AI Native Daily Paper Digest – 20250106
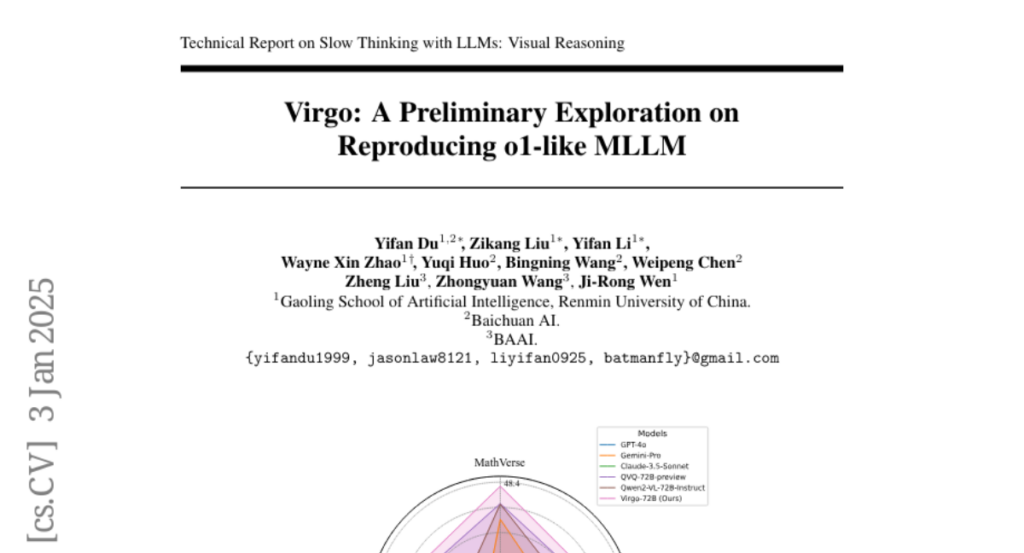
1. EnerVerse: Envisioning Embodied Future Space for Robotics Manipulation
π Keywords: EnerVerse, robotic manipulation, Free Anchor View, 4D Gaussian Splatting, sim-to-real gap
π‘ Category: Robotics and Autonomous Systems
π Research Objective:
– The study aims to develop EnerVerse, a framework designed to enhance future space generation for robotic manipulation tasks.
π οΈ Research Methods:
– EnerVerse incorporates convolutional and bidirectional attention mechanisms, alongside a sparse memory context and a generative paradigm to handle video data efficiently.
– Introduction of the Free Anchor View (FAV) space and utilization of a data engine with 4D Gaussian Splatting for improved data quality and diversity.
π¬ Research Conclusions:
– The implementation of EnerVerse provides significant improvements in policy predictive capabilities, enhancing the overall performance of robots, especially in long-range manipulation tasks.
π Paper link: https://huggingface.co/papers/2501.01895
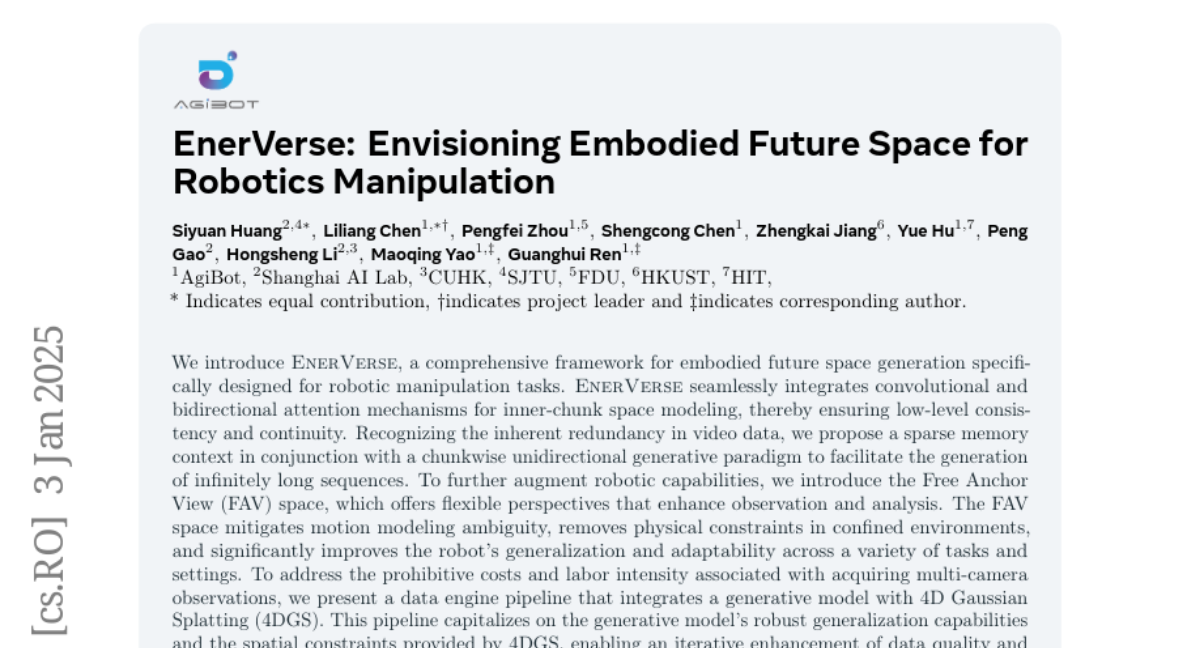
2. VITA-1.5: Towards GPT-4o Level Real-Time Vision and Speech Interaction
π Keywords: Multimodal Large Language Models, vision and speech interaction, end-to-end response speed
π‘ Category: Multi-Modal Learning
π Research Objective:
– To enhance multimodal dialogue systems by integrating vision and speech modalities through a multi-stage training methodology.
π οΈ Research Methods:
– A carefully designed multi-stage training approach that enables LLM to understand both visual and speech information without relying on separate ASR and TTS modules.
π¬ Research Conclusions:
– The proposed model excels in both visual and speech tasks, achieving near real-time interaction capabilities and outperforming state-of-the-art methods in relevant benchmarks.
π Paper link: https://huggingface.co/papers/2501.01957
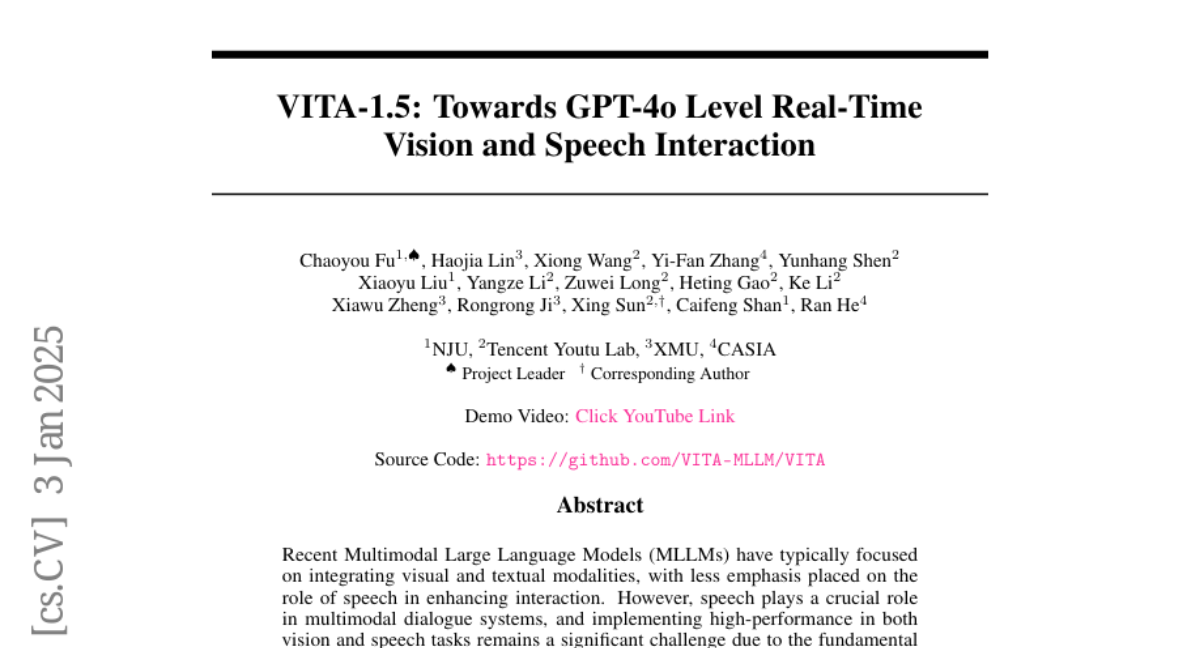
3. Virgo: A Preliminary Exploration on Reproducing o1-like MLLM
π Keywords: Multimodal Large Language Models, Slow-thinking reasoning, Textual reasoning data
π‘ Category: Multi-Modal Learning
π Research Objective:
– The research aims to explore the implementation of slow-thinking reasoning systems in multimodal large language models (MLLMs) by utilizing textual long-form thought data.
π οΈ Research Methods:
– The study involves fine-tuning a multimodal LLM with a small set of textual data to develop a multimodal slow-thinking system named Virgo.
π¬ Research Conclusions:
– It concludes that textual reasoning data is more effective than visual reasoning data for eliciting slow-thinking capacities in MLLMs and that these capacities are fundamentally linked to the language model component, transferable across modalities or domains.
π Paper link: https://huggingface.co/papers/2501.01904
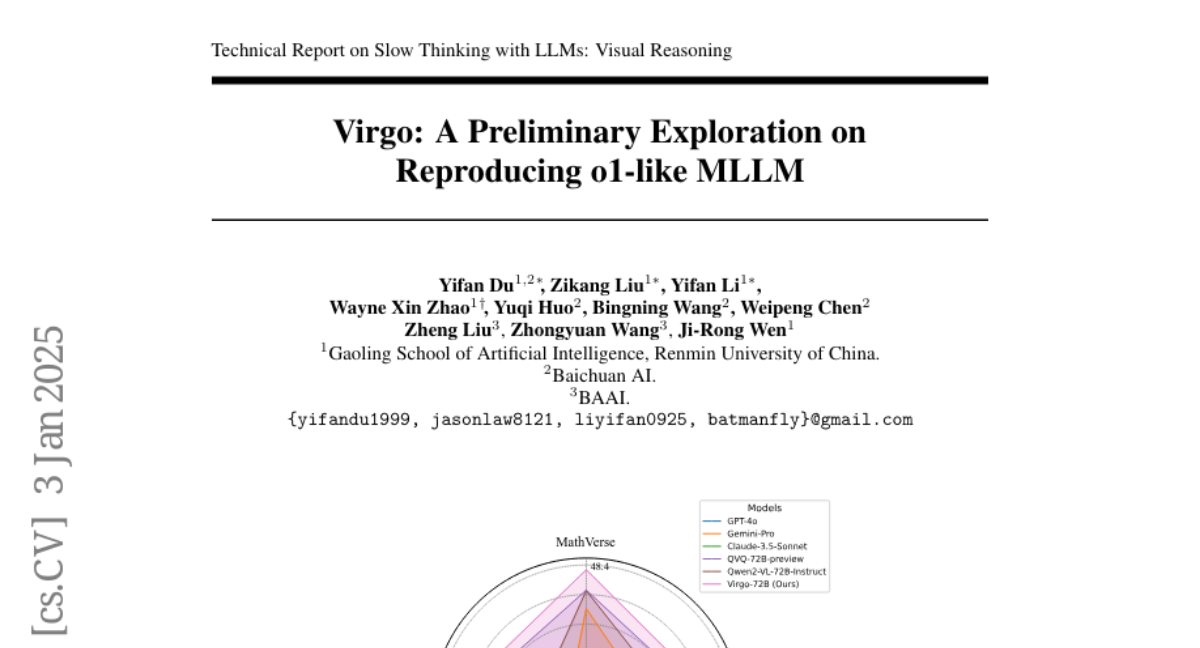
4. VisionReward: Fine-Grained Multi-Dimensional Human Preference Learning for Image and Video Generation
π Keywords: VisionReward, video generation, human preference, AI Native, video quality assessment
π‘ Category: Generative Models
π Research Objective:
– Introduce a strategy for aligning visual generation models with human preferences through the development of VisionReward.
π οΈ Research Methods:
– Design a multi-dimensional reward model based on decomposing human preferences into judgment questions for images and videos, and implement a multi-objective preference learning algorithm.
π¬ Research Conclusions:
– VisionReward surpasses existing scoring methods by 17.2% in video preference prediction and exhibits top performance according to both machine metrics and human evaluation.
π Paper link: https://huggingface.co/papers/2412.21059
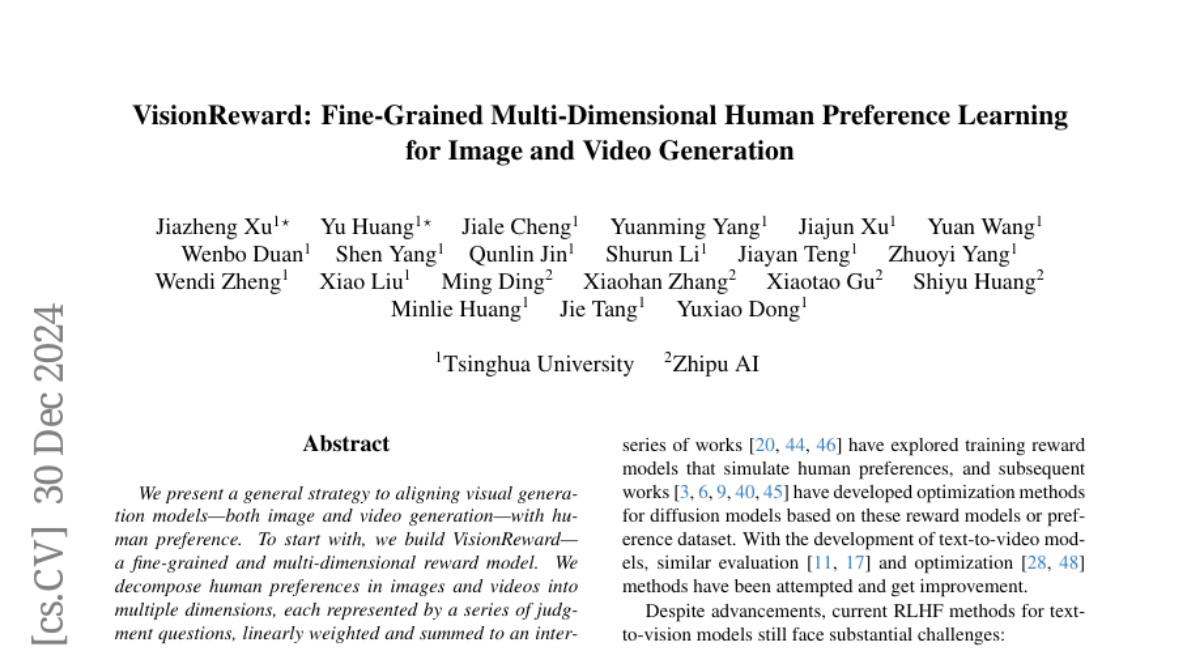
5. SDPO: Segment-Level Direct Preference Optimization for Social Agents
π Keywords: Large Language Models, Direct Preference Optimization, Social Agents, Multi-Turn Interactions
π‘ Category: Natural Language Processing
π Research Objective:
– To propose a Segment-Level Direct Preference Optimization (SDPO) method for enhancing multi-turn agent behavior in social dialogues.
π οΈ Research Methods:
– Utilize SDPO to optimize specific key segments within interactions, aiming to balance between turn-level and session-level approaches.
π¬ Research Conclusions:
– SDPO-tuned agents outperform existing DPO-based methods and proprietary LLMs like GPT-4o, demonstrating improved social intelligence in language model-based agents.
π Paper link: https://huggingface.co/papers/2501.01821
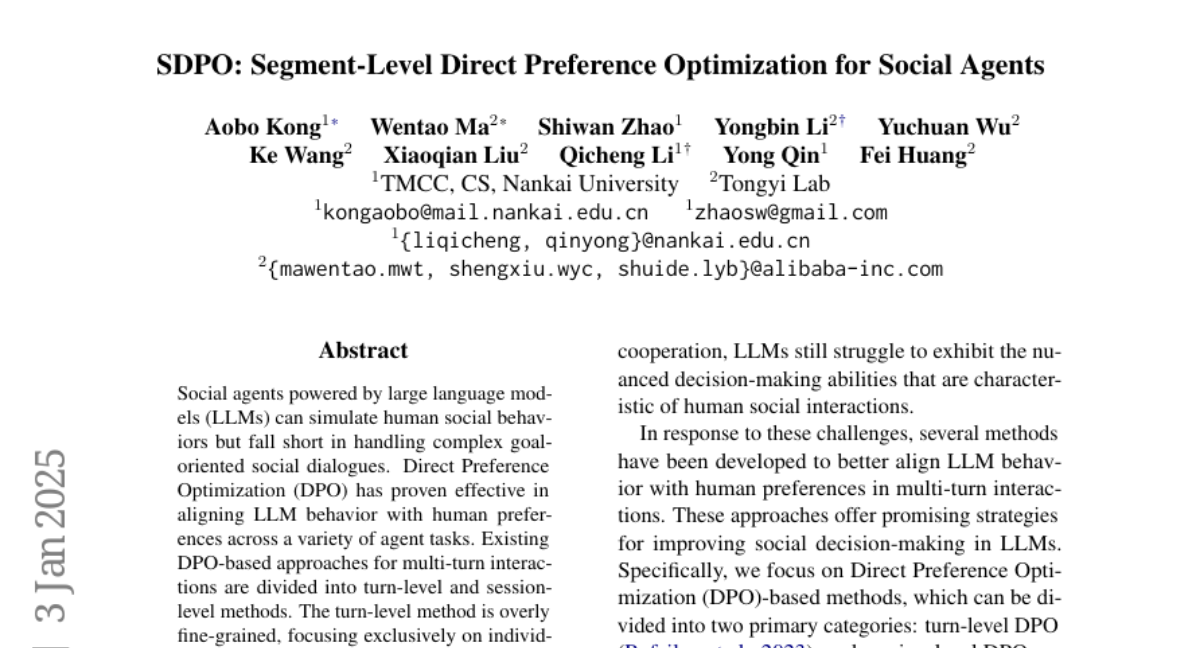
6. Graph Generative Pre-trained Transformer
π Keywords: Graph generation, Graph Generative Pre-trained Transformer (G2PT), Molecular design, Structured data
π‘ Category: Generative Models
π Research Objective:
– This study revisits sequence-based graph representation to improve the efficiency of graph generation.
π οΈ Research Methods:
– The introduction of an auto-regressive model, G2PT, for learning graph structures through next-token prediction and its fine-tuning for goal-oriented generation and property prediction.
π¬ Research Conclusions:
– G2PT demonstrates superior generative performance and adaptability across multiple graph datasets and downstream tasks, showing strong potential in molecular design and property prediction.
π Paper link: https://huggingface.co/papers/2501.01073
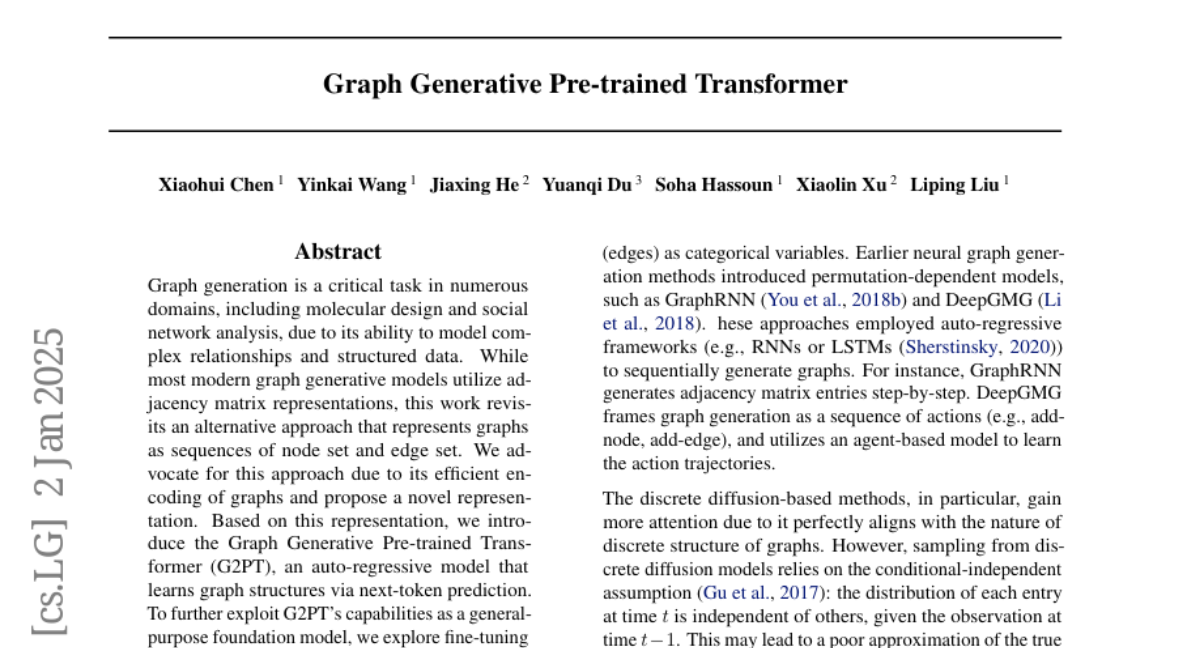
7. LUSIFER: Language Universal Space Integration for Enhanced Multilingual Embeddings with Large Language Models
π Keywords: LUSIFER, large language models, multilingual embedding, zero-shot
π‘ Category: Natural Language Processing
π Research Objective:
– The study aims to introduce LUSIFER, a novel zero-shot approach to adapt LLM-based embedding models for multilingual tasks without requiring multilingual supervision.
π οΈ Research Methods:
– LUSIFER integrates a multilingual encoder with an LLM-based embedding model optimized for specific tasks using minimal trainable parameters to facilitate language transfer.
π¬ Research Conclusions:
– LUSIFER enhances multilingual performance significantly, especially in medium and low-resource languages, without the need for explicit multilingual training data, as demonstrated by comprehensive evaluations across 14 languages.
π Paper link: https://huggingface.co/papers/2501.00874
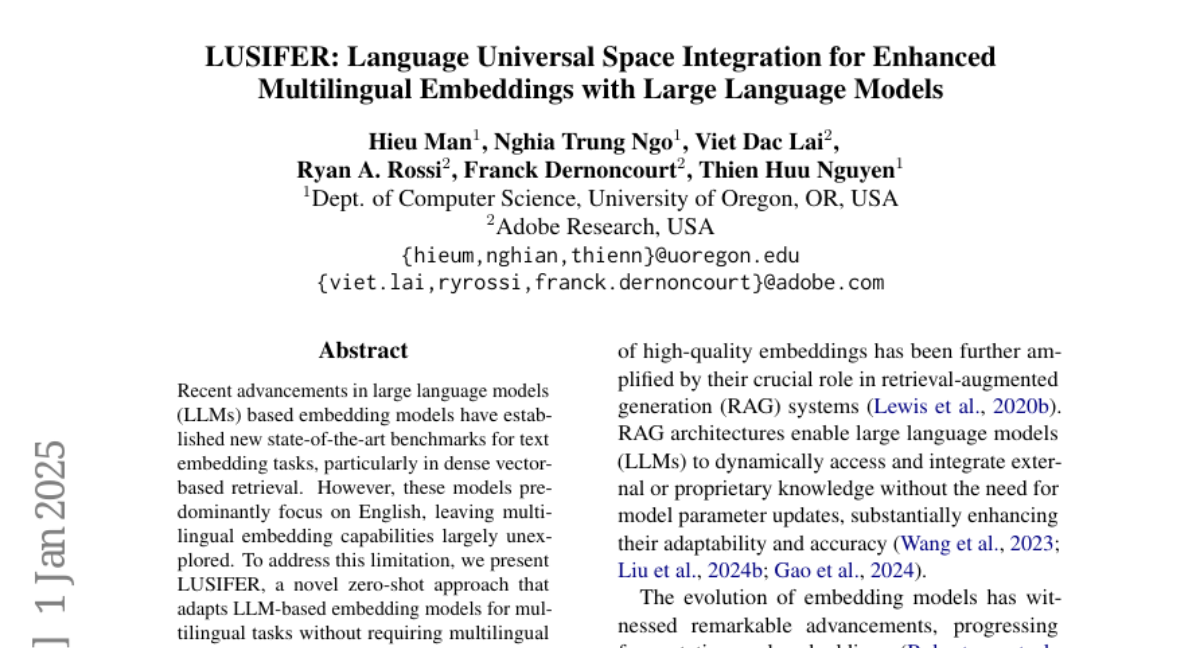
8. BoxingGym: Benchmarking Progress in Automated Experimental Design and Model Discovery
π Keywords: LLM-based scientific agents, experimental design, model discovery, generative probabilistic model, expected information gain
π‘ Category: Foundations of AI
π Research Objective:
– To create a benchmark called BoxingGym for evaluating the ability of AI agents to propose scientific models, collect experimental data, and revise theories systematically.
π οΈ Research Methods:
– Implementation of 10 environments using generative probabilistic models across real-world scientific domains, assessing experimental design via expected information gain and model discovery through prediction reliability and standard metrics.
π¬ Research Conclusions:
– Current LLMs, such as GPT-4o, face challenges in experimental design and model discovery, and augmenting them with statistical models does not notably enhance performance.
π Paper link: https://huggingface.co/papers/2501.01540
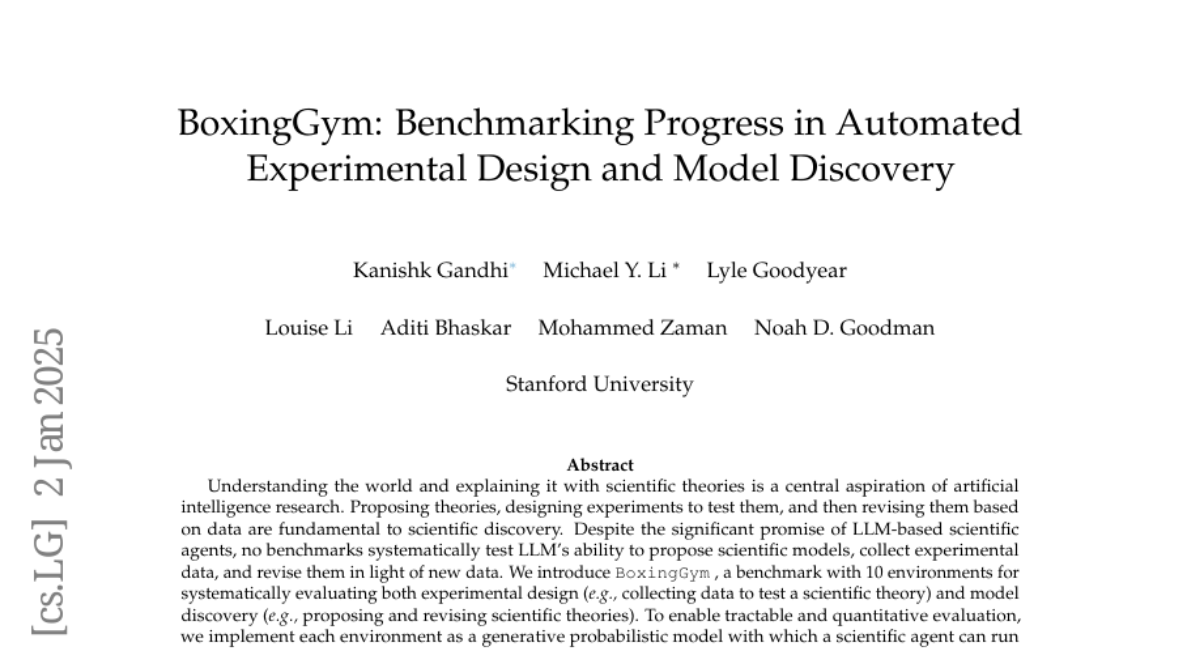