AI Native Daily Paper Digest – 20241213
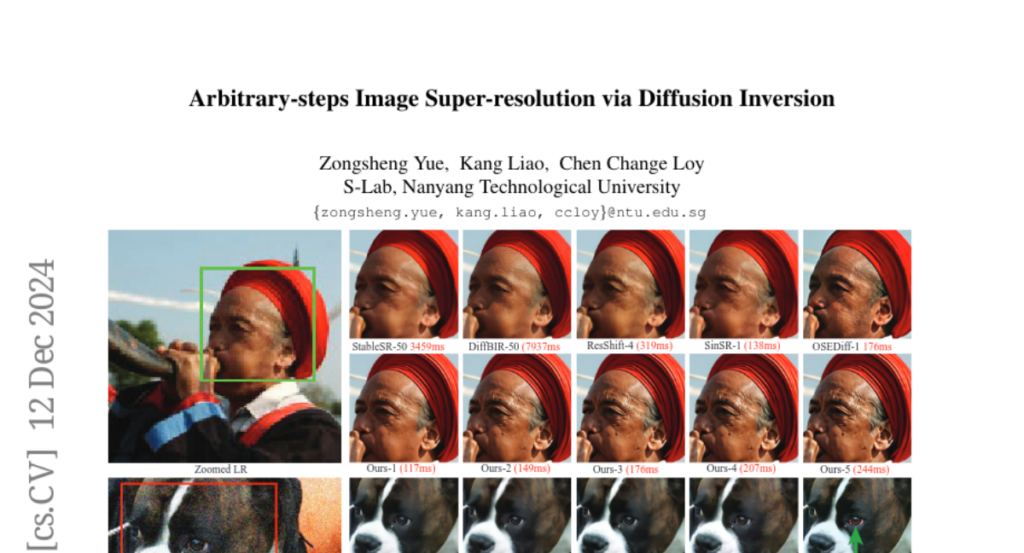
1. InternLM-XComposer2.5-OmniLive: A Comprehensive Multimodal System for Long-term Streaming Video and Audio Interactions
๐ Keywords: Specialized Generalist AI, Multimodal Large Language Models, Streaming Perception, Memory, Reasoning
๐ก Category: Multi-Modal Learning
๐ Research Objective:
– The paper aims to overcome limitations in current Multimodal Large Language Models (MLLMs) regarding continuous streaming perception, memory, and reasoning.
๐ ๏ธ Research Methods:
– Introduction of the InternLM-XComposer2.5-OmniLive (IXC2.5-OL) framework, consisting of three modules: Streaming Perception, Multi-modal Long Memory, and Reasoning.
๐ฌ Research Conclusions:
– The proposed framework simulates human-like cognition, enabling real-time, adaptive interaction with streaming video and audio inputs, enhancing the efficiency and accuracy of long-term interactions.
๐ Paper link: https://huggingface.co/papers/2412.09596
2. Phi-4 Technical Report
๐ Keywords: phi-4, language model, synthetic data, STEM-focused QA, reasoning-focused benchmarks
๐ก Category: Natural Language Processing
๐ Research Objective:
– The primary objective of this research is to develop phi-4, a 14-billion parameter language model, with a focus on enhancing data quality to improve performance on STEM-focused QA tasks.
๐ ๏ธ Research Methods:
– The research employs a novel training approach incorporating synthetic data strategically during the training process, differentiating it from models primarily relying on organic data sources.
๐ฌ Research Conclusions:
– phi-4 exceeds its predecessor, phi-3, and even its teacher model (GPT-4), particularly in reasoning-focused tasks, due to innovative data generation and post-training techniques.
๐ Paper link: https://huggingface.co/papers/2412.08905
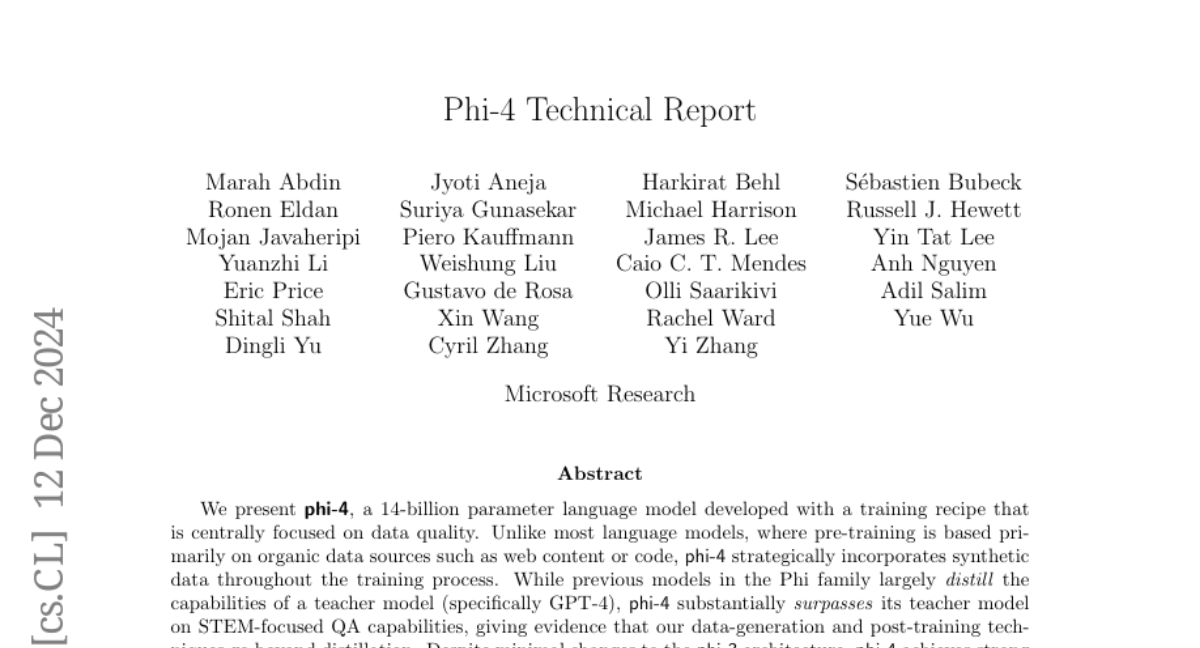
3. Euclid: Supercharging Multimodal LLMs with Synthetic High-Fidelity Visual Descriptions
๐ Keywords: Multimodal large language models, low-level visual perception, Geoperception, Euclid
๐ก Category: Multi-Modal Learning
๐ Research Objective:
– Assess and improve the capability of Multimodal large language models (MLLMs) to accurately perceive and describe geometric details in images.
๐ ๏ธ Research Methods:
– Introduced the Geoperception benchmark for evaluating MLLM geometric transcription abilities.
– Conducted empirical studies using model architectures, training techniques, and data strategies including synthetic data and multi-stage training with a data curriculum.
๐ฌ Research Conclusions:
– Developed Euclid, a model family optimized for geometric perception, demonstrating strong generalization and outperforming existing models like Gemini-1.5-Pro by significant margins on the Geoperception benchmark tasks.
๐ Paper link: https://huggingface.co/papers/2412.08737
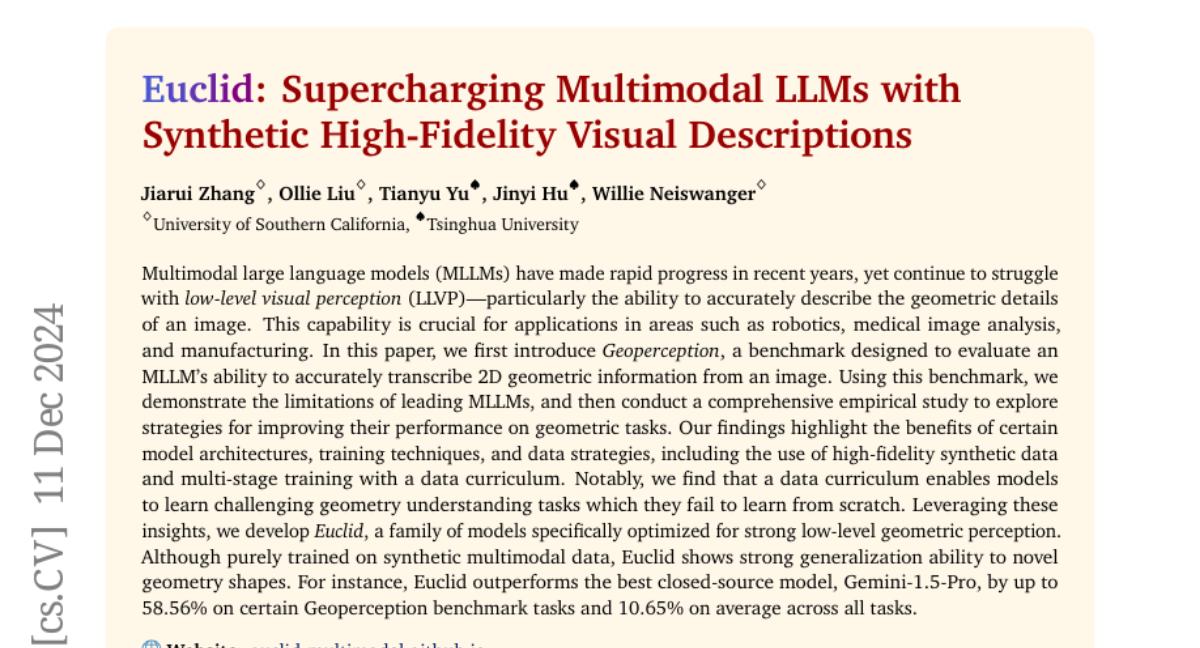
4. Multimodal Latent Language Modeling with Next-Token Diffusion
๐ Keywords: Multimodal generative models, LatentLM, causal Transformers, Variational Autoencoder, scalability
๐ก Category: Multi-Modal Learning
๐ Research Objective:
– The study aims to create a unified approach for handling both discrete and continuous data within multimodal generative models, utilizing Latent Language Modeling (LatentLM).
๐ ๏ธ Research Methods:
– The researchers employ a causal Transformer framework integrating a Variational Autoencoder for continuous data and develop next-token diffusion for autoregressive generation. They also introduce sigma-VAE to solve variance collapse challenges.
๐ฌ Research Conclusions:
– LatentLM demonstrates superior scalability and performance in multimodal tasks. Specifically, it outperforms Diffusion Transformers in image generation and the VALL-E 2 model in text-to-speech synthesis, establishing itself as a potent tool for advancing large multimodal models.
๐ Paper link: https://huggingface.co/papers/2412.08635
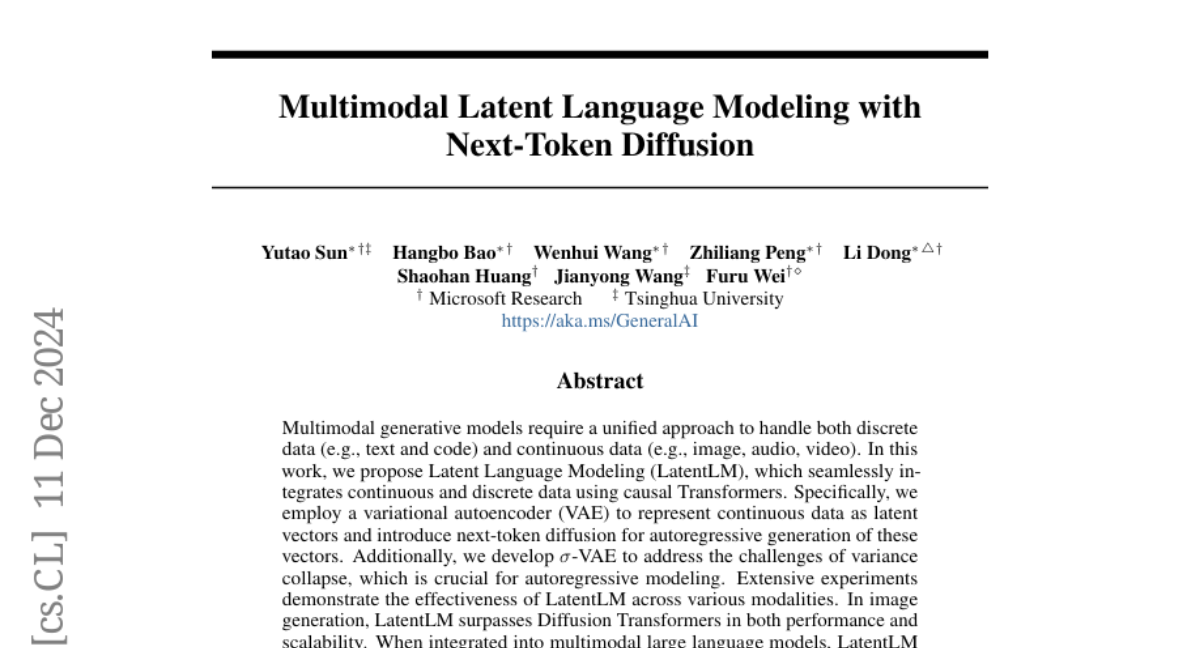
5. EasyRef: Omni-Generalized Group Image Reference for Diffusion Models via Multimodal LLM
๐ Keywords: EasyRef, diffusion models, multi-modal large language model, MRBench, zero-shot generalization
๐ก Category: Generative Models
๐ Research Objective:
– Introduce EasyRef to enable diffusion models to condition on multiple reference images and text prompts.
๐ ๏ธ Research Methods:
– Utilize multi-image comprehension with a multi-modal large language model, incorporating efficient reference aggregation and a progressive training scheme.
๐ฌ Research Conclusions:
– EasyRef outperforms both tuning-free methods and tuning-based methods in aesthetic quality and zero-shot generalization across various domains.
๐ Paper link: https://huggingface.co/papers/2412.09618
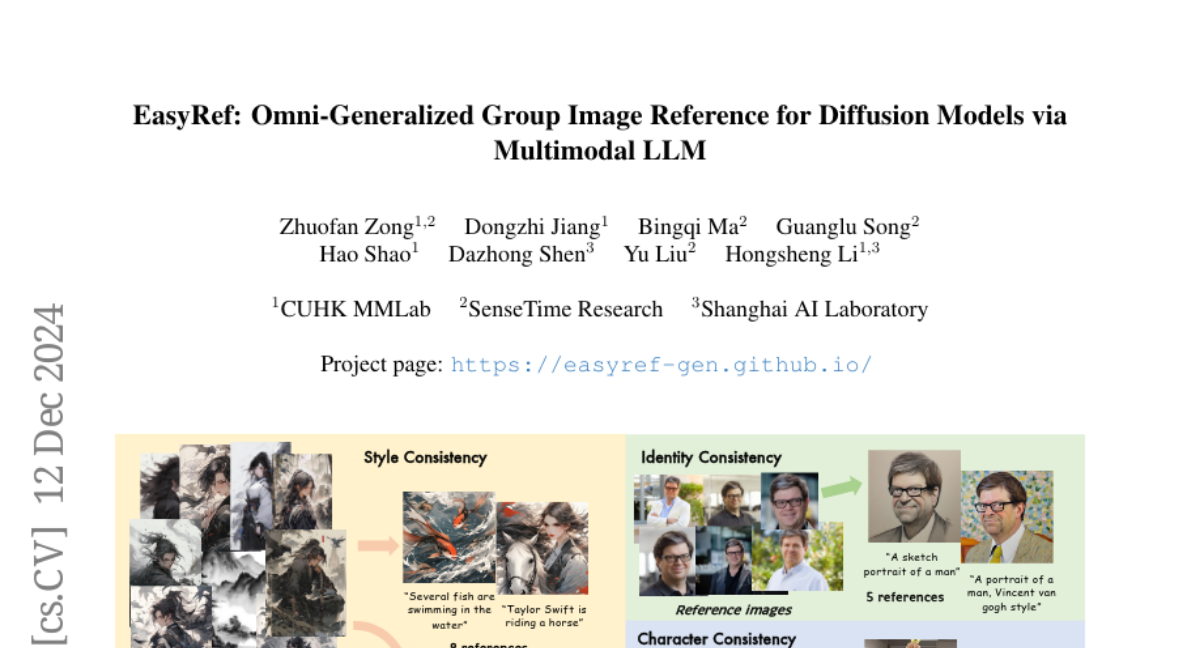
6. AgentTrek: Agent Trajectory Synthesis via Guiding Replay with Web Tutorials
๐ Keywords: GUI agents, data synthesis, visual-language model, automation
๐ก Category: AI Systems and Tools
๐ Research Objective:
– To develop a scalable data synthesis pipeline, AgentTrek, for generating high-quality GUI agent trajectories using web tutorials, aiming to overcome the limitations of current human annotation methods.
๐ ๏ธ Research Methods:
– AgentTrek gathers tutorial-like texts from the internet, transforms them into task goals with step-by-step instructions, and utilizes a visual-language model agent to simulate execution in real digital environments.
– A VLM-based evaluator ensures the accuracy of these generated trajectories.
๐ฌ Research Conclusions:
– Synthesized trajectories enable GUI agents to improve grounding and planning performance more significantly than existing models.
– The proposed approach is more cost-efficient compared to traditional human annotation methods, suggesting the potential for large-scale GUI agent training.
๐ Paper link: https://huggingface.co/papers/2412.09605
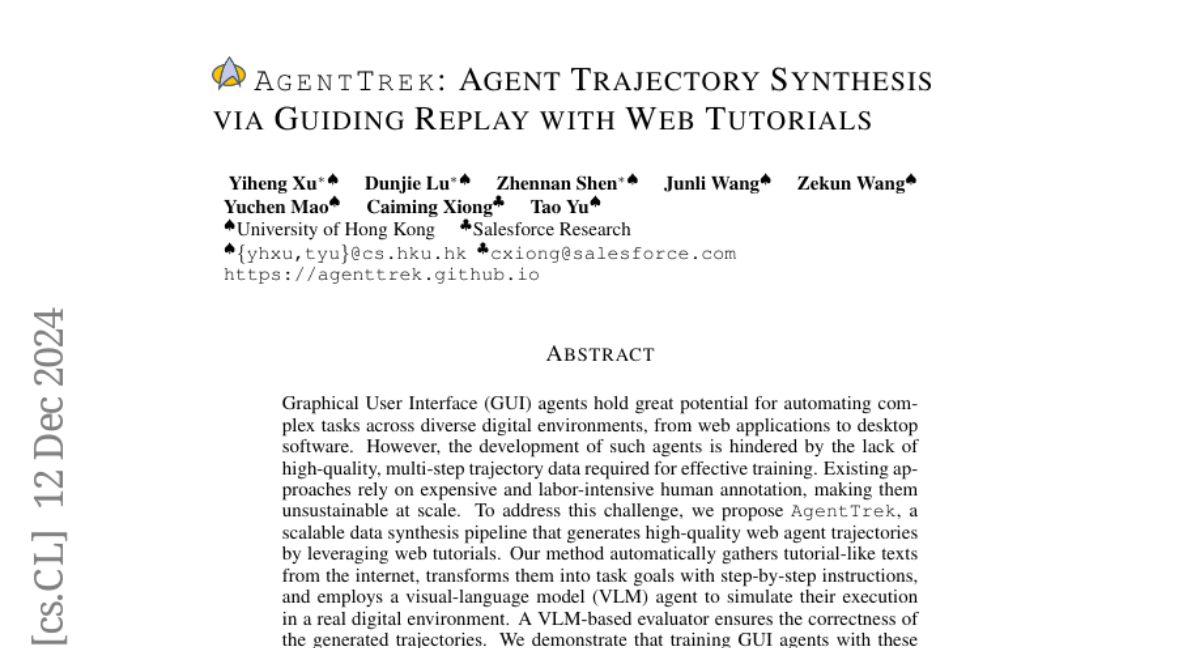
7. SnapGen: Taming High-Resolution Text-to-Image Models for Mobile Devices with Efficient Architectures and Training
๐ Keywords: Text-to-Image (T2I) diffusion models, high-resolution images, mobile platforms, knowledge distillation, SnapGen
๐ก Category: Generative Models
๐ Research Objective:
– To develop a small and fast text-to-image diffusion model capable of generating high-resolution, high-quality images on mobile devices.
๐ ๏ธ Research Methods:
– Exploration of optimal network architecture to minimize model size and latency without compromising on quality.
– Implementation of cross-architecture knowledge distillation using a multi-level approach for model training.
– Integration of adversarial guidance with knowledge distillation to enable few-step image generation.
๐ฌ Research Conclusions:
– The SnapGen model can generate 1024×1024 px images on mobile devices in about 1.4 seconds and outperform larger models on T2I benchmarks, achieving significant size reductions while maintaining high performance.
๐ Paper link: https://huggingface.co/papers/2412.09619
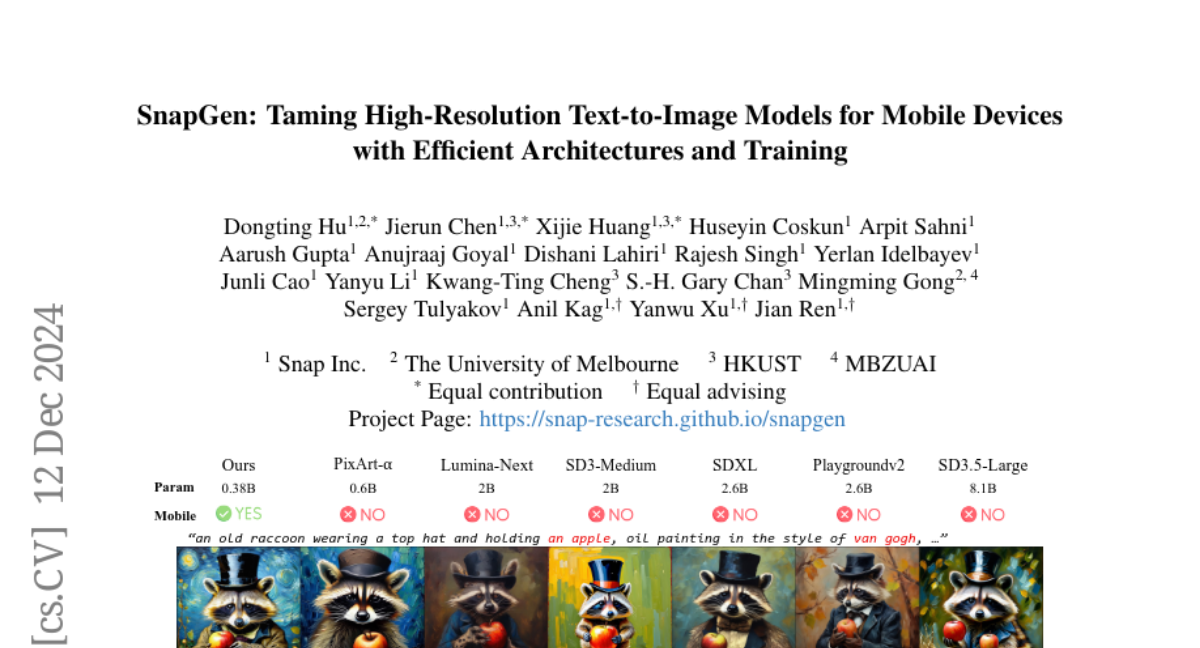
8. Neural LightRig: Unlocking Accurate Object Normal and Material Estimation with Multi-Light Diffusion
๐ Keywords: Neural LightRig, intrinsic estimation, diffusion model, surface normals, PBR material estimation
๐ก Category: Computer Vision
๐ Research Objective:
– To recover the geometry and materials of objects from a single image using the Neural LightRig framework, enhancing intrinsic estimation with multi-lighting conditions.
๐ ๏ธ Research Methods:
– The method involves utilizing illumination priors from large-scale diffusion models to create a synthetic relighting dataset, generating images with varied lighting conditions. A large G-buffer model with a U-Net backbone is trained to predict surface normals and materials accurately.
๐ฌ Research Conclusions:
– The proposed approach outperforms state-of-the-art methods, providing accurate surface normal and PBR material estimation with vivid relighting effects.
๐ Paper link: https://huggingface.co/papers/2412.09593
9. PIG: Physics-Informed Gaussians as Adaptive Parametric Mesh Representations
๐ Keywords: Physics-Informed Neural Networks, Partial Differential Equations, Gaussian Functions
๐ก Category: Machine Learning
๐ Research Objective:
– To overcome the limitations of existing neural network methods in approximating complex Partial Differential Equations.
๐ ๏ธ Research Methods:
– Introducing Physics-Informed Gaussians (PIGs) that combine Gaussian feature embeddings with a lightweight neural network, using trainable parameters for dynamic adaptability.
๐ฌ Research Conclusions:
– The proposed PIGs demonstrate competitive performance across various PDEs, maintaining the benefits of the existing optimization framework, showcasing robustness in solving complex PDEs.
๐ Paper link: https://huggingface.co/papers/2412.05994
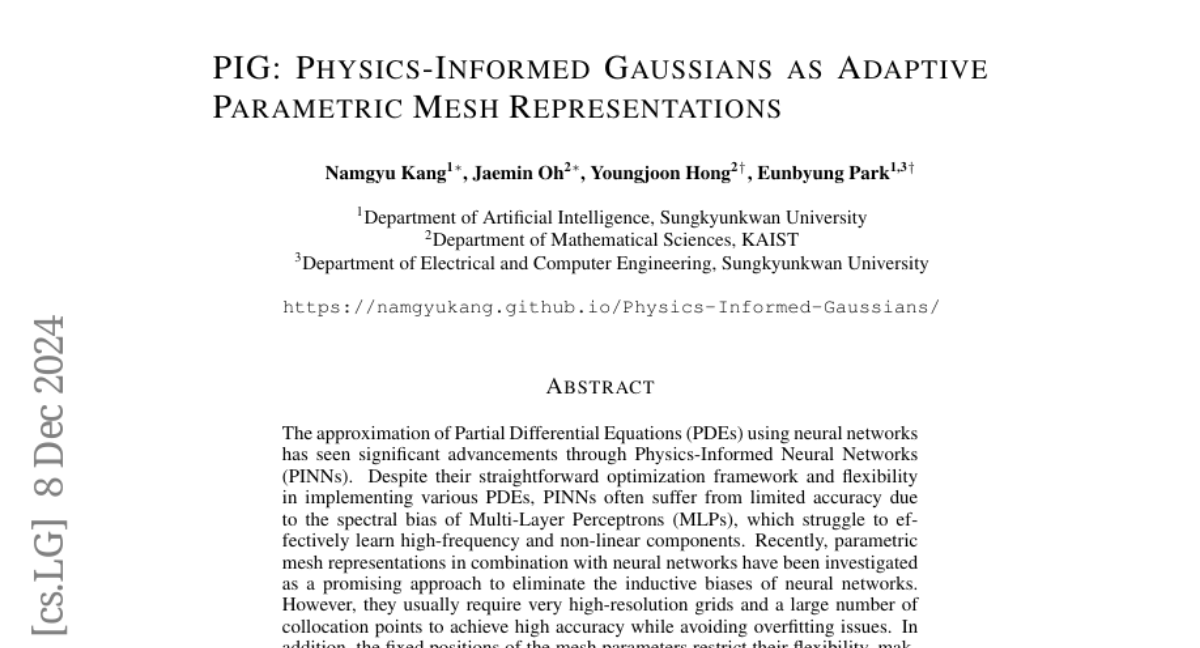
10. JuStRank: Benchmarking LLM Judges for System Ranking
๐ Keywords: generative AI, LLM judge, system ranking, bias, AI Native
๐ก Category: AI Systems and Tools
๐ Research Objective:
– The research aims to systematically evaluate and compare various generative AI models and configurations using LLM-based judges.
๐ ๏ธ Research Methods:
– Conducting a large-scale study where LLM judges rank systems by aggregating judgment scores from multiple outputs and comparing these to human-based rankings.
๐ฌ Research Conclusions:
– The study highlights the decisive and potentially biased behavior of LLM judges, and validates their effectiveness as system rankers compared to human judgment.
๐ Paper link: https://huggingface.co/papers/2412.09569
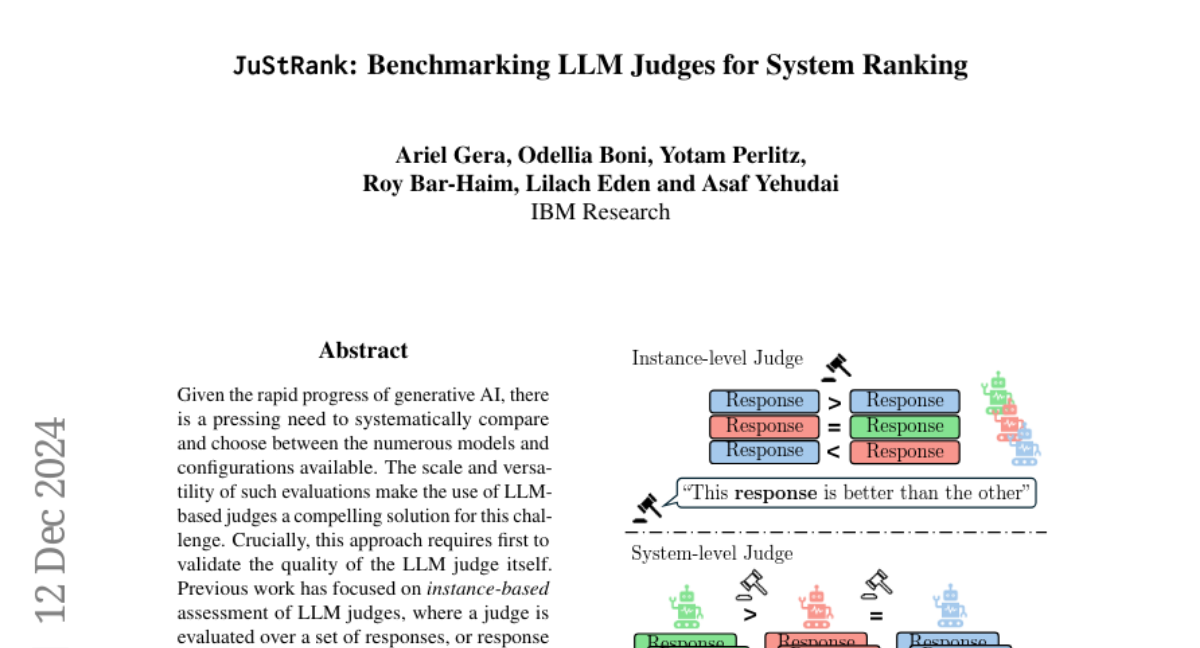
11. Lyra: An Efficient and Speech-Centric Framework for Omni-Cognition
๐ Keywords: Multi-modal Large Language Models, Lyra, speech integration, omni-cognition
๐ก Category: Multi-Modal Learning
๐ Research Objective:
– To develop Lyra, a Multi-modal Large Language Model (MLLM) that enhances multimodal abilities, focusing on integrating speech with other modalities for more versatile AI performance.
๐ ๏ธ Research Methods:
– Lyra uses three strategies: using open-source models with a multi-modality LoRA to reduce costs, employing a latent multi-modality regularizer to link speech with other modalities, and creating an extensive dataset of 1.5M multi-modal samples and 12K long speech samples.
๐ฌ Research Conclusions:
– Lyra demonstrates state-of-the-art performance on various benchmarks in vision-language, vision-speech, and speech-language tasks, achieving efficient omni-cognition while using fewer resources.
๐ Paper link: https://huggingface.co/papers/2412.09501
12. Learned Compression for Compressed Learning
๐ Keywords: Compressed-domain learning, WaLLoC, Neural codec architecture, Linear operations, Efficient computing
๐ก Category: Machine Learning
๐ Research Objective:
– The paper introduces WaLLoC (Wavelet Learned Lossy Compression), a neural codec architecture designed to overcome limitations in existing compressed learning systems by enhancing efficiency and reducing dimensionality without significant information loss.
๐ ๏ธ Research Methods:
– WaLLoC combines linear transform coding with nonlinear dimensionality-reducing autoencoders, incorporating an invertible wavelet packet transform, entropy bottleneck, and shallow asymmetric autoencoder, largely based on linear operations for high efficiency.
๐ฌ Research Conclusions:
– WaLLoC outperforms current state-of-the-art autoencoders in latent diffusion models by providing efficient representations across different modalities, making it ideal for applications like mobile computing and data learning while supporting tasks such as image classification and music source separation.
๐ Paper link: https://huggingface.co/papers/2412.09405
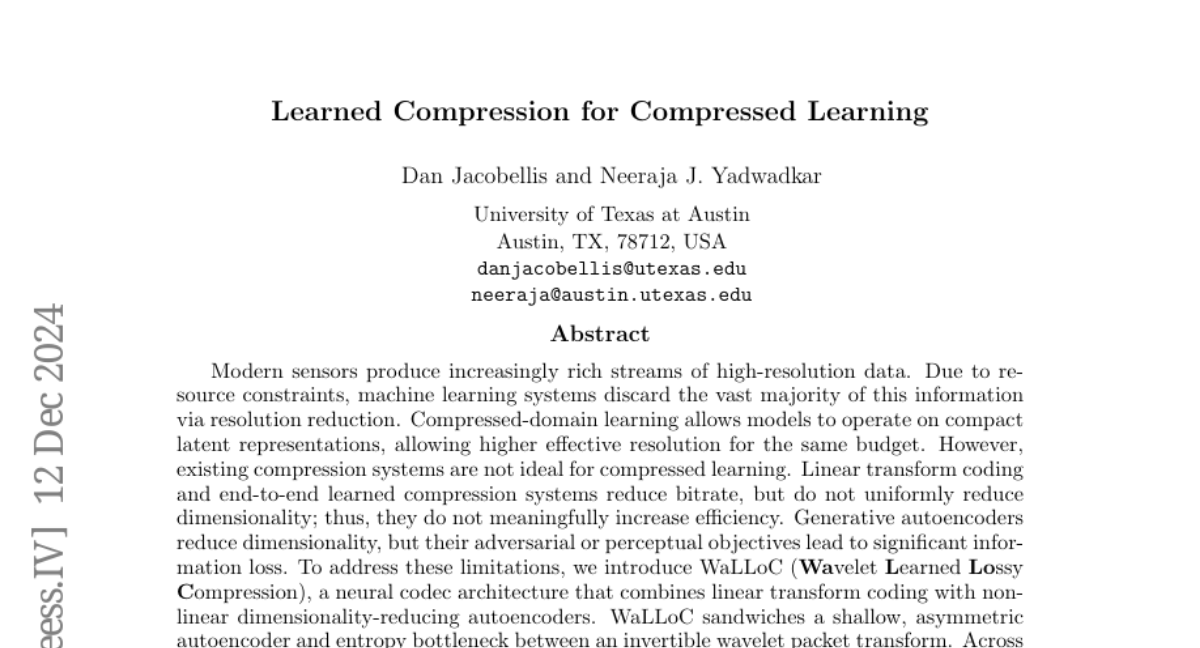
13. RuleArena: A Benchmark for Rule-Guided Reasoning with LLMs in Real-World Scenarios
๐ Keywords: RuleArena, LLMs, real-world applications, logical reasoning
๐ก Category: Knowledge Representation and Reasoning
๐ Research Objective:
– The objective is to evaluate the proficiency of large language models (LLMs) in adhering to complex real-world rules requiring logical reasoning, long-context understanding, and accurate mathematical computation.
๐ ๏ธ Research Methods:
– RuleArena, a novel benchmark, is employed to assess LLMs, focusing on three practical domains: airline baggage fees, NBA transactions, and tax regulations.
๐ฌ Research Conclusions:
– Results indicate significant challenges for LLMs, such as difficulty in rule identification, application, and performing accurate mathematical computations, revealing their limitations in real-life applications.
๐ Paper link: https://huggingface.co/papers/2412.08972
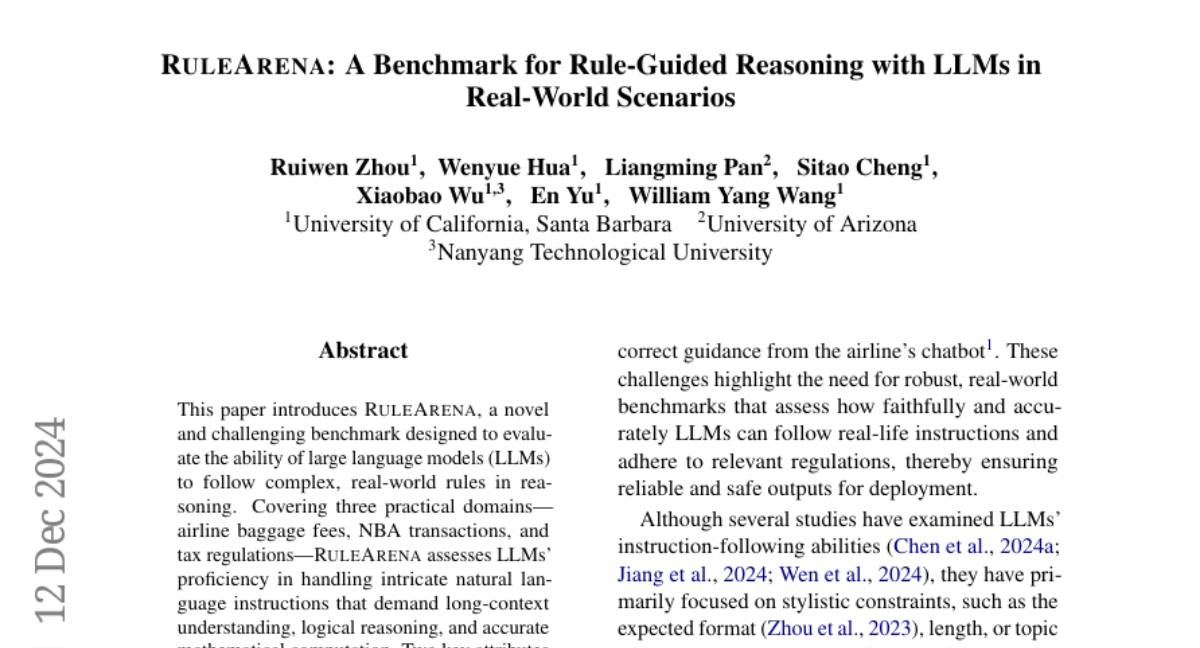
14. OLA-VLM: Elevating Visual Perception in Multimodal LLMs with Auxiliary Embedding Distillation
๐ Keywords: MLLM, OLA-VLM, visual representations, embedding optimization, natural language supervision
๐ก Category: Multi-Modal Learning
๐ Research Objective:
– To optimize intermediate LLM representations through a vision-oriented approach to enhance visual understanding.
๐ ๏ธ Research Methods:
– Introduced OLA-VLM, an approach distilling knowledge into LLM’s hidden representations using visual data during pretraining, focusing on visual embedding and text-token prediction.
๐ฌ Research Conclusions:
– OLA-VLM demonstrated improved quality of representations and outperformed existing models, boosting performance by up to 8.7% on the Depth task in CV-Bench.
๐ Paper link: https://huggingface.co/papers/2412.09585
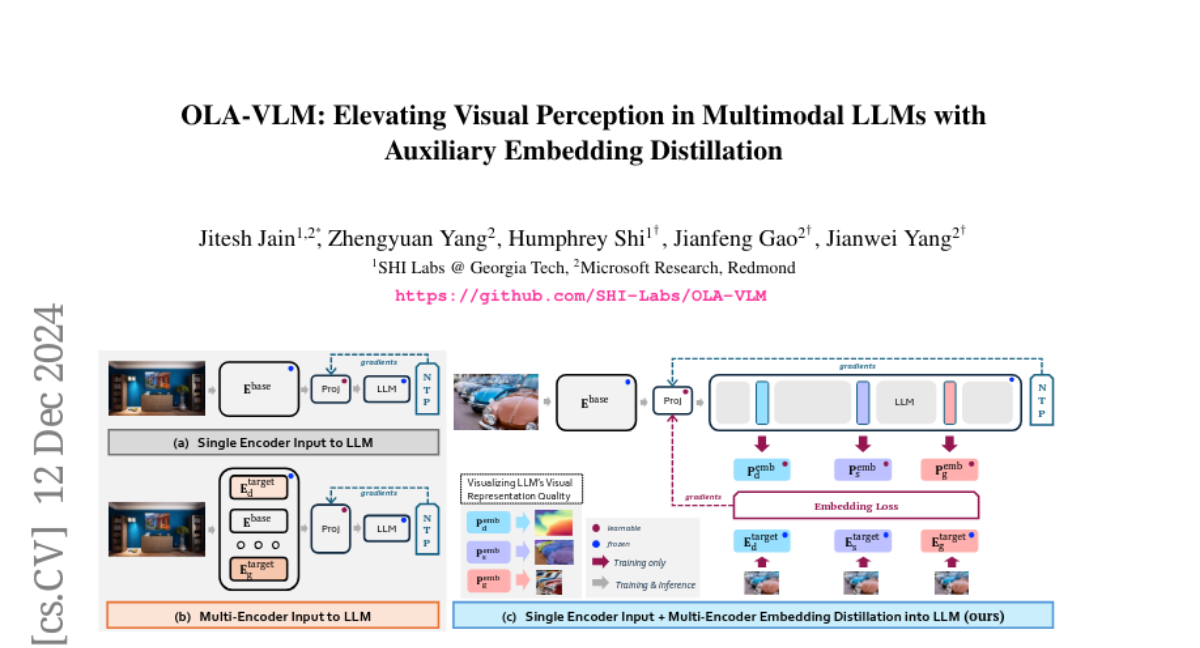
15. Gaze-LLE: Gaze Target Estimation via Large-Scale Learned Encoders
๐ Keywords: Gaze Target Estimation, Transformer Framework, DINOv2 Encoder
๐ก Category: Computer Vision
๐ Research Objective:
– To predict where a person is looking in a scene using gaze target estimation.
๐ ๏ธ Research Methods:
– Introduced Gaze-LLE, a transformer framework that simplifies the gaze estimation process by utilizing features from a frozen DINOv2 encoder.
๐ฌ Research Conclusions:
– Achieved state-of-the-art performance on several gaze benchmarks, validating the effectiveness of their design approach.
๐ Paper link: https://huggingface.co/papers/2412.09586
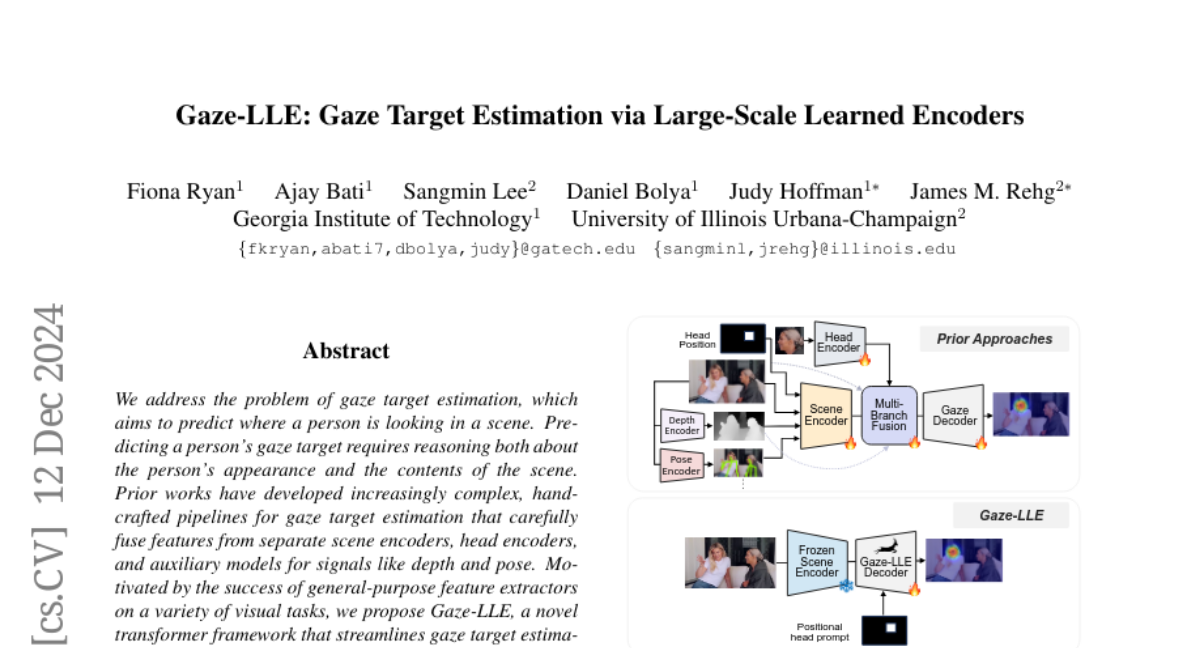
16. DisPose: Disentangling Pose Guidance for Controllable Human Image Animation
๐ Keywords: Controllable human image animation, sparse guidance, DisPose, dense motion field, plug-and-play hybrid ControlNet
๐ก Category: Computer Vision
๐ Research Objective:
– To improve controllable human image animation by generating videos from reference images with a focus on more generalizable control signals without dense input requirements.
๐ ๏ธ Research Methods:
– Introduction of DisPose, which uses sparse skeleton pose to create a dense motion field and keypoint correspondence, along with a plug-and-play hybrid ControlNet for improved video quality and consistency.
๐ฌ Research Conclusions:
– DisPose demonstrates superior performance in both qualitative and quantitative evaluations, providing better video alignment and identity preservation compared to existing methods.
๐ Paper link: https://huggingface.co/papers/2412.09349
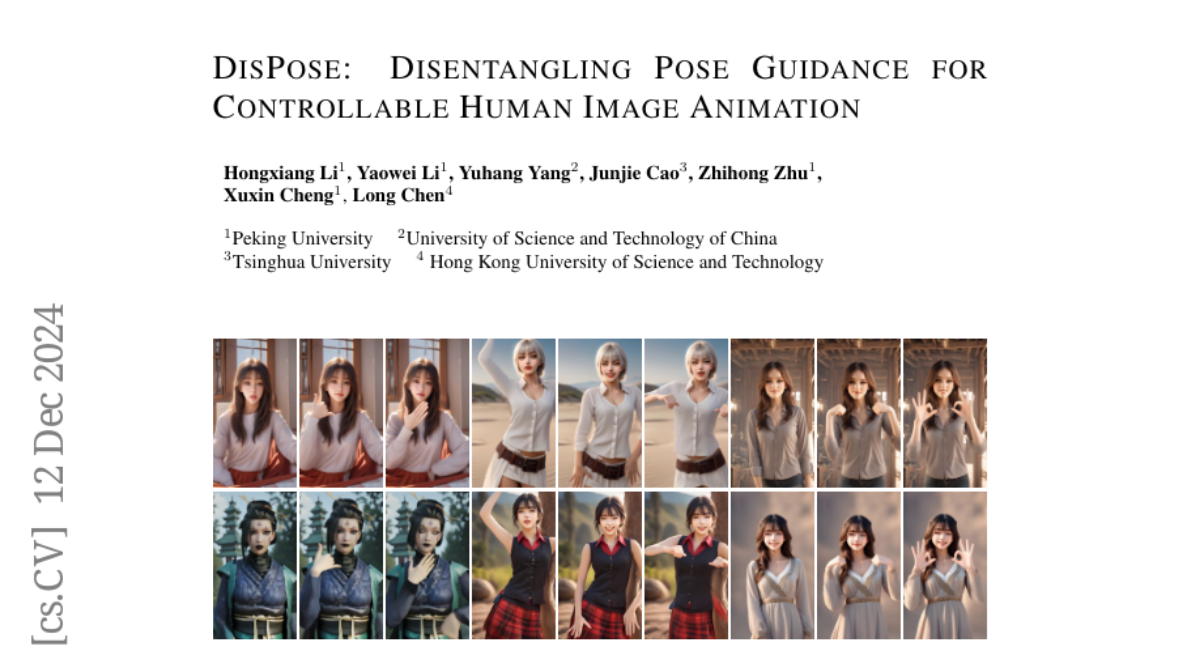
17. Word Sense Linking: Disambiguating Outside the Sandbox
๐ Keywords: Word Sense Disambiguation (WSD), Word Sense Linking (WSL), Transformer-based architecture
๐ก Category: Natural Language Processing
๐ Research Objective:
– The study aims to introduce a new task called Word Sense Linking (WSL) which extends Word Sense Disambiguation (WSD) by requiring systems to identify and link spans in text to their correct meanings without prior assumptions.
๐ ๏ธ Research Methods:
– A transformer-based architecture is developed and evaluated against state-of-the-art WSD systems, gradually relaxing the conventional WSD assumptions.
๐ฌ Research Conclusions:
– The paper proposes that WSL can further integrate lexical semantics into downstream applications more effectively than traditional WSD, addressing key challenges in application contexts.
๐ Paper link: https://huggingface.co/papers/2412.09370
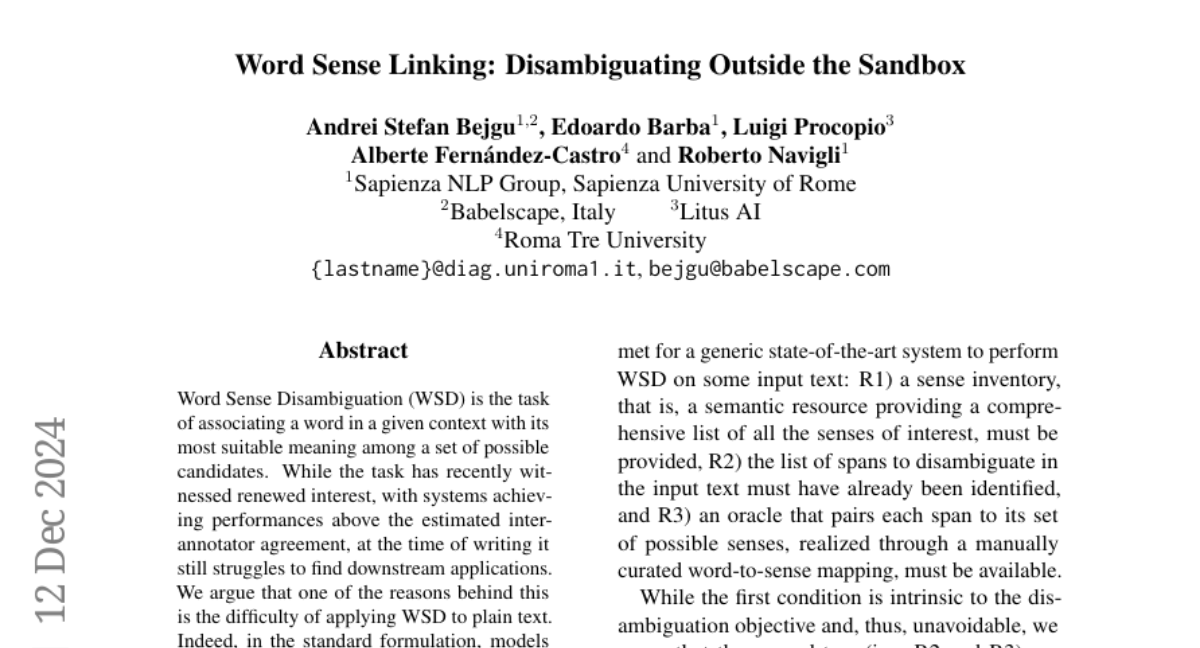
18. LoRACLR: Contrastive Adaptation for Customization of Diffusion Models
๐ Keywords: Text-to-Image Customization, Personalized Images, Multi-Concept Image Generation, LoRACLR
๐ก Category: Generative Models
๐ Research Objective:
– Introduce LoRACLR for combining multiple personalized models into a unified model for multi-concept image generation.
๐ ๏ธ Research Methods:
– Use a contrastive objective to align and merge the weight spaces of LoRA models, ensuring distinct and cohesive representations for each concept.
๐ฌ Research Conclusions:
– LoRACLR effectively merges multiple concepts, enhancing the capabilities of personalized image generation with high-quality synthesis.
๐ Paper link: https://huggingface.co/papers/2412.09622
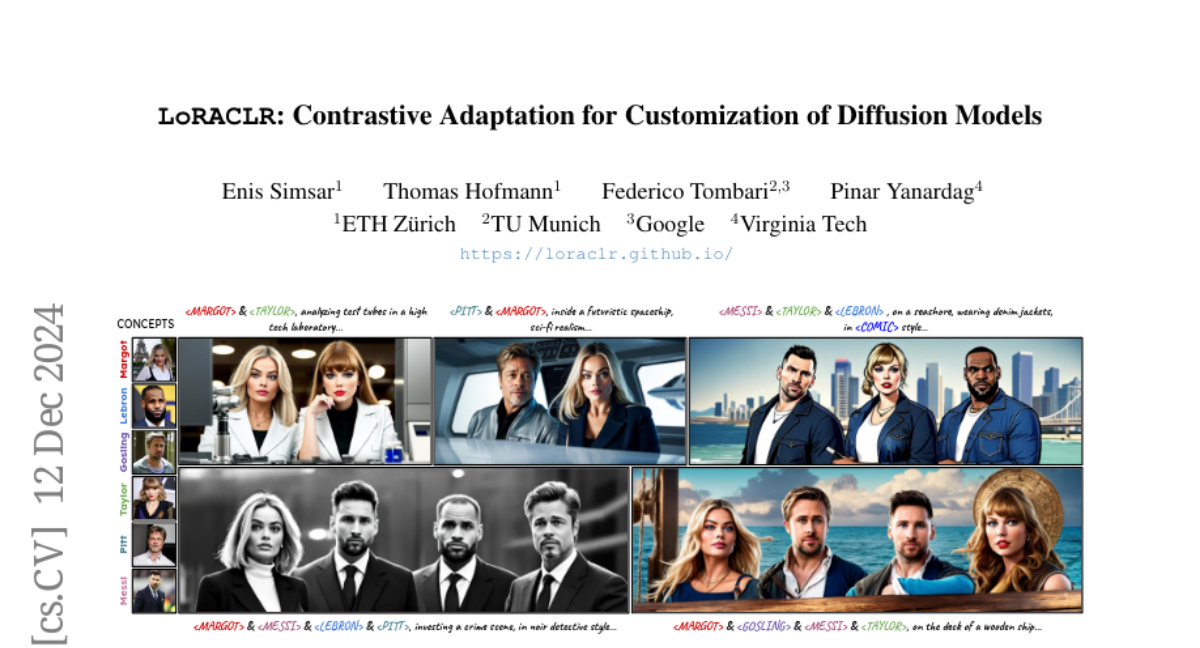
19. FreeSplatter: Pose-free Gaussian Splatting for Sparse-view 3D Reconstruction
๐ Keywords: Sparse-view reconstruction, FreeSplatter, transformer architecture, 3D Gaussians, camera parameters
๐ก Category: Computer Vision
๐ Research Objective:
– Develop a scalable reconstruction framework (FreeSplatter) for high-quality 3D modeling from uncalibrated sparse-view images.
๐ ๏ธ Research Methods:
– Utilize a streamlined transformer architecture with sequential self-attention to process multi-view image tokens into 3D Gaussian primitives and estimate camera parameters.
๐ฌ Research Conclusions:
– FreeSplatter surpasses state-of-the-art baselines in reconstruction quality and pose estimation accuracy and enhances applications, including text/image-to-3D content creation.
๐ Paper link: https://huggingface.co/papers/2412.09573
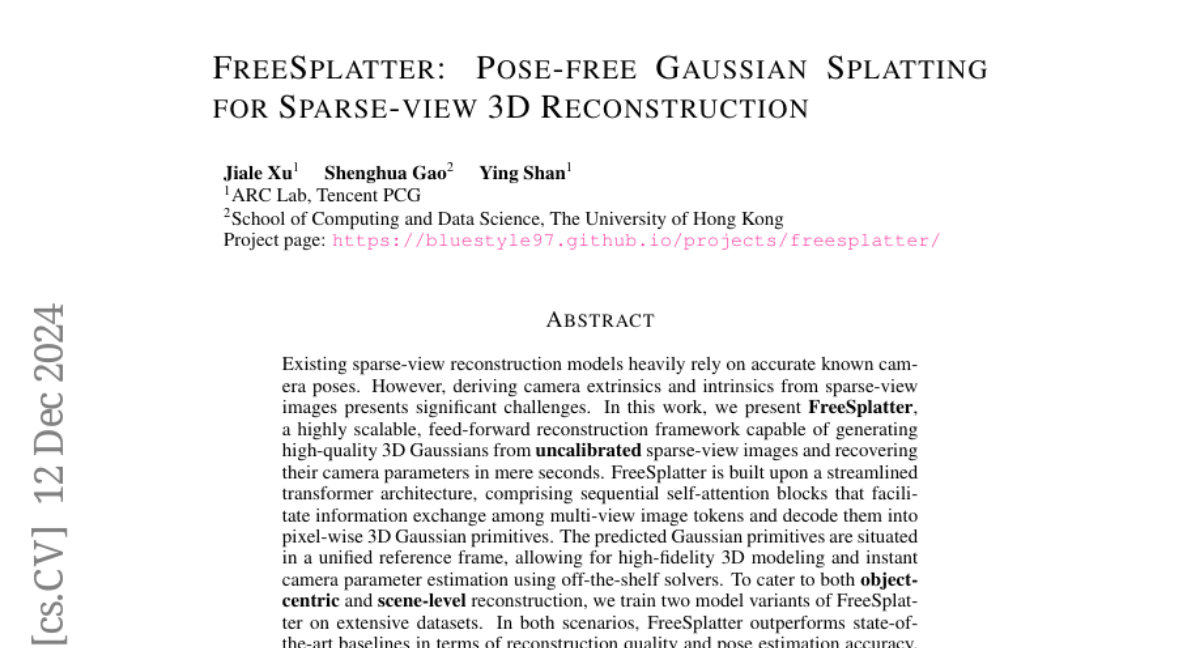
20. Arbitrary-steps Image Super-resolution via Diffusion Inversion
๐ Keywords: Image Super-Resolution, Diffusion Inversion, Noise Prediction, Sampling Mechanism
๐ก Category: Computer Vision
๐ Research Objective:
– Introduce a new image super-resolution technique utilizing diffusion inversion to leverage rich image priors from large pre-trained diffusion models for improved performance.
๐ ๏ธ Research Methods:
– Design of a Partial Noise Prediction strategy to establish an intermediate state of the diffusion model for the sampling process.
– Deployment of a deep noise predictor to estimate optimal noise maps for the forward diffusion process, facilitating flexible and efficient sampling.
๐ฌ Research Conclusions:
– The proposed method demonstrates superior or comparable performance to recent state-of-the-art approaches, even with a minimal number of sampling steps, offering increased flexibility without compromising efficiency.
๐ Paper link: https://huggingface.co/papers/2412.09013
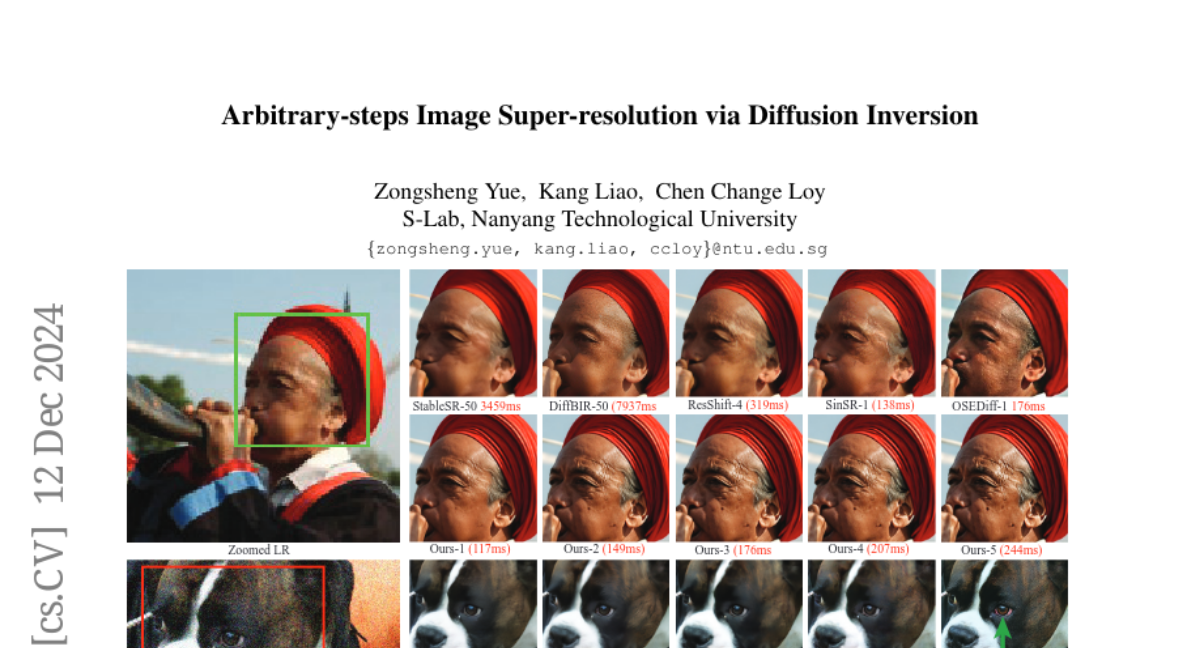
21. The Impact of Copyrighted Material on Large Language Models: A Norwegian Perspective
๐ Keywords: Copyrighted Materials, Generative Language Models, Large Language Models, AI Ethics
๐ก Category: AI Ethics and Fairness
๐ Research Objective:
– To empirically assess the impact of copyrighted materials on the performance of large language models (LLMs) for the Norwegian language.
๐ ๏ธ Research Methods:
– Evaluating LLM performance using a diverse set of Norwegian benchmarks to measure contributions from different types of copyrighted texts.
๐ฌ Research Conclusions:
– Both books and newspapers enhance LLM performance, whereas fiction may reduce it, suggesting the need for a compensation scheme for authors.
๐ Paper link: https://huggingface.co/papers/2412.09460
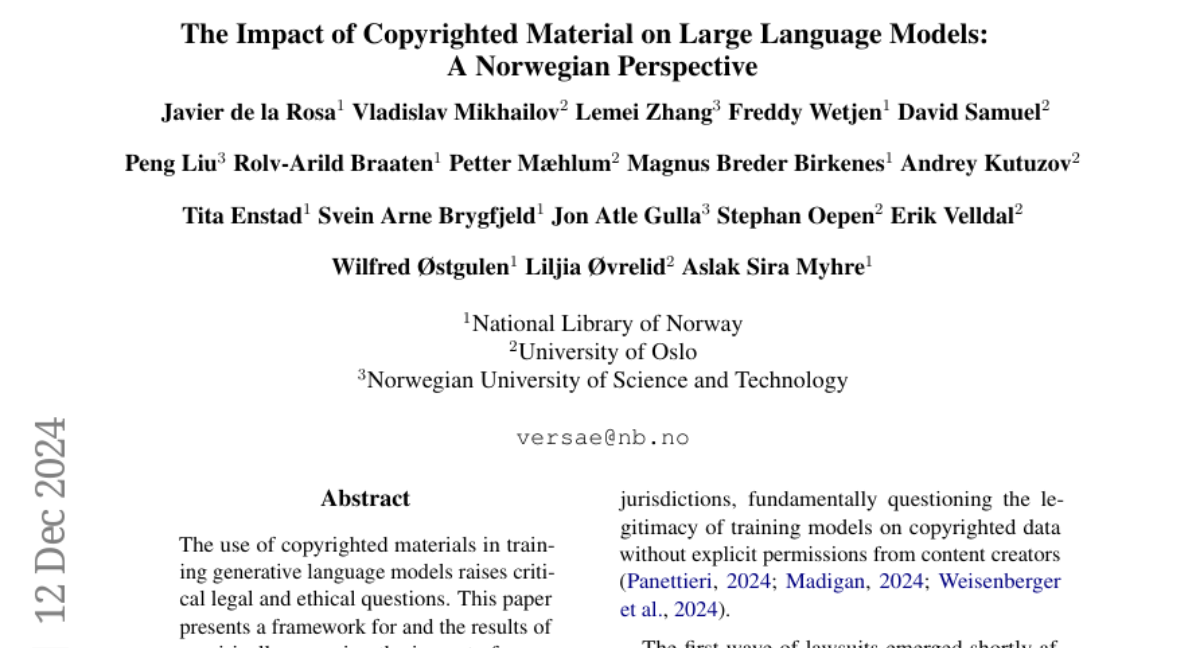
22. VisionArena: 230K Real World User-VLM Conversations with Preference Labels
๐ Keywords: Vision-Language Models, VisionArena, User-VLM Interactions, Multilingual Dataset, Benchmarking
๐ก Category: Human-AI Interaction
๐ Research Objective:
– The primary aim is to create a benchmark capturing authentic user-vision-language model interactions.
๐ ๏ธ Research Methods:
– Developed VisionArena, a dataset with 230K real-world conversations, including subsets for chat, comparison, and automatic benchmarking.
– Analysis of user questions and response styles.
๐ฌ Research Conclusions:
– Open-ended tasks like captioning and humor are highly style-dependent; current models struggle with spatial reasoning.
– Finetuning on VisionArena-Chat outperforms existing models with significant improvements in benchmark scores.
๐ Paper link: https://huggingface.co/papers/2412.08687
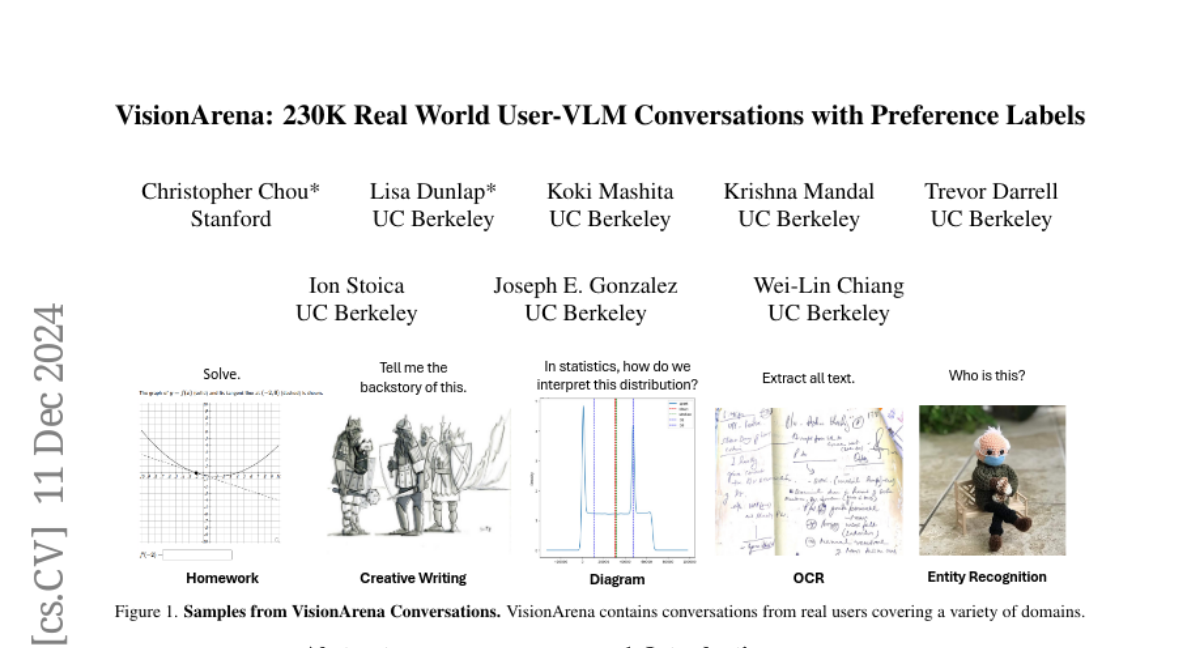
23. Normalizing Flows are Capable Generative Models
๐ Keywords: Normalizing Flows, TarFlow, Transformer-based, Generative Modeling, Diffusion Models
๐ก Category: Generative Models
๐ Research Objective:
– To demonstrate the capabilities and power of Normalizing Flows (NFs) with a new architecture called TarFlow.
๐ ๏ธ Research Methods:
– Development of TarFlow, a simple and scalable Transformer-based architecture integrating autoregressive flows for image patches, with techniques like Gaussian noise augmentation, post training denoising, and effective guidance methods.
๐ฌ Research Conclusions:
– TarFlow achieves state-of-the-art results in likelihood estimation for images, surpassing previous best methods and producing sample quality and diversity comparable to diffusion models as a stand-alone NF model.
๐ Paper link: https://huggingface.co/papers/2412.06329
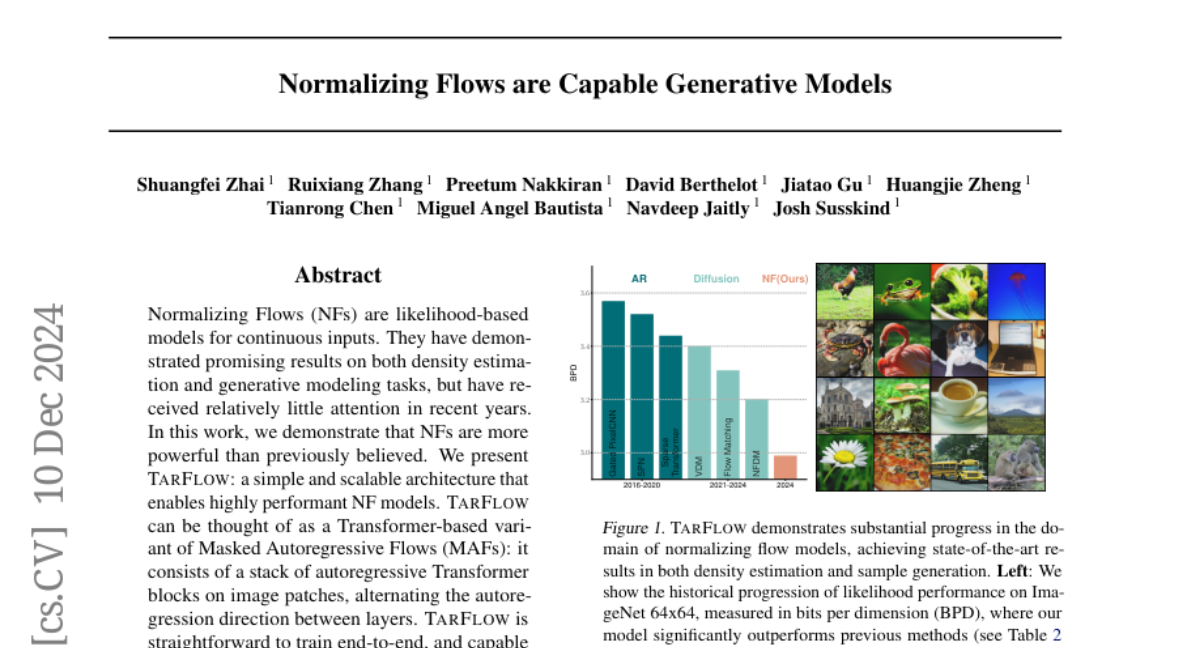
24. SAME: Learning Generic Language-Guided Visual Navigation with State-Adaptive Mixture of Experts
๐ Keywords: Visual Navigation, Language-Guided Navigation, State-Adaptive Mixture of Experts, Instruction-Guided
๐ก Category: Robotics and Autonomous Systems
๐ Research Objective:
– The paper aims to unify diverse visual navigation tasks by proposing a framework that consolidates high-level category-specific search and low-level language-guided navigation, understanding both instructions and environments.
๐ ๏ธ Research Methods:
– Introducing a novel State-Adaptive Mixture of Experts (SAME) model to manage decision-making processes based on varying language granularity and dynamic observations.
๐ฌ Research Conclusions:
– The SAME-enabled agent demonstrates versatility by handling seven navigation tasks simultaneously, with performance surpassing or comparable to task-specific agents.
๐ Paper link: https://huggingface.co/papers/2412.05552
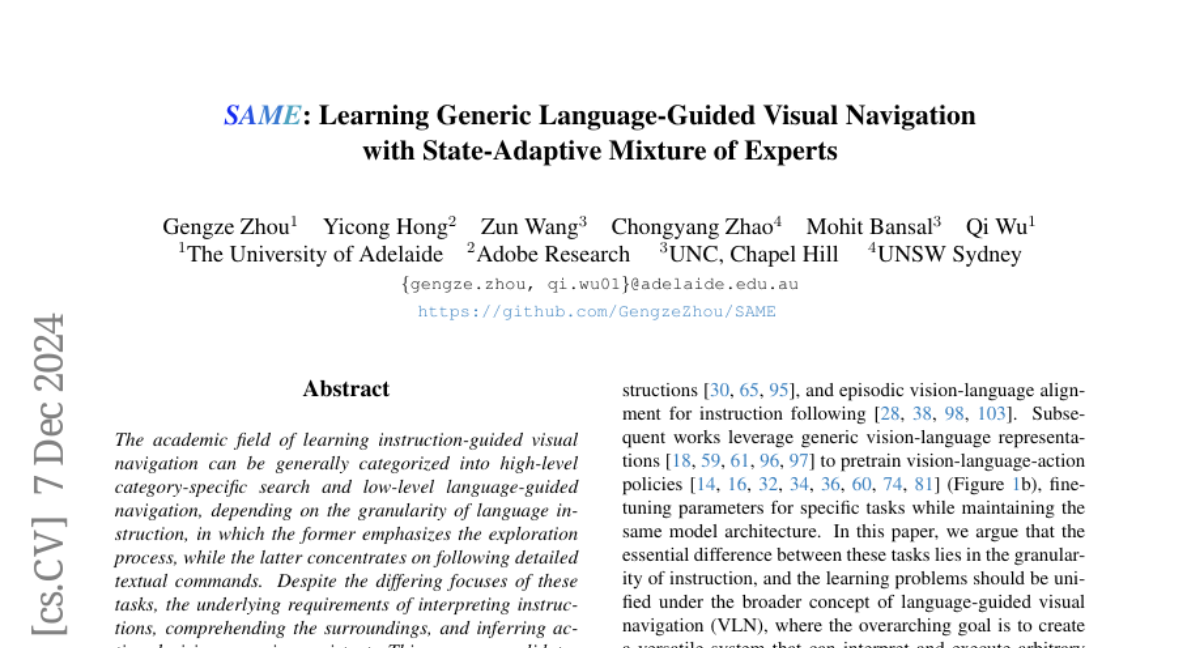
25. Shiksha: A Technical Domain focused Translation Dataset and Model for Indian Languages
๐ Keywords: Neural Machine Translation, low-resource languages, multilingual corpus
๐ก Category: Natural Language Processing
๐ Research Objective:
– To address the challenge of translating scientific and technical content in low-resource Indian languages.
๐ ๏ธ Research Methods:
– Created a multilingual parallel corpus by mining human-translated transcriptions of NPTEL video lectures, containing over 2.8 million translation pairs.
๐ฌ Research Conclusions:
– The finetuned NMT models surpassed other available models in domain-specific tasks and improved out-of-domain translations, achieving over 2 BLEU scores higher on average for Indian languages.
๐ Paper link: https://huggingface.co/papers/2412.09025
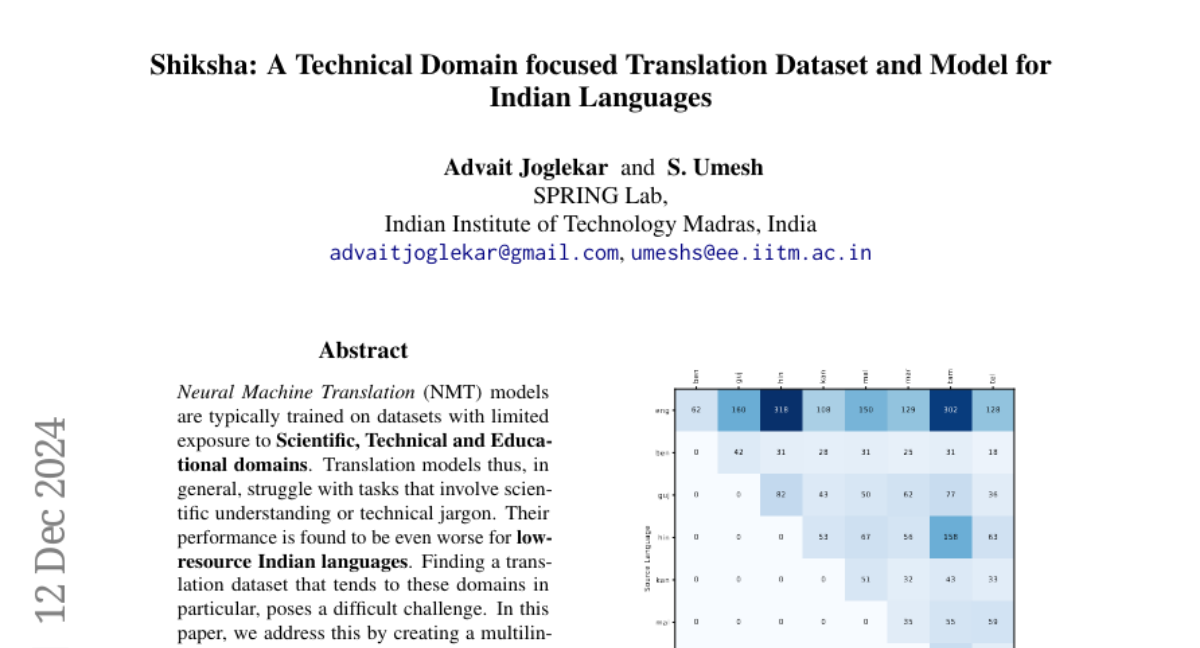
26. ONEBench to Test Them All: Sample-Level Benchmarking Over Open-Ended Capabilities
๐ Keywords: ONEBench, foundation models, open-ended evaluation, heterogeneity, model ranking
๐ก Category: Foundations of AI
๐ Research Objective:
– To introduce ONEBench, a new testing paradigm for evaluating the open-ended capabilities of foundation models through a unified and ever-expanding sample pool.
๐ ๏ธ Research Methods:
– Proposal and implementation of an aggregation algorithm to combine sparse measurements for reliable model scores, ensuring identifiability and rapid convergence.
๐ฌ Research Conclusions:
– The proposed method demonstrates robustness with significant missing data, reducing evaluation costs substantially while maintaining consistent model rankings. Introduction of ONEBench-LLM for language models and ONEBench-LMM for vision-language models, providing a comprehensive cross-domain evaluation framework.
๐ Paper link: https://huggingface.co/papers/2412.06745
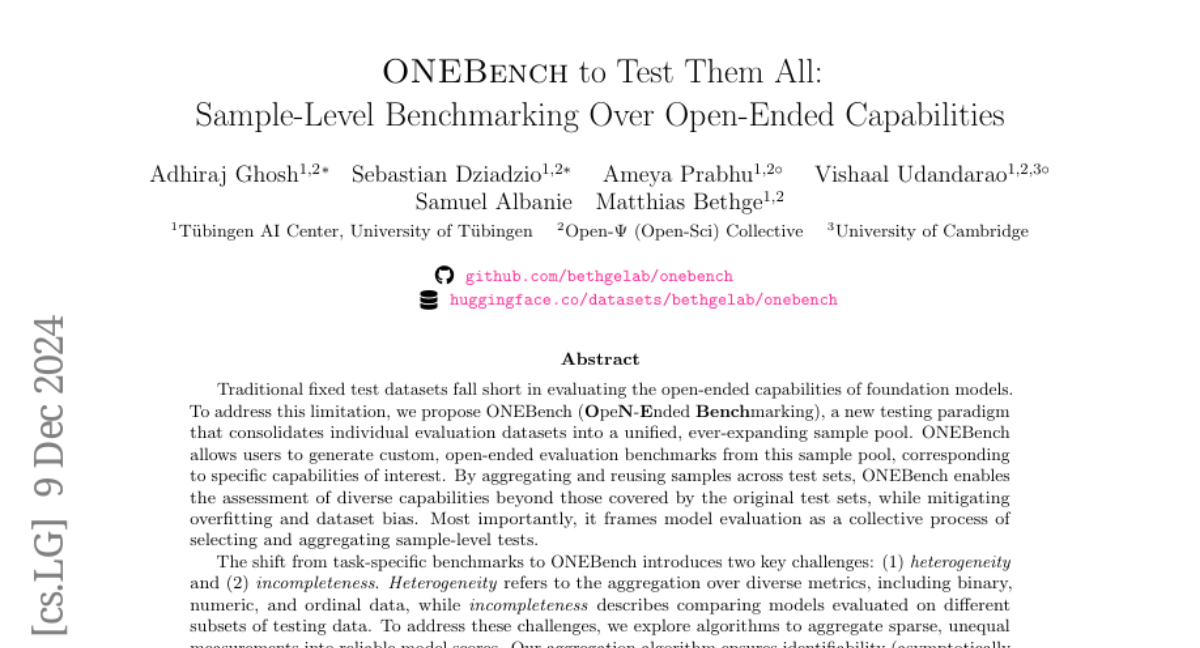