity.
π οΈ Research Methods:
– The proposed method focuses on filtering content-related patches while retaining style ones using prompt-patch similarity. It also involves generating a paired style dataset for global style extraction and training a lightweight motion adapter for seamless image-to-video stylization.
π¬ Research Conclusions:
– StyleMaster significantly improves style resemblance and temporal coherence in video generation, outperforming competitors in producing high-quality stylized videos with enhanced alignment to reference images.
π Paper link: https://huggingface.co/papers/2412.07744
6. StreamChat: Chatting with Streaming Video
π Keywords: StreamChat, Large Multimodal Models, Streaming Video, Crossattention-based Architecture
π‘ Category: Multi-Modal Learning
π Research Objective:
– The paper introduces StreamChat to enhance interaction capabilities of Large Multimodal Models with streaming video content by updating visual context dynamically.
π οΈ Research Methods:
– Utilizes a crossattention-based architecture to efficiently process dynamic streaming inputs and employs a new dense instruction dataset with a parallel 3D-RoPE mechanism for better encoding temporal information.
π¬ Research Conclusions:
– StreamChat demonstrates competitive performance on image and video benchmarks, and shows superior streaming interaction capabilities compared to state-of-the-art video LMM.
π Paper link: https://huggingface.co/papers/2412.08646
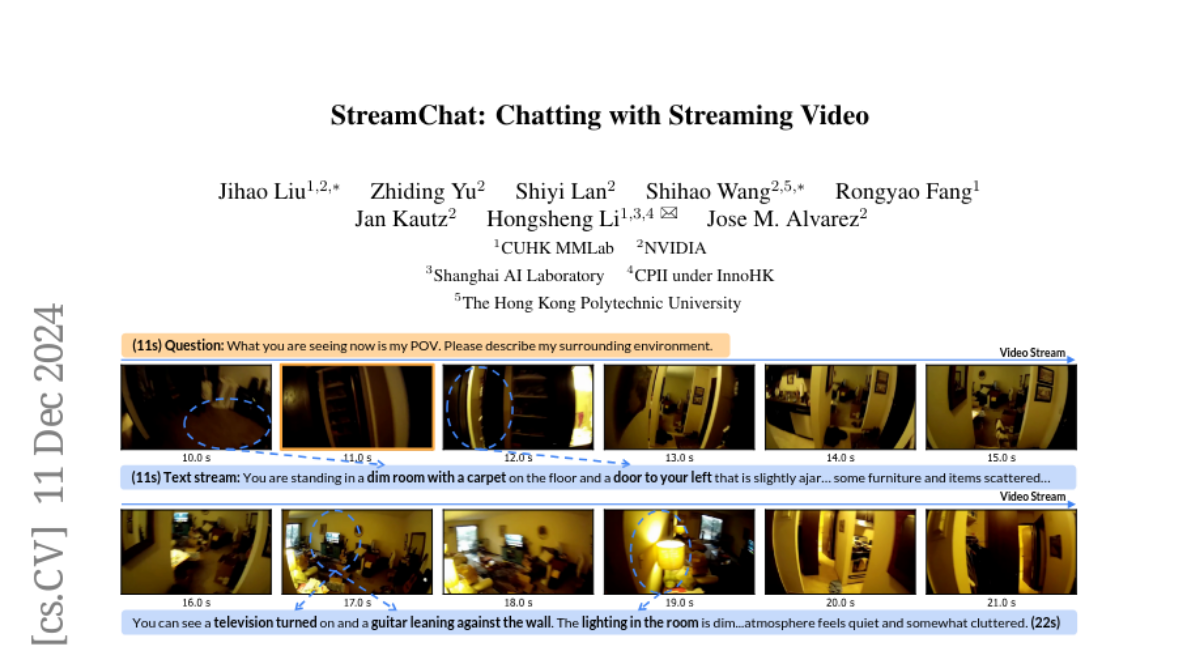
7. 3DSRBench: A Comprehensive 3D Spatial Reasoning Benchmark
π Keywords: 3D spatial reasoning, LMMs, 3DSRBench, FlipEval, camera viewpoints
π‘ Category: Computer Vision
π Research Objective:
– Introduction of 3DSRBench for comprehensive evaluation of 3D spatial reasoning in LMMs.
π οΈ Research Methods:
– Utilization of a balanced data distribution and the novel FlipEval strategy; inclusion of paired image subsets to assess viewpoint robustness.
π¬ Research Conclusions:
– Identification of limitations in LMMs regarding 3D awareness and performance degradation with uncommon viewpoints; insights offered for further development of LMMs in 3D reasoning.
π Paper link: https://huggingface.co/papers/2412.07825
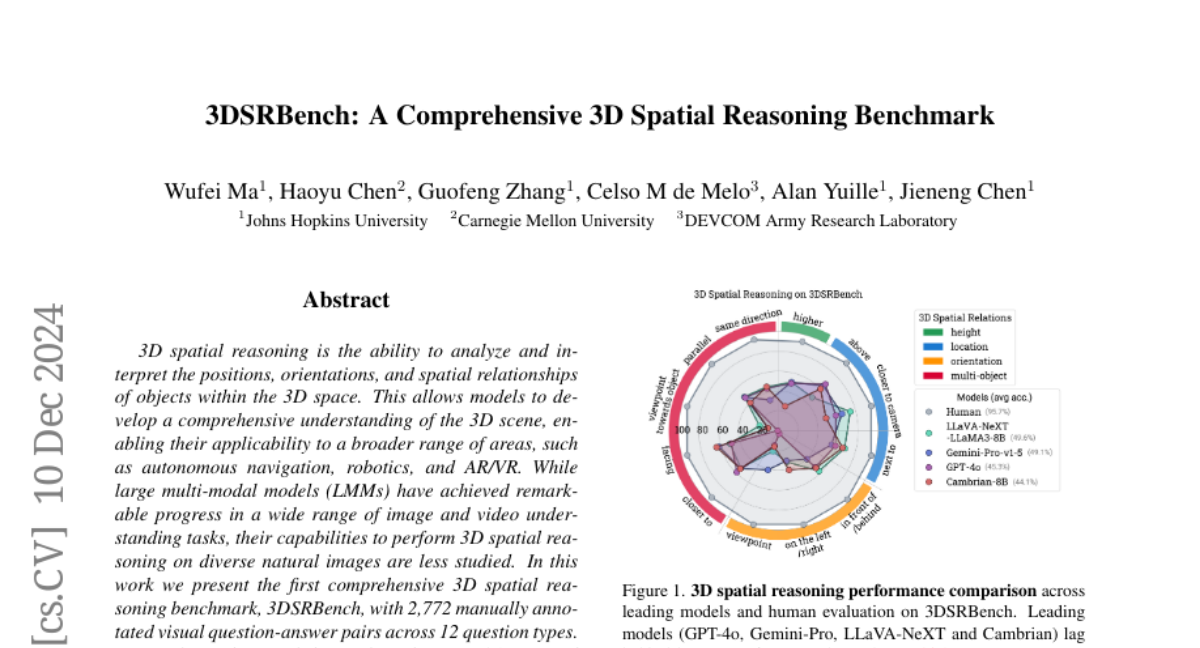
8. Generative Densification: Learning to Densify Gaussians for High-Fidelity Generalizable 3D Reconstruction
π Keywords: Generalized feed-forward Gaussian models, sparse-view 3D reconstruction, Generative Densification
π‘ Category: Computer Vision
π Research Objective:
– To propose Generative Densification for enhancing fine detail representation in generalized feed-forward Gaussian models for sparse-view 3D reconstruction.
π οΈ Research Methods:
– Introduces a method to up-sample feature representations in a single forward pass instead of iterative splitting in 3D-GS.
π¬ Research Conclusions:
– Demonstrates superior performance and detail representation in object-level and scene-level reconstruction tasks, outperforming state-of-the-art models with comparable or smaller sizes.
π Paper link: https://huggingface.co/papers/2412.06234
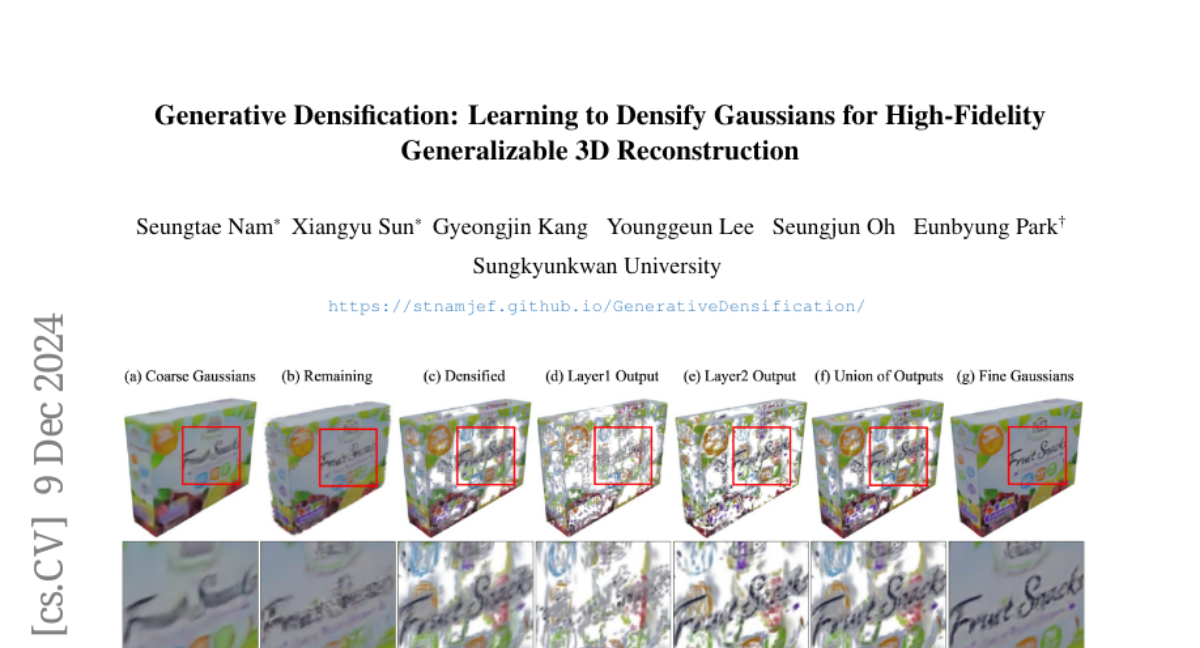
9. The BrowserGym Ecosystem for Web Agent Research
π Keywords: BrowserGym, web agents, Large Language Models, benchmarking
π‘ Category: AI Systems and Tools
π Research Objective:
– To create a unified environment for evaluating web agents, facilitating standardized benchmarking and reducing complexity in LLM-driven web automation.
π οΈ Research Methods:
– Development of BrowserGym ecosystem, complemented by AgentLab for agent creation, and conducting a large-scale, multi-benchmark experiment.
π¬ Research Conclusions:
– Highlighted discrepancies in LLM performance, with Claude-3.5-Sonnet outperforming in most benchmarks except vision-related tasks dominated by GPT-4o; emphasized the challenges in developing effective web agents due to complex web environments.
π Paper link: https://huggingface.co/papers/2412.05467
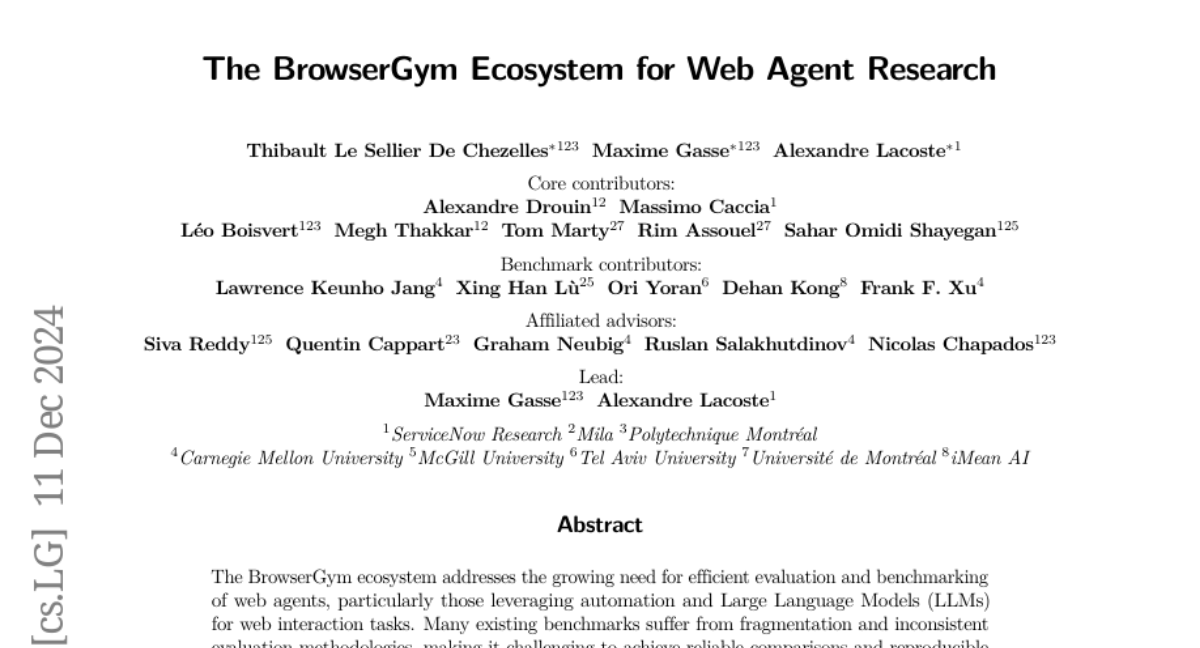
10. FlowEdit: Inversion-Free Text-Based Editing Using Pre-Trained Flow Models
π Keywords: AI Native, text-to-image, diffusion model, FlowEdit
π‘ Category: Generative Models
π Research Objective:
– The research introduces FlowEdit, a text-based editing method designed for pre-trained text-to-image (T2I) flow models.
π οΈ Research Methods:
– Constructs an ODE for mapping between source and target distributions without inversion or optimization, achieving a lower transport cost.
π¬ Research Conclusions:
– FlowEdit delivers state-of-the-art results and is demonstrated using Stable Diffusion 3 and FLUX. The proposed method is model agnostic and incurs less transport cost compared to inversion approaches.
π Paper link: https://huggingface.co/papers/2412.08629
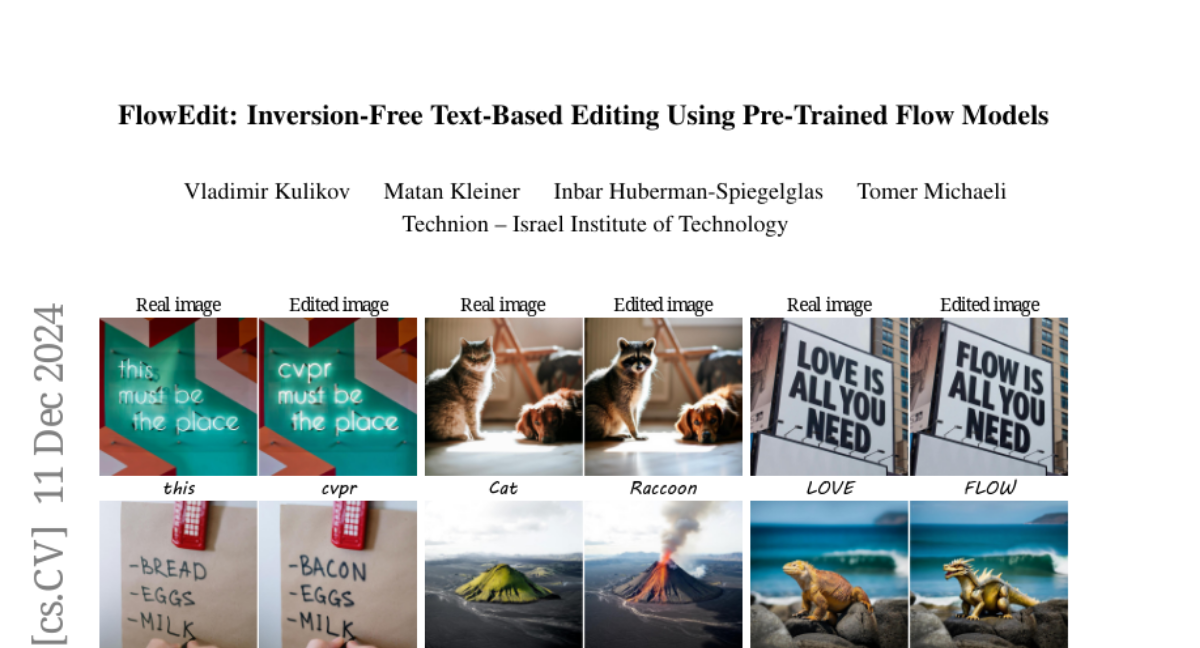
11. Mogo: RQ Hierarchical Causal Transformer for High-Quality 3D Human Motion Generation
π Keywords: Text-to-Motion Generation, BERT-type Models, GPT-type Models, Hierarchical Causal Transformer
π‘ Category: Generative Models
π Research Objective:
– To enhance the quality of text-to-motion generation models by combining GPT-type models’ streaming capabilities with BERT-type models’ quality.
π οΈ Research Methods:
– Introduction of a novel architecture named Mogo, combining RVQ-VAE and Hierarchical Causal Transformer to generate lifelike 3D human motions.
π¬ Research Conclusions:
– Mogo surpasses existing models by generating longer motion sequences and achieving superior FID scores in both in-distribution and out-of-distribution scenarios.
π Paper link: https://huggingface.co/papers/2412.07797
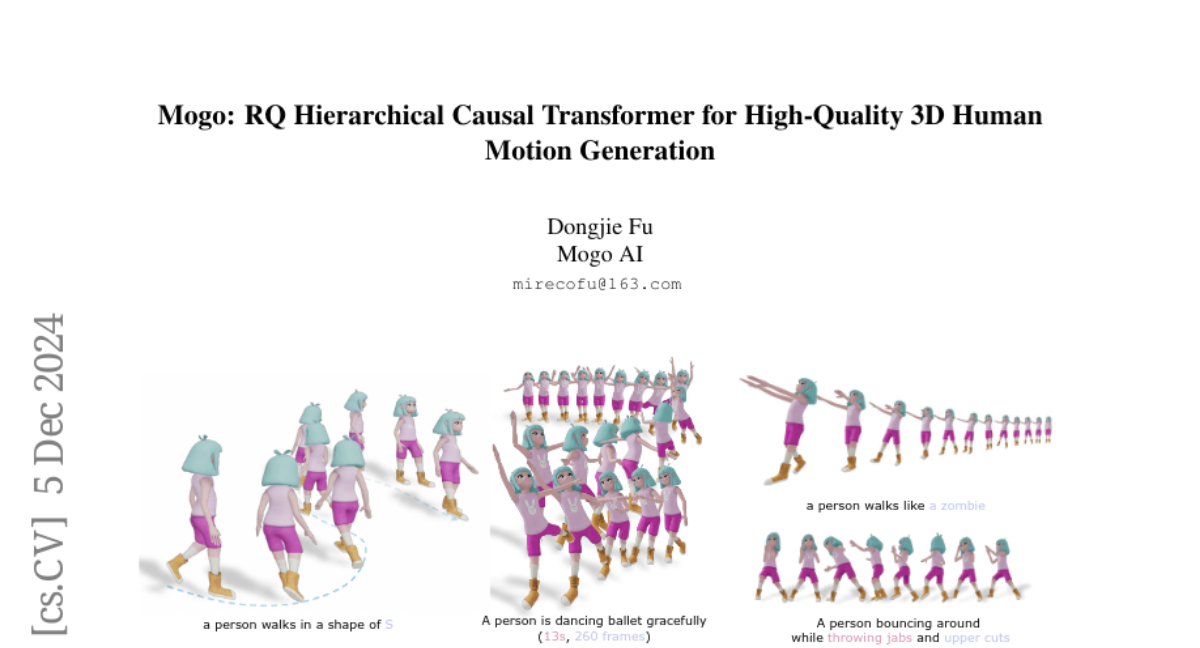
12. Track4Gen: Teaching Video Diffusion Models to Track Points Improves Video Generation
π Keywords: Video Generation, Spatial Tracking, Appearance Drift, Video Diffusion, Visual Coherence
π‘ Category: Generative Models
π Research Objective:
– The study aims to address appearance drift in video generators by integrating point tracking for better spatial supervision.
π οΈ Research Methods:
– The proposed Track4Gen framework combines video diffusion loss with point tracking using minimal architectural changes to existing video generation models, specifically leveraging Stable Video Diffusion.
π¬ Research Conclusions:
– Track4Gen demonstrates effective reduction of appearance drift, achieving temporally stable and visually coherent video output by unifying video generation and point tracking tasks.
π Paper link: https://huggingface.co/papers/2412.06016
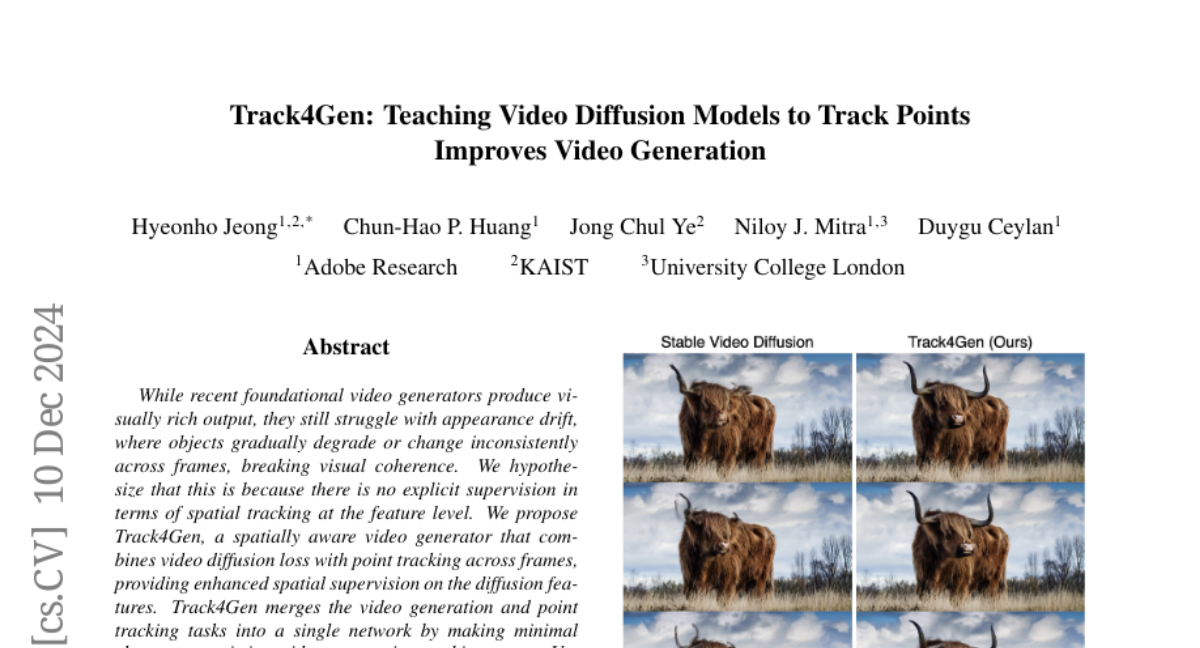
13. KaSA: Knowledge-Aware Singular-Value Adaptation of Large Language Models
π Keywords: PEFT, LLM, Knowledge-aware Singular-value Adaptation, SVD, LoRA
π‘ Category: Natural Language Processing
π Research Objective:
– Introduce Knowledge-aware Singular-value Adaptation (KaSA) to enhance model performance by dynamically activating relevant knowledge for specific tasks.
π οΈ Research Methods:
– Utilized Singular Value Decomposition (SVD) with knowledge-aware singular values in AI Native, applying the approach across a range of large language models (LLMs) on various tasks.
π¬ Research Conclusions:
– Demonstrated that KaSA consistently outperforms other PEFT methods and baselines in 16 benchmarks and 4 synthetic datasets, highlighting its efficiency and adaptability.
π Paper link: https://huggingface.co/papers/2412.06071
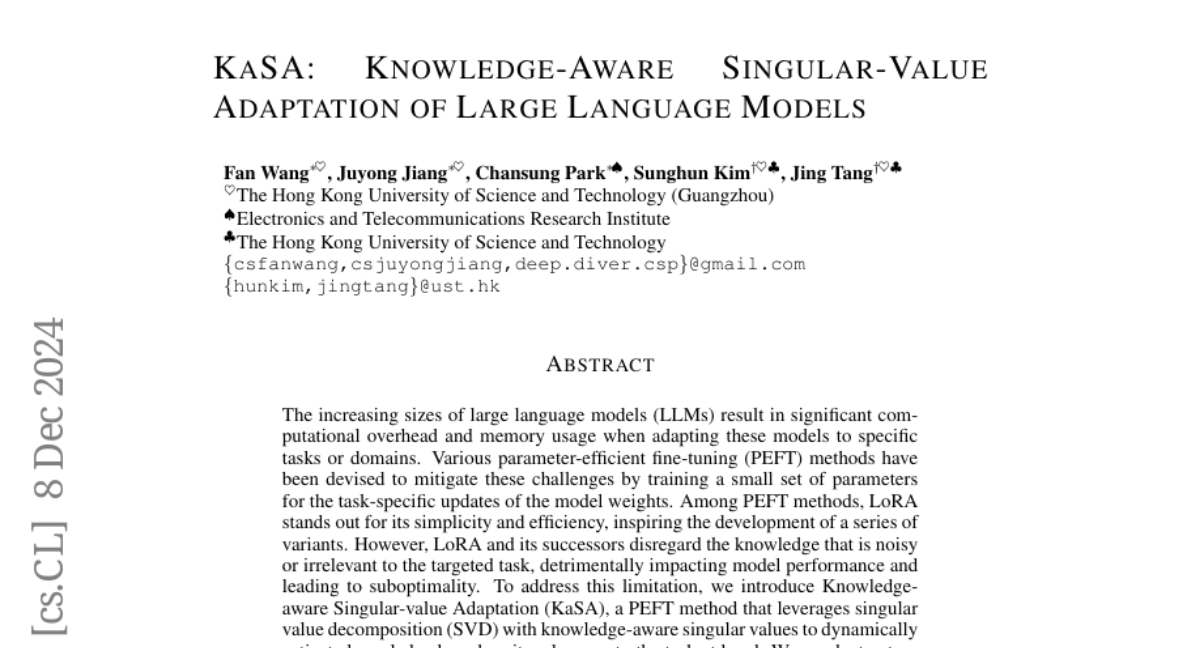
14. StyleStudio: Text-Driven Style Transfer with Selective Control of Style Elements
π Keywords: Text-driven style transfer, Reference image, Cross-modal Adaptive Instance Normalization, Style-based Classifier-Free Guidance
π‘ Category: Multi-Modal Learning
π Research Objective:
– To address challenges in text-driven style transfer, specifically overfitting, limited stylistic control, and misalignment with textual content.
π οΈ Research Methods:
– Introducing a cross-modal Adaptive Instance Normalization mechanism to enhance the integration of style and text features.
– Developing a Style-based Classifier-Free Guidance approach for selective control over stylistic elements.
– Incorporating a teacher model in early generation stages to stabilize spatial layouts and mitigate artifacts.
π¬ Research Conclusions:
– Demonstrated significant improvements in style transfer quality and alignment with textual prompts, and the approach can be integrated into existing frameworks without fine-tuning.
π Paper link: https://huggingface.co/papers/2412.08503
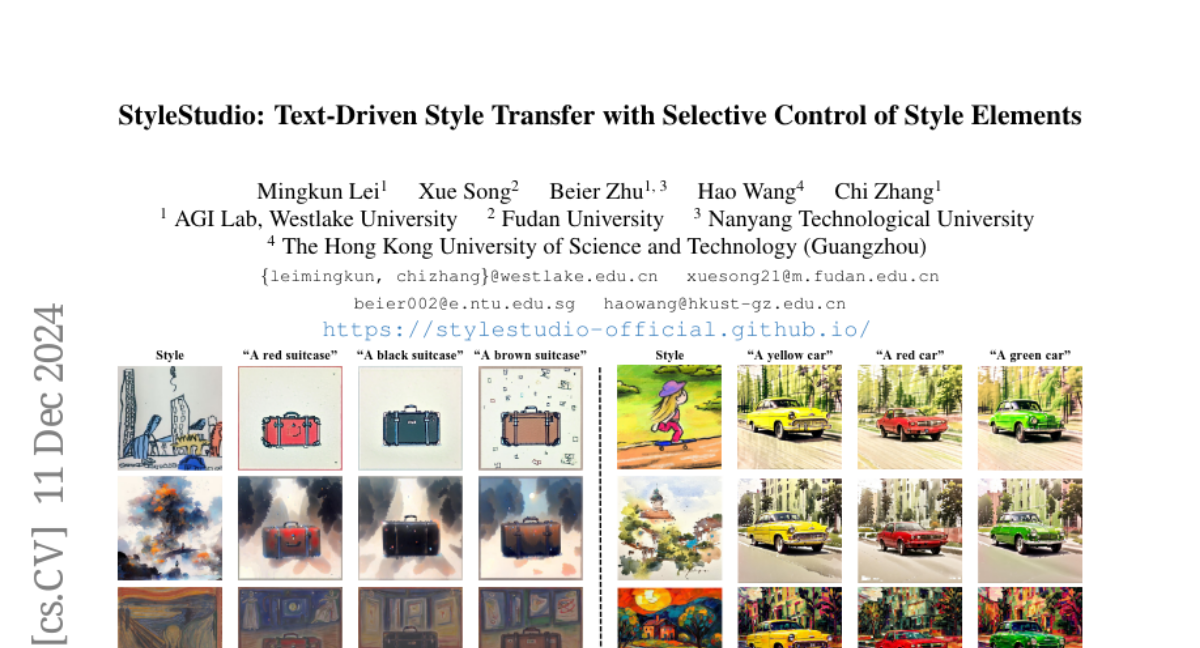
15. I Don’t Know: Explicit Modeling of Uncertainty with an [IDK] Token
π Keywords: Large Language Models, hallucinations, calibration method, [IDK] token
π‘ Category: Natural Language Processing
π Research Objective:
– Propose a novel calibration method to reduce hallucinations in Large Language Models by incorporating an [IDK] token.
π οΈ Research Methods:
– Introduce an objective function that redistributes probability to the [IDK] token when predictions are incorrect. Evaluate performance across various model architectures and tasks.
π¬ Research Conclusions:
– The method enables models to explicitly express uncertainty with minimal loss of encoded knowledge. Detailed analysis of precision-recall tradeoff is provided.
π Paper link: https://huggingface.co/papers/2412.06676
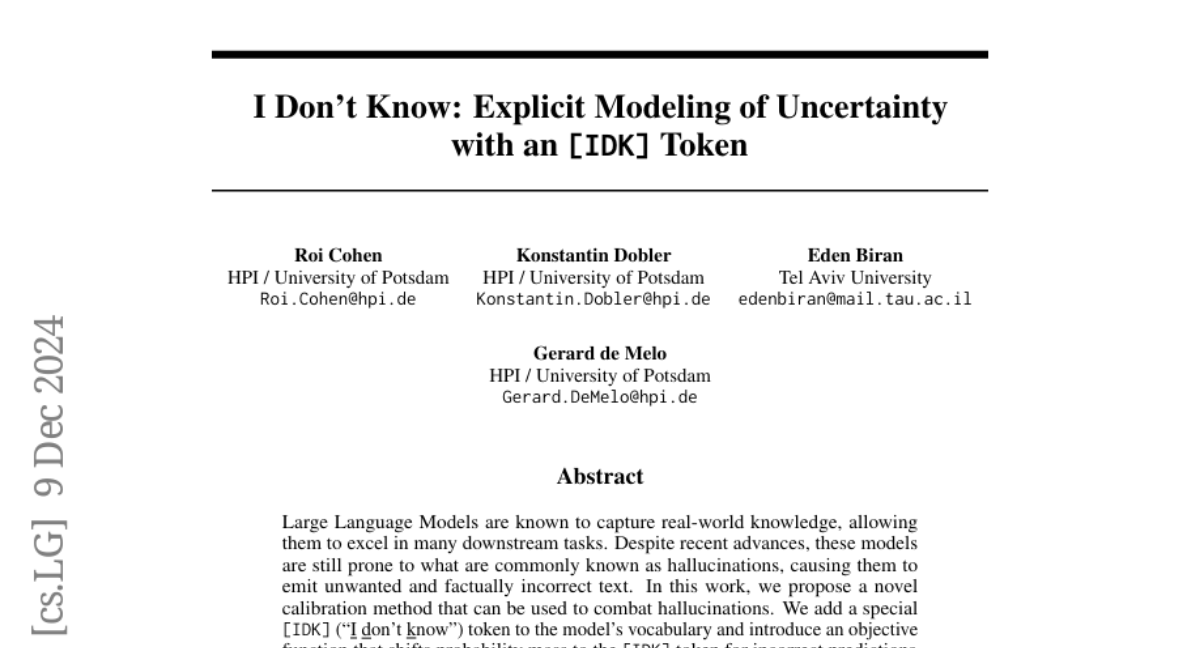
16. MIT-10M: A Large Scale Parallel Corpus of Multilingual Image Translation
π Keywords: Image Translation, Multilingual, MIT-10M, Dataset improvement, Performance
π‘ Category: Natural Language Processing
π Research Objective:
– The paper aims to address the limitations in scale, diversity, and quality of existing Image Translation datasets by introducing MIT-10M, a large-scale multilingual corpus.
π οΈ Research Methods:
– Extensive data cleaning and multilingual translation validation were performed on the dataset, which consists of over 10 million image-text pairs from real-world data, encompassing 28 categories and 14 languages.
π¬ Research Conclusions:
– MIT-10M demonstrates higher adaptability and significantly improves model performance in complex and real-world image translation tasks, with performance tripling when models are fine-tuned with this dataset compared to the baseline.
π Paper link: https://huggingface.co/papers/2412.07147
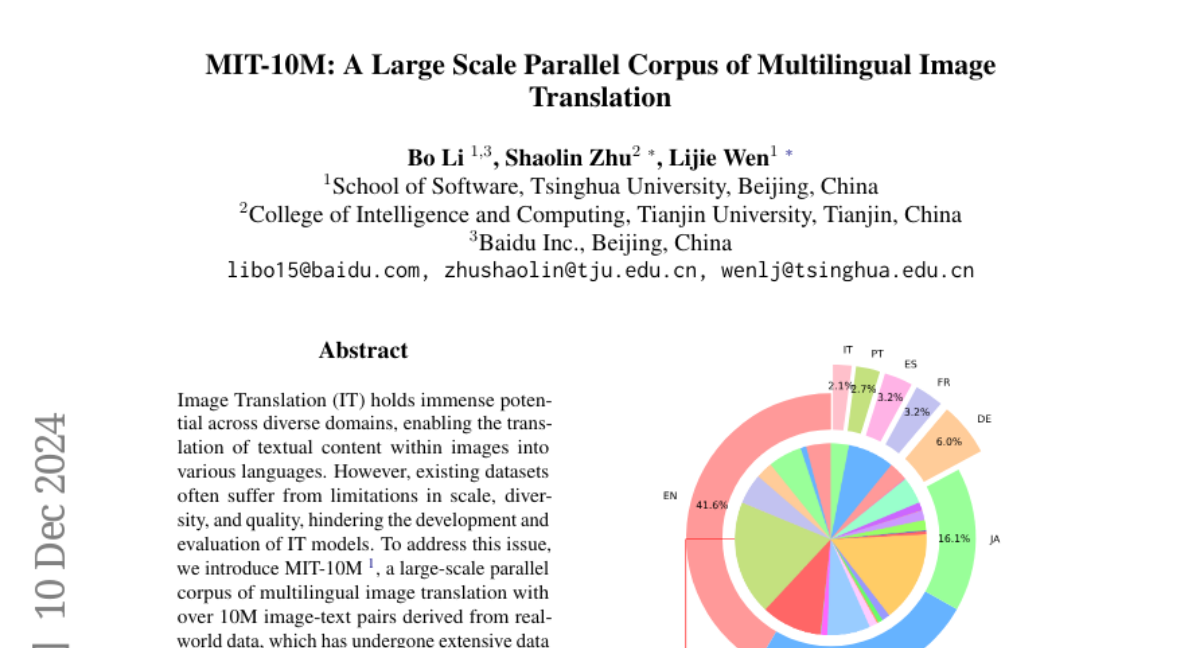
17. Bootstrapping Language-Guided Navigation Learning with Self-Refining Data Flywheel
π Keywords: Self-Refining Data Flywheel, Embodied AI, Navigational instruction-trajectory pairs
π‘ Category: Natural Language Processing
π Research Objective:
– Introduce a novel Self-Refining Data Flywheel (SRDF) to enhance data quality and scale for training language-instructed agents.
π οΈ Research Methods:
– Implements an iterative refinement process using a collaboration between an instruction generator and a navigator, eliminating human-in-loop annotation.
π¬ Research Conclusions:
– Demonstrated significant performance improvement of navigators and generators, with superior results in standard benchmarks such as R2R and SPICE, and generalization across diverse tasks surpassing state-of-the-art methods.
π Paper link: https://huggingface.co/papers/2412.08467
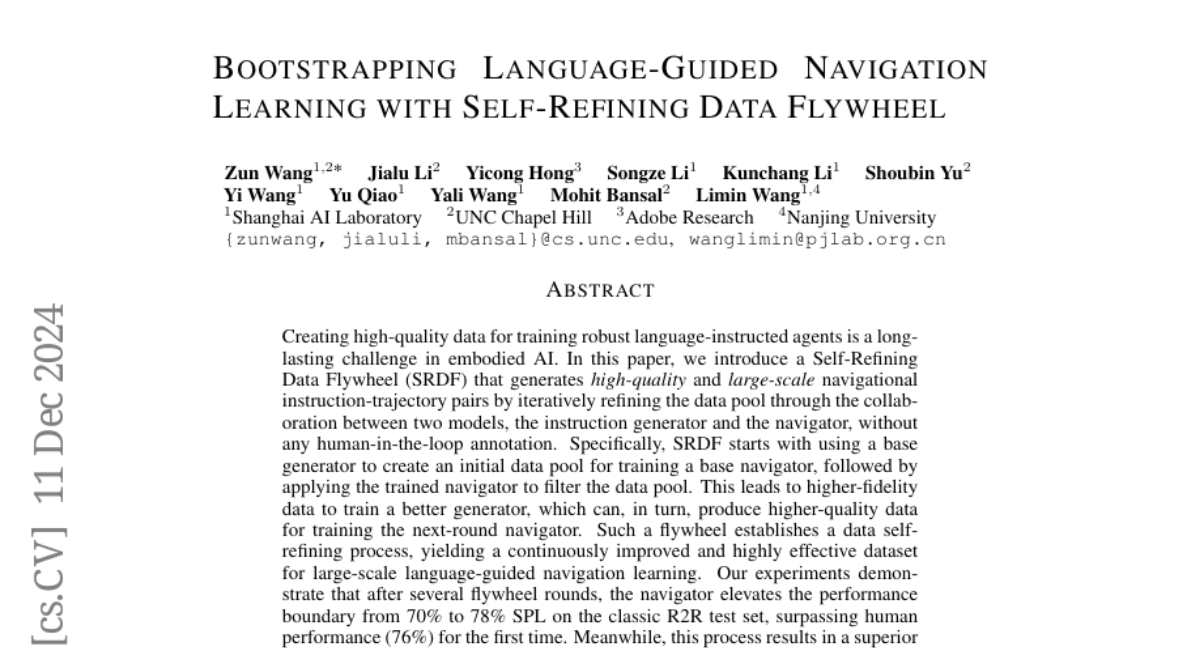