AI Native Daily Paper Digest – 20241223
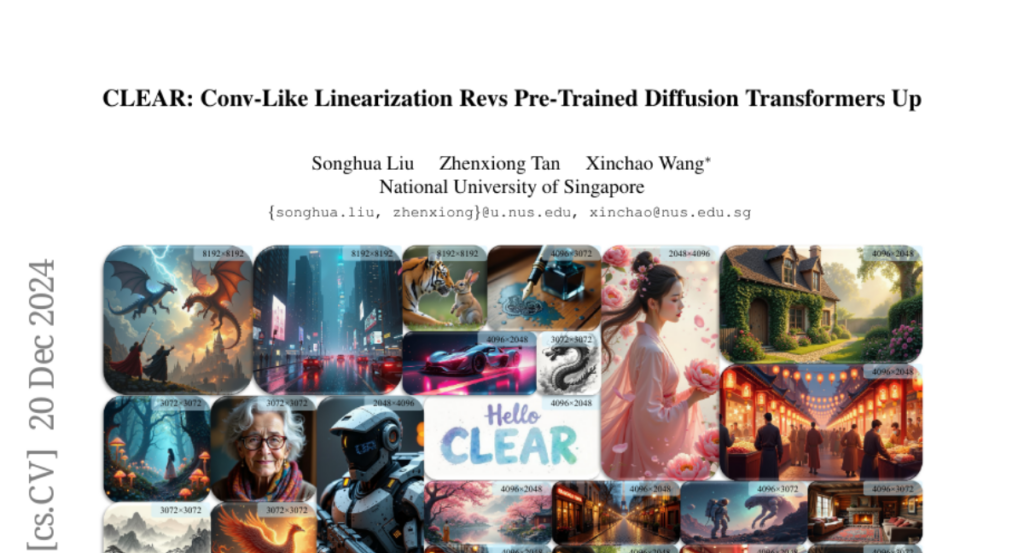
1. Parallelized Autoregressive Visual Generation
๐ Keywords: Autoregressive models, Parallel generation, Visual generation, Inference speed
๐ก Category: Generative Models
๐ Research Objective:
– To enhance the efficiency of visual generation using autoregressive models by introducing a method for parallelized token generation.
๐ ๏ธ Research Methods:
– Developed a parallel generation strategy that generates tokens with weak dependencies in parallel while maintaining sequential generation for strongly dependent tokens, integrated without altering existing model architectures.
๐ฌ Research Conclusions:
– The method achieves a significant speedup in the generation process (up to 9.5x) with minimal impact on quality, as demonstrated in experiments on both image and video datasets like ImageNet and UCF-101.
๐ Paper link: https://huggingface.co/papers/2412.15119
2. Offline Reinforcement Learning for LLM Multi-Step Reasoning
๐ Keywords: Offline Reinforcement Learning, Multi-Step Reasoning, Direct Preference Optimization, Value Function, Multi-Iteration Framework
๐ก Category: Reinforcement Learning
๐ Research Objective:
– To enhance the multi-step reasoning capability of large language models (LLMs) using offline reinforcement learning.
๐ ๏ธ Research Methods:
– Developed OREO (Offline Reasoning Optimization), leveraging the soft Bellman Equation to jointly learn a policy model and value function.
– Compared OREO to existing methods on benchmarks such as GSM8K, MATH, and ALFWorld.
๐ฌ Research Conclusions:
– OREO reduces reliance on pairwise preference data and enhances credit assignment.
– Demonstrated superior performance over existing offline learning methods for multi-step reasoning tasks.
๐ Paper link: https://huggingface.co/papers/2412.16145
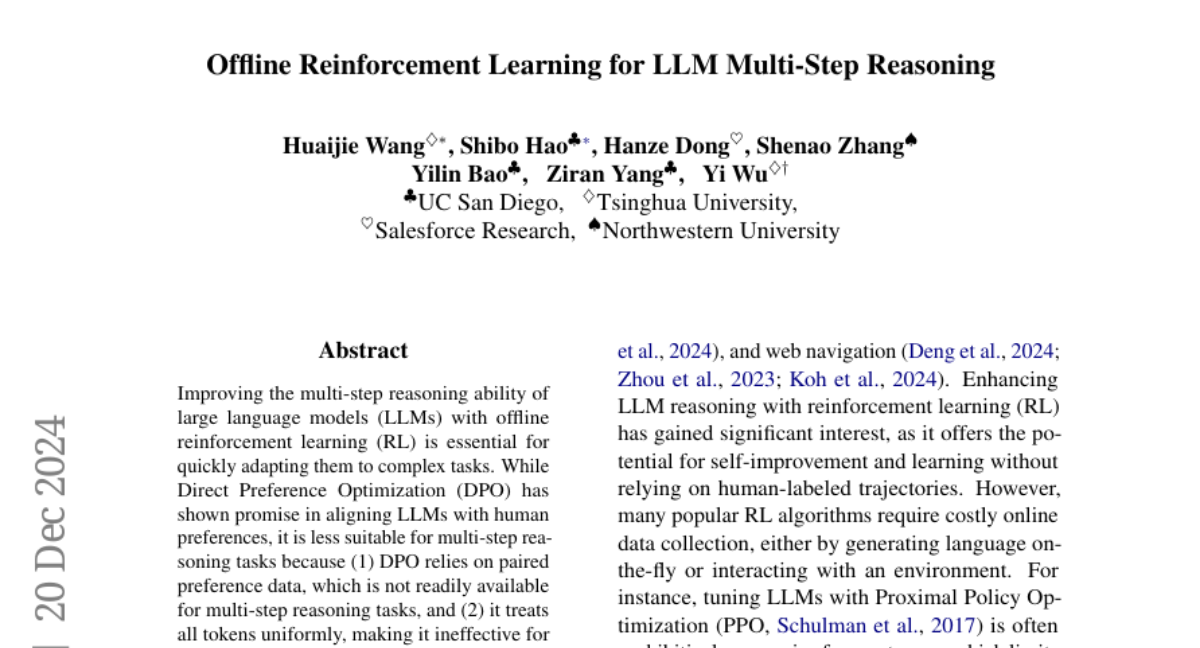
3. SCOPE: Optimizing Key-Value Cache Compression in Long-context Generation
๐ Keywords: KV cache, LLMs, long-context generation, SCOPE, decoding phase
๐ก Category: Generative Models
๐ Research Objective:
– Address bottlenecks in KV cache optimization during the decoding phase for long-context generation in LLMs.
๐ ๏ธ Research Methods:
– Proposed a framework called SCOPE with novel strategies for preserving essential information and optimizing memory usage.
๐ฌ Research Conclusions:
– SCOPE demonstrates effectiveness and generalization in experiments on LongGenBench, showing its compatibility as a plug-in for other KV compression methods.
๐ Paper link: https://huggingface.co/papers/2412.13649
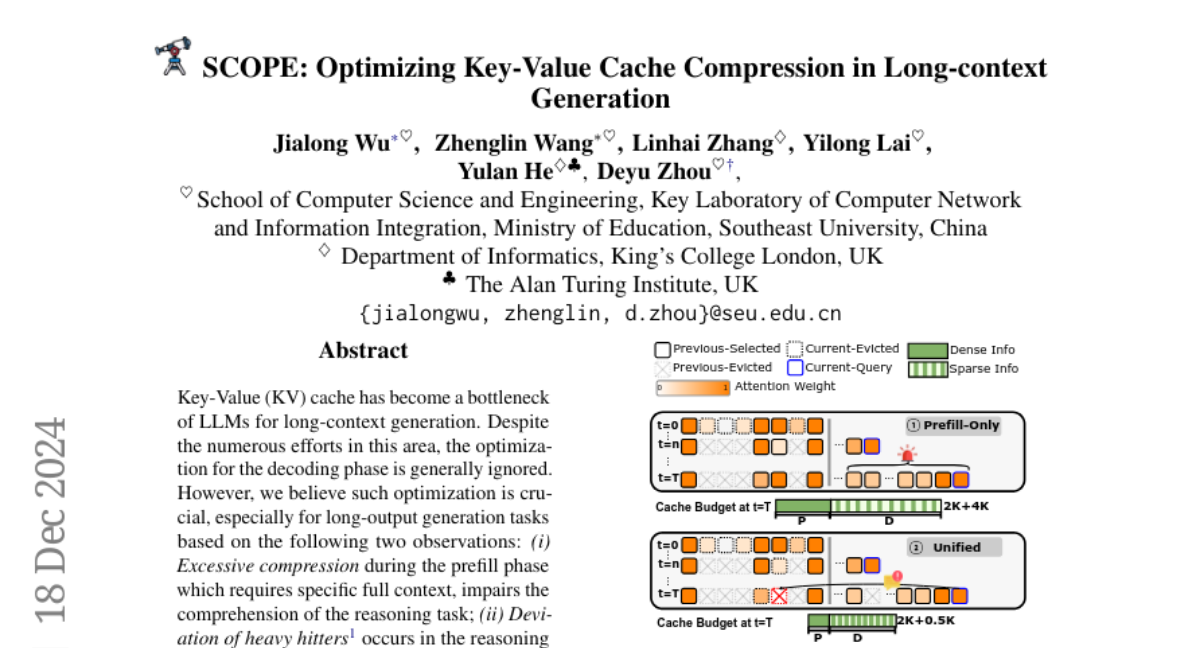
4. Taming Multimodal Joint Training for High-Quality Video-to-Audio Synthesis
๐ Keywords: Multimodal Training, Audio-Visual Synchronization, State-of-the-Art, Semantic Alignment
๐ก Category: Multi-Modal Learning
๐ Research Objective:
– Propose a novel multimodal joint training framework, MMAudio, to synthesize high-quality synchronized audio from video and optional text inputs.
๐ ๏ธ Research Methods:
– Jointly train MMAudio using large-scale text-audio data with a conditional synchronization module for frame-level alignment.
– Optimize with a flow matching objective to achieve superior video-to-audio performance.
๐ฌ Research Conclusions:
– MMAudio achieves state-of-the-art performance in generating audio quality, semantic alignment, and synchronization, with low inference time and compact model size.
– The framework performs competitively in text-to-audio generation, indicating joint training benefits both multi-modal and single-modality tasks.
๐ Paper link: https://huggingface.co/papers/2412.15322
5. CLEAR: Conv-Like Linearization Revs Pre-Trained Diffusion Transformers Up
๐ Keywords: Diffusion Transformers, linear attention mechanism, CLEAR, high-resolution images, zero-shot generalization
๐ก Category: Generative Models
๐ Research Objective:
– To develop a linear attention mechanism to reduce the complexity of pre-trained Diffusion Transformers while maintaining high performance in high-resolution image generation.
๐ ๏ธ Research Methods:
– Analyzed existing efficient attention mechanisms.
– Identified key factors for successful linearization: locality, formulation consistency, high-rank attention maps, and feature integrity.
– Proposed a local attention strategy called CLEAR to limit feature interactions to a local window.
๐ฌ Research Conclusions:
– Successfully reduced attention computations by 99.5% and accelerated generation by 6.3 times for 8K-resolution images.
– Achieved similar results to the teacher model with significantly lower complexity.
– Demonstrated advantageous properties in distilled layers, including zero-shot generalization and improved support for multi-GPU parallel inference.
๐ Paper link: https://huggingface.co/papers/2412.16112
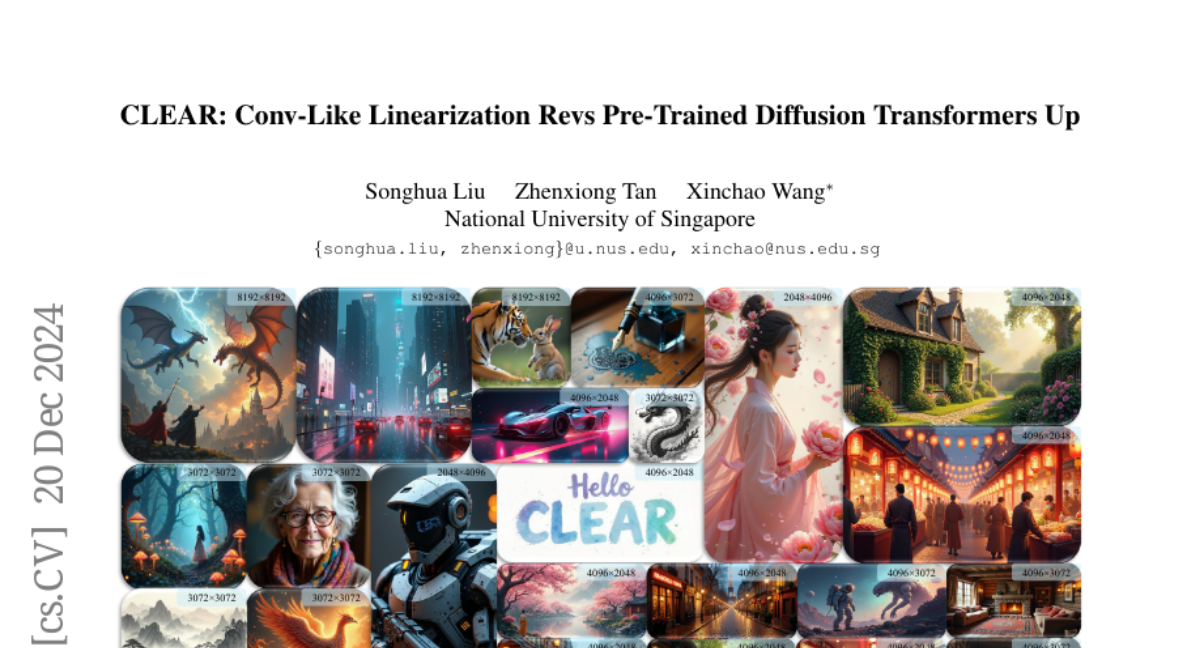
6. Toward Robust Hyper-Detailed Image Captioning: A Multiagent Approach and Dual Evaluation Metrics for Factuality and Coverage
๐ Keywords: Multimodal large language models, hallucination detection, LLM-MLLM collaboration, GPT-4V
๐ก Category: Multi-Modal Learning
๐ Research Objective:
– Address hallucinations in detailed captions generated by Multimodal large language models (MLLMs).
๐ ๏ธ Research Methods:
– Introduce a multiagent approach with LLM-MLLM collaboration to correct captions and develop an evaluation framework and benchmark dataset.
๐ฌ Research Conclusions:
– The proposed method enhances the factual accuracy of captions and better aligns with human judgment compared to existing metrics, even outperforming in hyper-detailed image captioning tasks.
๐ Paper link: https://huggingface.co/papers/2412.15484
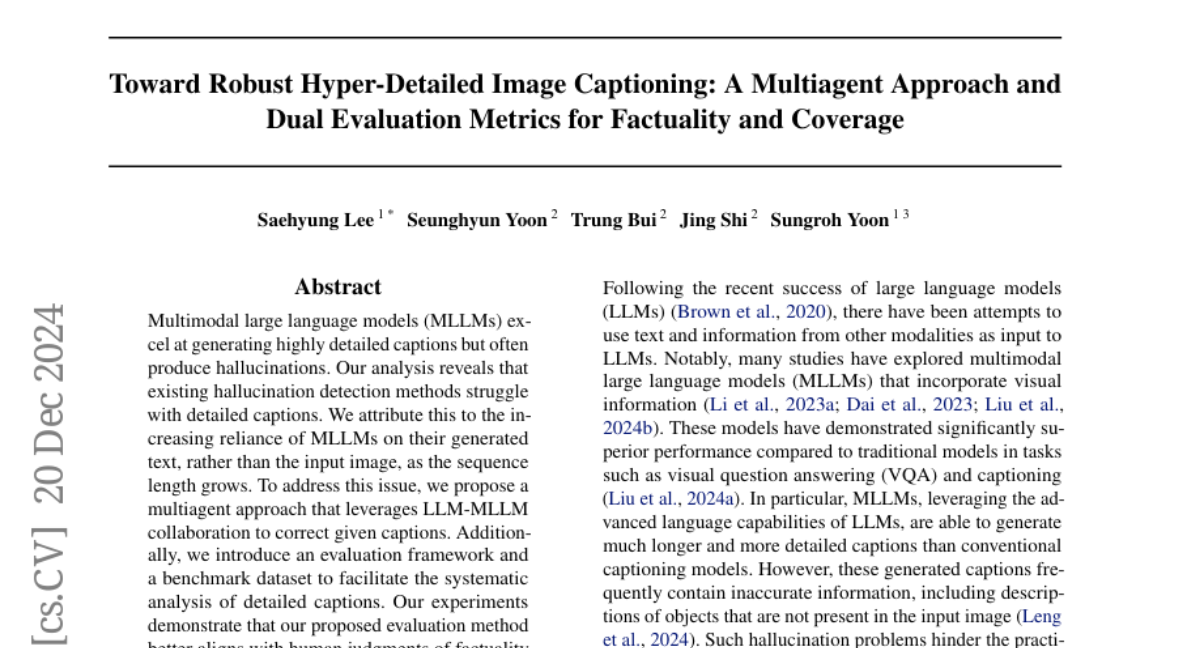
7. Sequence Matters: Harnessing Video Models in 3D Super-Resolution
๐ Keywords: 3D Super-Resolution, Video Super-Resolution, Spatial Consistency, Low-Resolution Images, Benchmark Datasets
๐ก Category: Computer Vision
๐ Research Objective:
– To improve 3D super-resolution models by leveraging video super-resolution techniques to enhance spatial consistency and accuracy in reconstructed 3D models from low-resolution multi-view images.
๐ ๏ธ Research Methods:
– A comprehensive study was conducted utilizing video super-resolution models to align low-resolution images effectively, without the need for fine-tuning, ensuring consistent spatial information.
๐ฌ Research Conclusions:
– The study demonstrates that video super-resolution models effectively handle sequences lacking precise spatial alignment, achieving state-of-the-art results in 3D super-resolution on datasets such as NeRF-synthetic and MipNeRF-360.
๐ Paper link: https://huggingface.co/papers/2412.11525
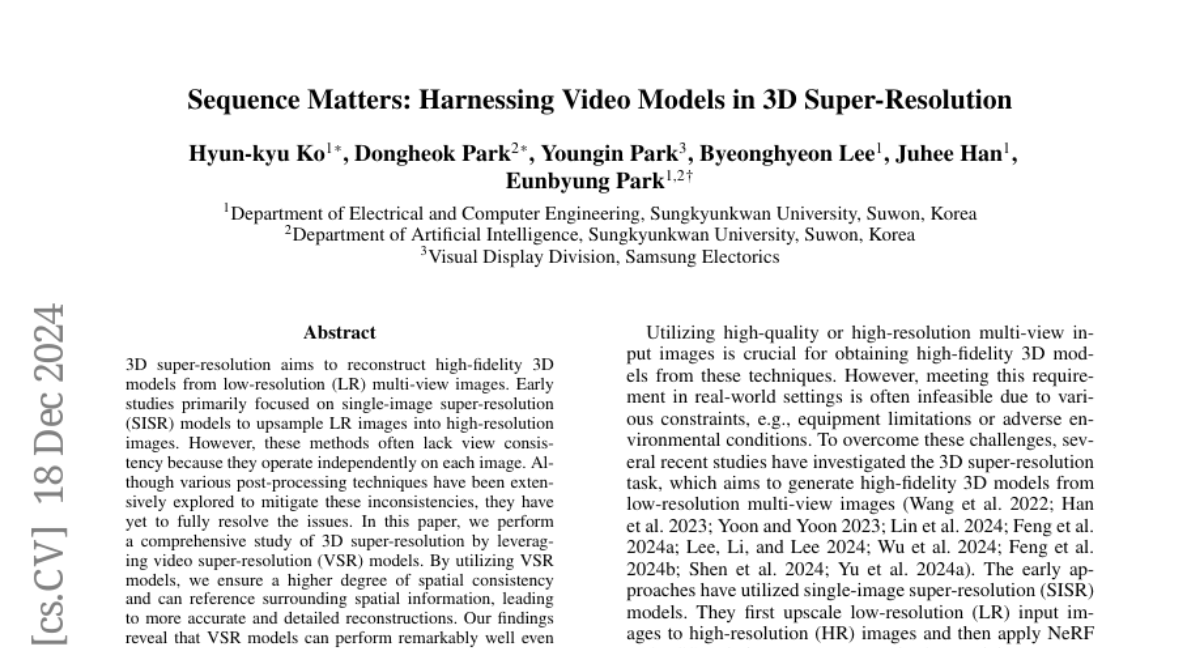
8. MixLLM: LLM Quantization with Global Mixed-precision between Output-features and Highly-efficient System Design
๐ Keywords: Quantization, Mixed-Precision, System Efficiency, LLMs, Output Features
๐ก Category: AI Systems and Tools
๐ Research Objective:
– To analyze and optimize quantization principles to improve the accuracy, memory consumption, and efficiency of quantized LLMs.
๐ ๏ธ Research Methods:
– Developed MixLLM, employing mixed-precision quantization that focuses on globally significant output features.
– Utilized two-step dequantization and a software pipeline for minimizing dequantization overhead and enhancing system performance.
๐ฌ Research Conclusions:
– MixLLM achieves reduced PPL increasement and enhances accuracy with less memory usage and improved system efficiency over state-of-the-art methods.
๐ Paper link: https://huggingface.co/papers/2412.14590
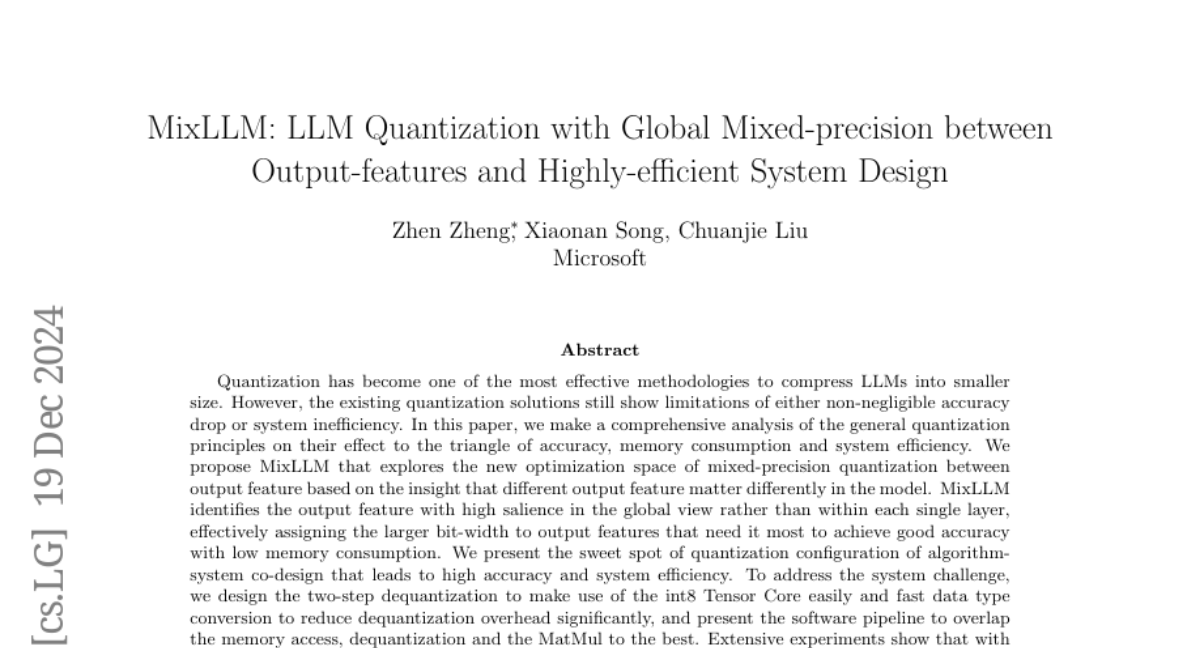
9. TRecViT: A Recurrent Video Transformer
๐ Keywords: Gated Linear Recurrent Units, Self-Attention, Video Modelling, TRecViT
๐ก Category: Computer Vision
๐ Research Objective:
– The development of a novel block specifically designed for video modelling through a unique time-space-channel factorisation approach.
๐ ๏ธ Research Methods:
– Utilization of gated linear recurrent units for temporal information mixing, self-attention for spatial mixing, and MLPs for channel mixing, integrated into the architecture known as TRecViT.
๐ฌ Research Conclusions:
– TRecViT demonstrates superior or equivalent performance compared to a pure attention model (ViViT-L) on large-scale video datasets, with significantly fewer parameters, smaller memory footprint, and lower FLOPs count.
๐ Paper link: https://huggingface.co/papers/2412.14294
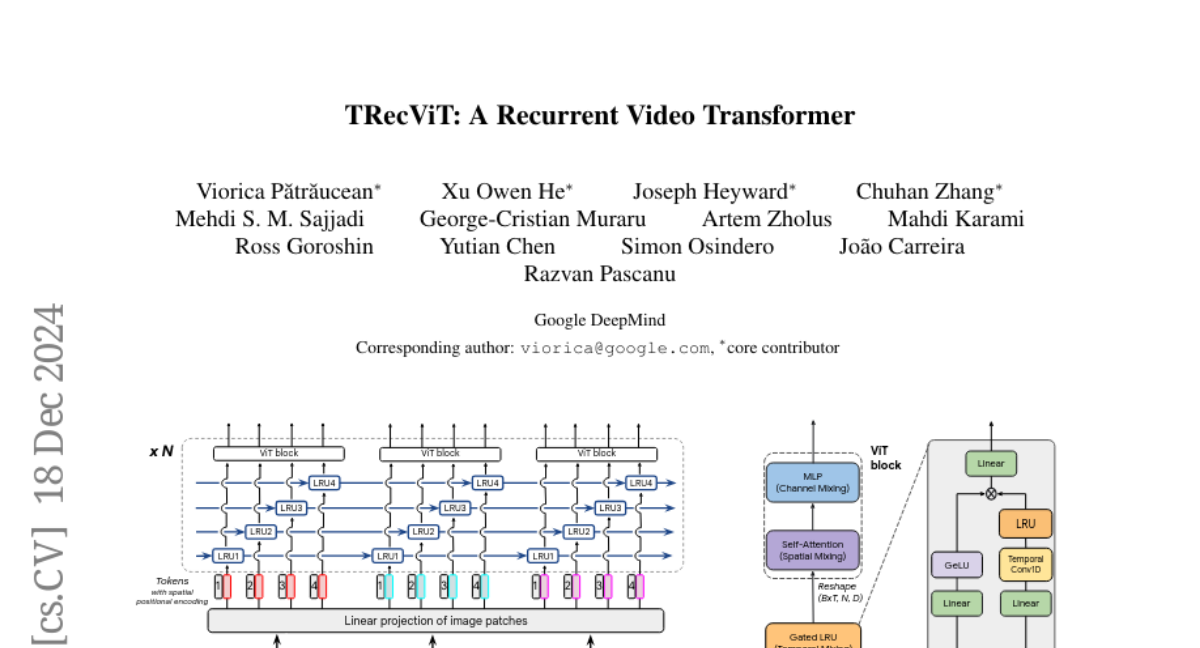
10. Fietje: An open, efficient LLM for Dutch
๐ Keywords: Small Language Models, Dutch language, Transparency, Reproducibility, Evaluation
๐ก Category: Natural Language Processing
๐ Research Objective:
– To introduce Fietje, a family of small language models specifically designed for processing the Dutch language.
๐ ๏ธ Research Methods:
– Utilization of Phi 2, an English-centric model with 2.7 billion parameters, to develop Fietje, ensuring the model is fully open-source with publicly accessible resources for transparency and reproducibility.
๐ฌ Research Conclusions:
– Fietje, despite being a smaller language model, demonstrates competitive performance against larger models, showcasing the potential and rapid progress for compact language models in Dutch language processing.
๐ Paper link: https://huggingface.co/papers/2412.15450
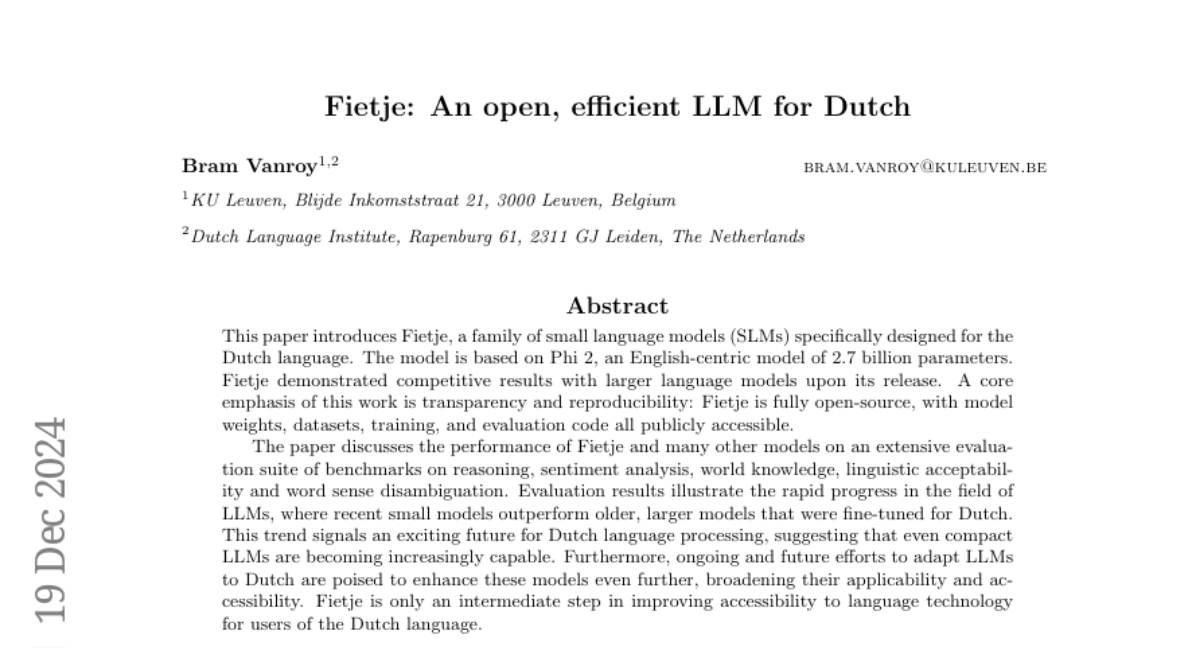
11. LLMs Lost in Translation: M-ALERT uncovers Cross-Linguistic Safety Gaps
๐ Keywords: Large Language Models, M-ALERT, multilingual benchmark, safety analysis, linguistic diversity
๐ก Category: AI Ethics and Fairness
๐ Research Objective:
– The research introduces M-ALERT, a multilingual benchmark designed to evaluate the safety of Large Language Models (LLMs) across five languages, aiming to ensure safe access and promote linguistic diversity.
๐ ๏ธ Research Methods:
– The study employs 15k high-quality prompts per language across five languages, totaling 75k prompts, following the ALERT taxonomy, to assess the safety of 10 state-of-the-art LLMs.
๐ฌ Research Conclusions:
– Experiments reveal significant inconsistencies in safety across languages and categories; for example, the Llama3.2 model shows a high level of unsafety in the crime_tax category in Italian, while remaining safer in other languages. The need for robust multilingual safety practices in LLMs is emphasized to promote safe usage across diverse communities.
๐ Paper link: https://huggingface.co/papers/2412.15035
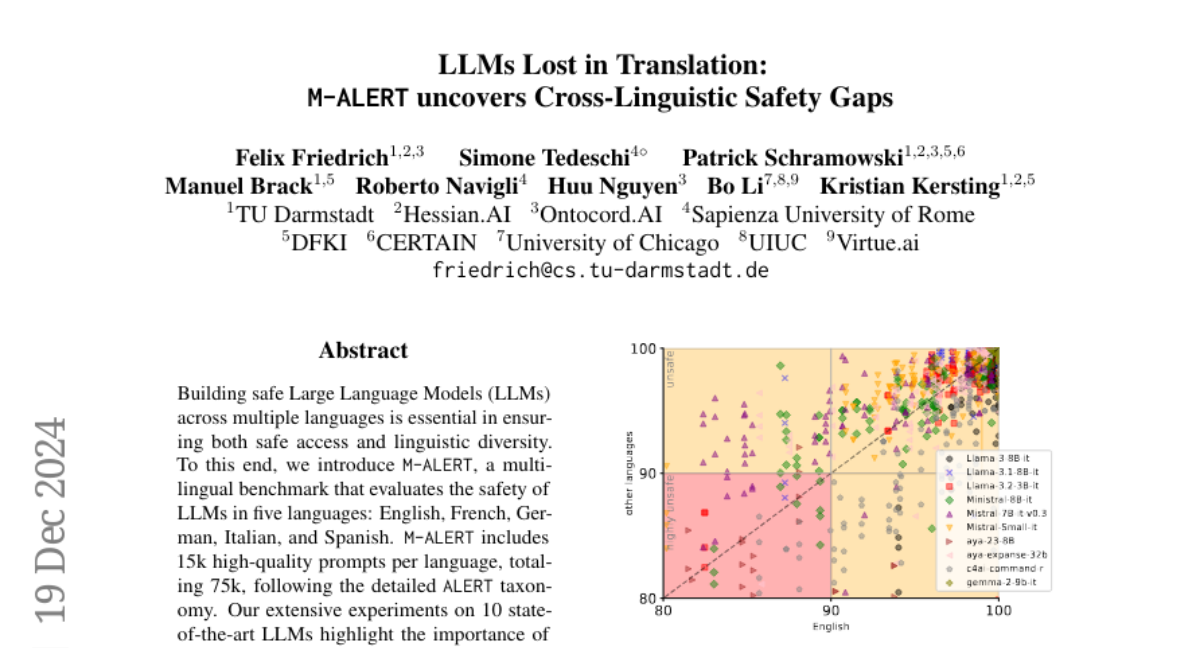
12. IDOL: Instant Photorealistic 3D Human Creation from a Single Image
๐ Keywords: High-fidelity, 3D full-body avatar, Transformer model, Photorealistic, Single image
๐ก Category: Computer Vision
๐ Research Objective:
– To create a high-fidelity, animatable 3D full-body avatar from a single image leveraging a new dataset and model architecture.
๐ ๏ธ Research Methods:
– Development of HuGe100K, a large-scale dataset of diverse human images.
– Use of a feed-forward transformer model to predict a 3D human Gaussian representation for pose and body shape disentanglement.
๐ฌ Research Conclusions:
– The model efficiently reconstructs photorealistic humans at 1K resolution from a single input image using a single GPU and supports shape and texture editing without post-processing.
๐ Paper link: https://huggingface.co/papers/2412.14963
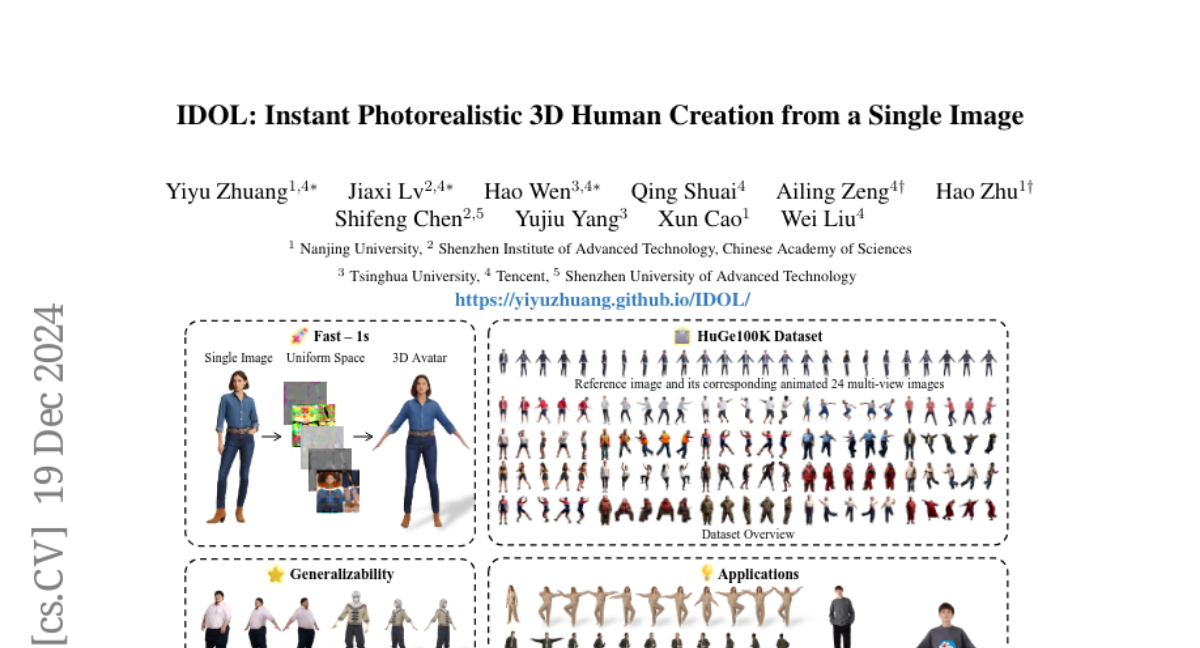
13. Multi-LLM Text Summarization
๐ Keywords: Multi-LLM, Summarization, Decentralized, Centralized
๐ก Category: Natural Language Processing
๐ Research Objective:
– The paper proposes a Multi-LLM summarization framework.
๐ ๏ธ Research Methods:
– It investigates centralized and decentralized multi-LLM strategies for text summarization, with crucial steps of generation and evaluation.
๐ฌ Research Conclusions:
– The Multi-LLM approaches significantly outperform single LLM baselines by up to 3x, demonstrating their effectiveness in summarization tasks.
๐ Paper link: https://huggingface.co/papers/2412.15487
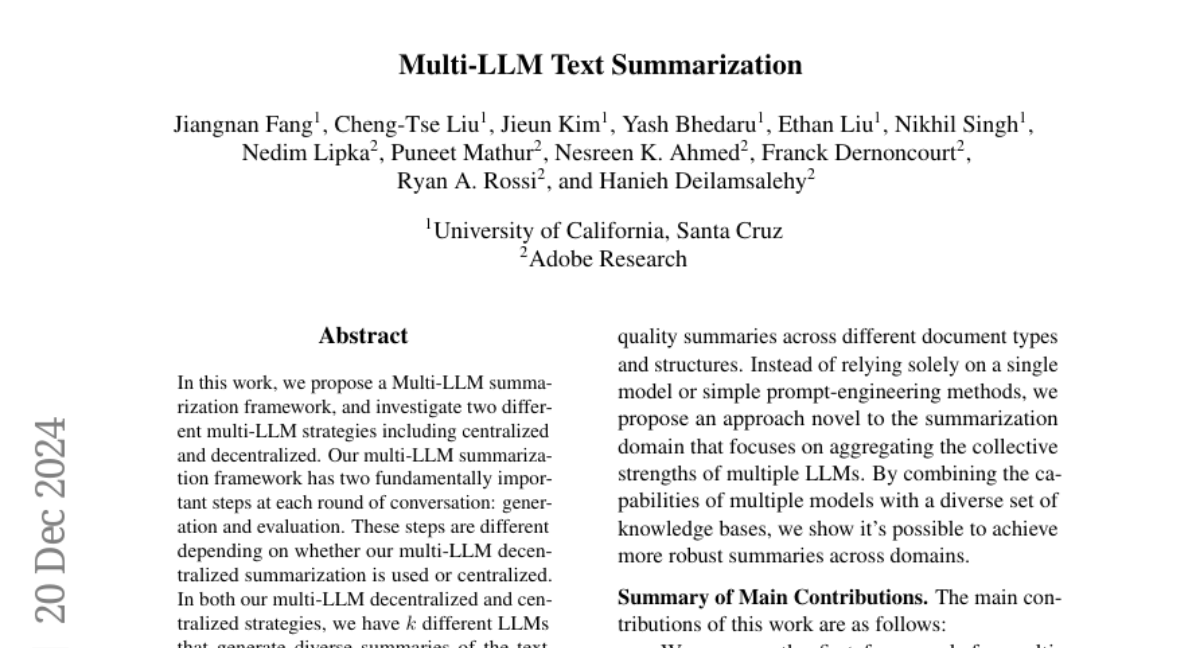