AI Native Daily Paper Digest – 20250107
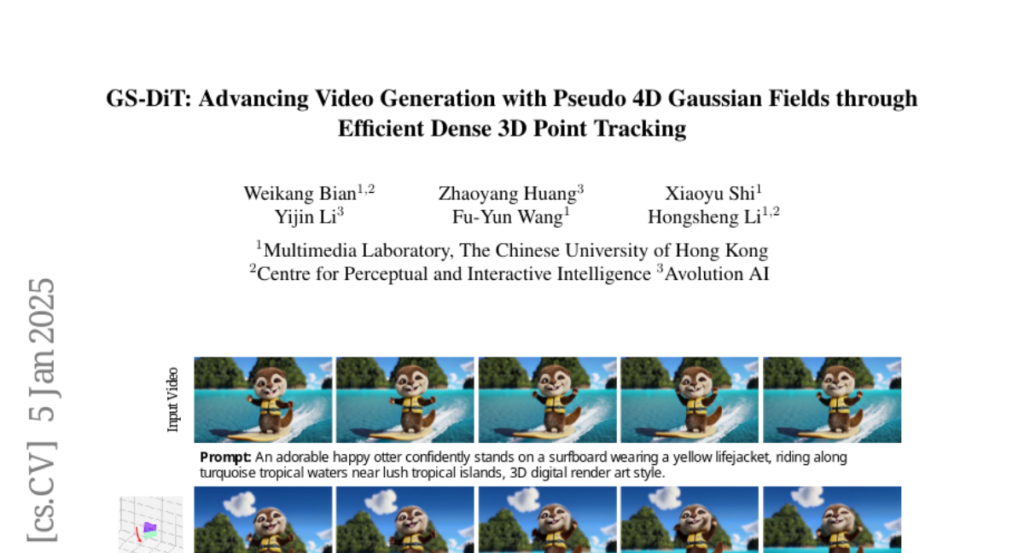
1. STAR: Spatial-Temporal Augmentation with Text-to-Video Models for Real-World Video Super-Resolution
π Keywords: Video Super-Resolution, Temporal Consistency, T2V Models, GAN, Artifacts
π‘ Category: Computer Vision
π Research Objective:
– Address the over-smoothing issue in GAN-based video super-resolution models by improving temporal consistency with T2V models.
π οΈ Research Methods:
– Introduce a Local Information Enhancement Module (LIEM) to enrich local details and mitigate degradation artifacts.
– Propose a Dynamic Frequency Loss to enhance fidelity and guide the model’s focus during diffusion steps.
π¬ Research Conclusions:
– The presented approach, leveraging T2V models, outperforms state-of-the-art methods in both synthetic and real-world datasets by achieving realistic spatial details and robust temporal consistency.
π Paper link: https://huggingface.co/papers/2501.02976
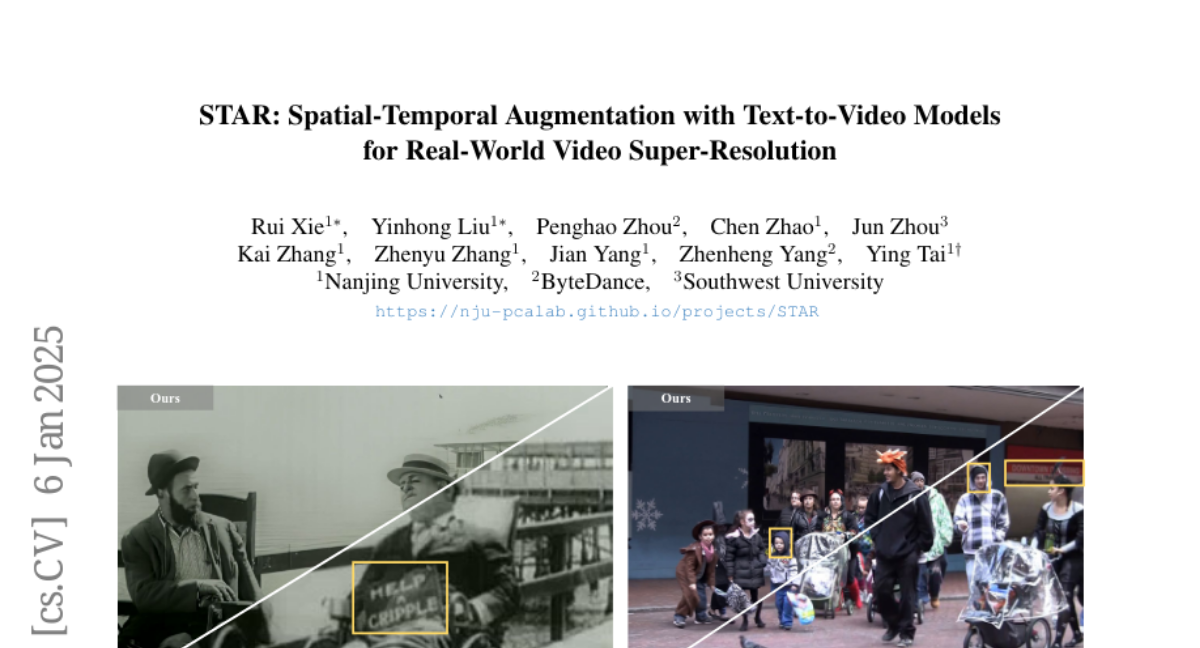
2. Dispider: Enabling Video LLMs with Active Real-Time Interaction via Disentangled Perception, Decision, and Reaction
π Keywords: Active Real-time interaction, Video LLMs, Dispider, Proactive streaming, Decision and Reaction
π‘ Category: Human-AI Interaction
π Research Objective:
– Introduce a new paradigm for human-computer interaction using Video LLMs that allows real-time interaction by understanding user intent and processing streaming video simultaneously.
π οΈ Research Methods:
– Development of Dispider, a system that disentangles perception, decision, and reaction processes, using a lightweight proactive streaming video processing module and asynchronous interaction module to optimize real-time interactions.
π¬ Research Conclusions:
– Dispider demonstrates strong performance in both conventional video QA tasks and significantly outperforms existing models in streaming scenarios, validating the proposed architecture’s effectiveness.
π Paper link: https://huggingface.co/papers/2501.03218
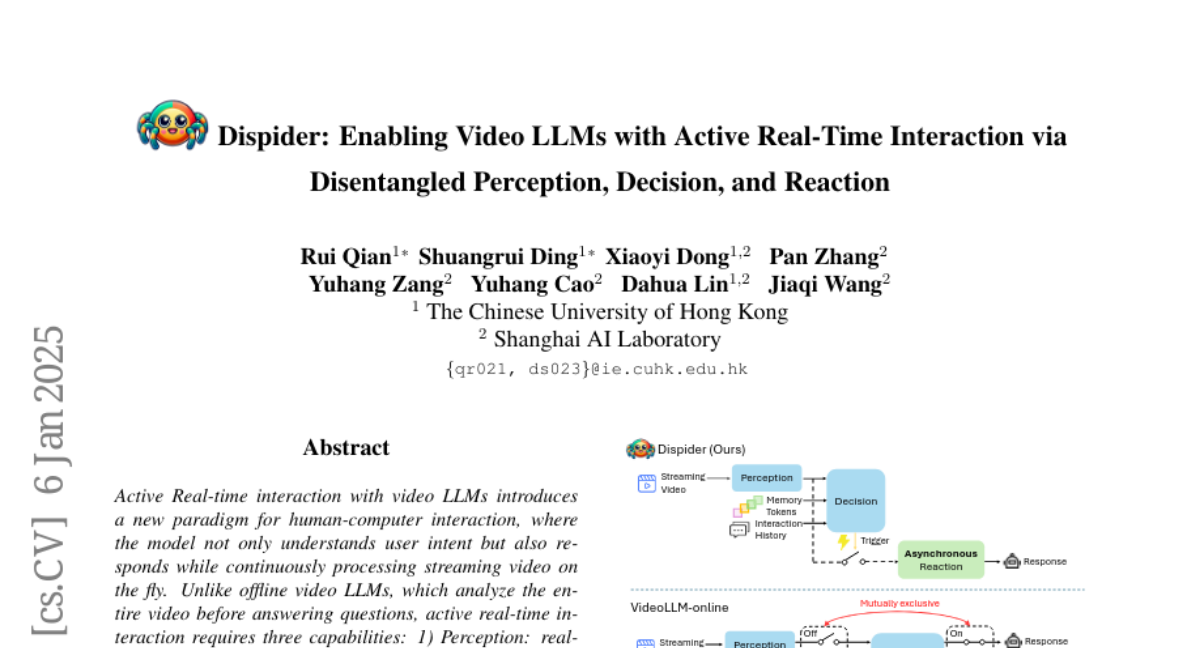
3. BoostStep: Boosting mathematical capability of Large Language Models via improved single-step reasoning
π Keywords: Large Language Models, In-Context Learning, BoostStep, Monte Carlo Tree Search, Reasoning Quality
π‘ Category: Natural Language Processing
π Research Objective:
– To enhance reasoning quality within each step of large language models when solving complex math problems by addressing granularity mismatches and negative-effect noise issues in in-context learning examples.
π οΈ Research Methods:
– Introduction of BoostStep to align granularity and provide highly relevant in-context learning examples through a novel ‘first-try’ strategy, integrating with Monte Carlo Tree Search methods for improved reasoning and decision-making.
π¬ Research Conclusions:
– BoostStep increases model reasoning quality, leading to a performance improvement of 3.6% for GPT-4o and 2.0% for Qwen2.5-Math-72B on mathematical benchmarks, with a 7.5% gain when combined with Monte Carlo Tree Search.
π Paper link: https://huggingface.co/papers/2501.03226
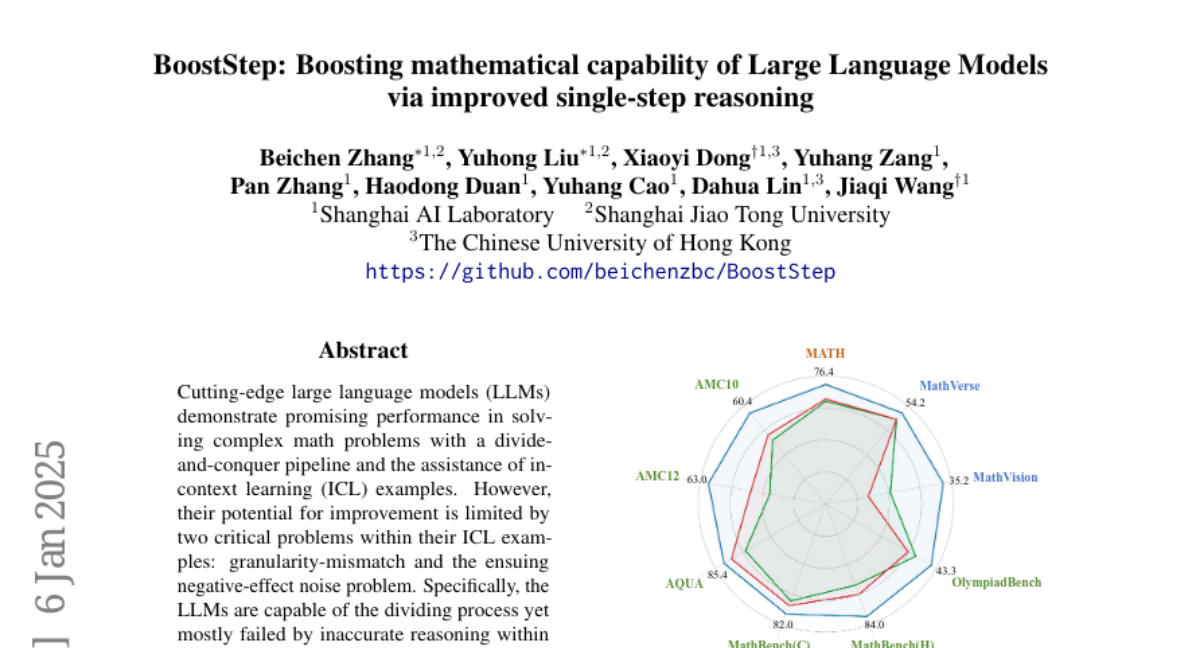
4. Test-time Computing: from System-1 Thinking to System-2 Thinking
π Keywords: test-time computing, System-2 thinking, reasoning ability, distribution shifts, model robustness
π‘ Category: Knowledge Representation and Reasoning
π Research Objective:
– To survey the concept and application of test-time computing scaling, tracing its evolution from System-1 to System-2 models.
π οΈ Research Methods:
– Analysis of test-time computing’s role in System-1 models addressing distribution shifts and enhancing robustness through parameter updating and input modification, and in System-2 models improving reasoning ability through repeated sampling and tree search.
π¬ Research Conclusions:
– The survey highlights the significance of test-time computing in transitioning from System-1 models to strong System-2 models and suggests potential future research directions.
π Paper link: https://huggingface.co/papers/2501.02497
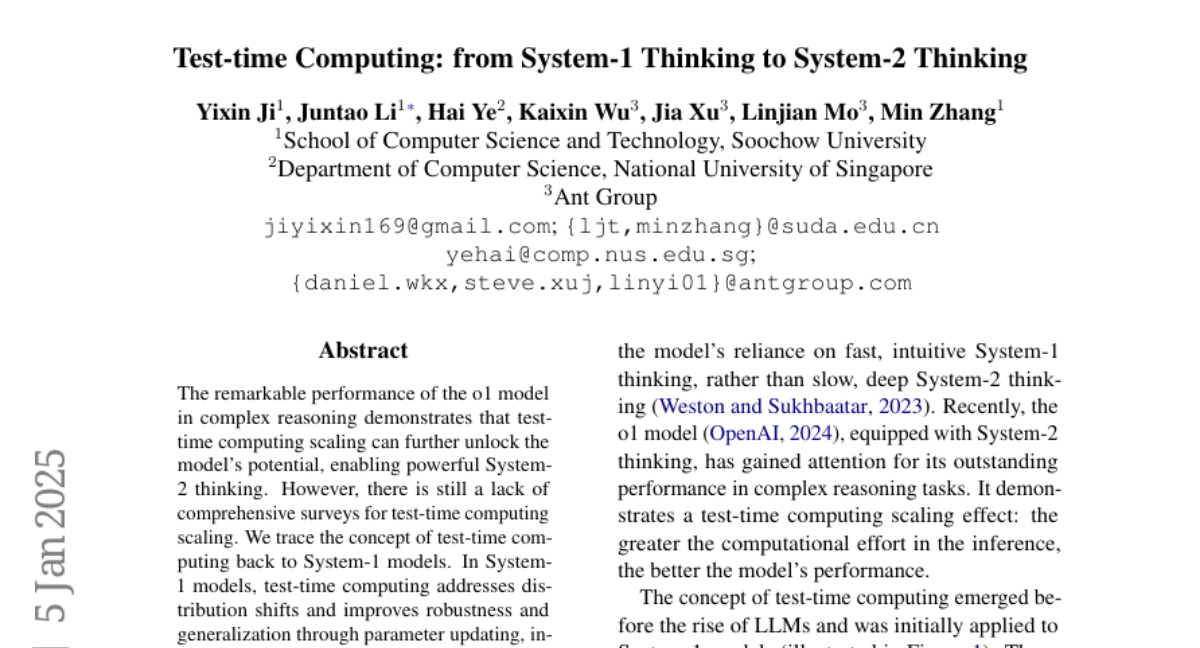
5. Personalized Graph-Based Retrieval for Large Language Models
π Keywords: Personalized Graph-based Retrieval-Augmented Generation, PGraphRAG, Large Language Models, User-Centric Knowledge Graphs
π‘ Category: Natural Language Processing
π Research Objective:
– To enhance personalization in text generation using Personalized Graph-based Retrieval-Augmented Generation (PGraphRAG) by integrating user-centric knowledge graphs.
π οΈ Research Methods:
– Developed a framework that enriches personalized responses by integrating structured user knowledge into the retrieval process and augmenting prompts with user-relevant context.
π¬ Research Conclusions:
– PGraphRAG significantly outperforms state-of-the-art personalization methods, showcasing the unique benefits of graph-based retrieval approaches in scenarios with sparse user history.
π Paper link: https://huggingface.co/papers/2501.02157
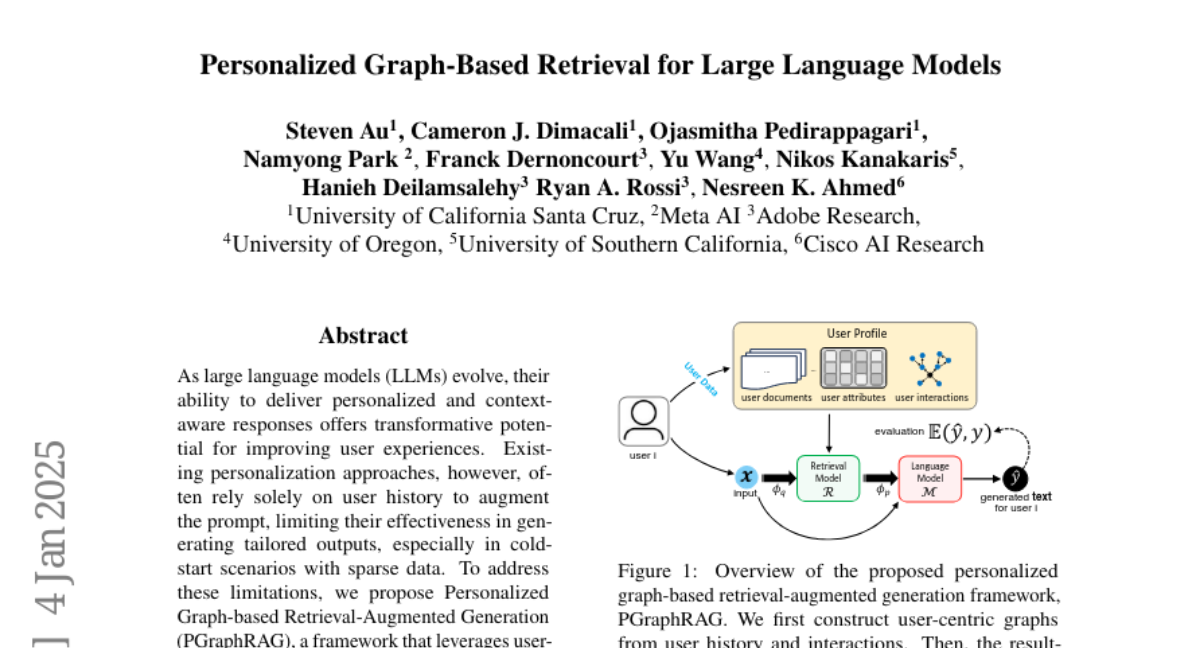
6. METAGENE-1: Metagenomic Foundation Model for Pandemic Monitoring
π Keywords: METAGENE-1, metagenomic, pathogen detection, pandemic monitoring, genomic benchmarks
π‘ Category: AI in Healthcare
π Research Objective:
– The objective is to pretrain the METAGENE-1 model on diverse metagenomic DNA and RNA sequences to improve pandemic monitoring and pathogen detection.
π οΈ Research Methods:
– Utilized byte-pair encoding (BPE) tokenization tailored for metagenomic sequences and detailed the pretraining dataset, tokenization strategy, and model architecture.
π¬ Research Conclusions:
– METAGENE-1 achieves state-of-the-art results in genomic benchmarks and has potential applications in public health for biosurveillance and early detection of emerging health threats.
π Paper link: https://huggingface.co/papers/2501.02045
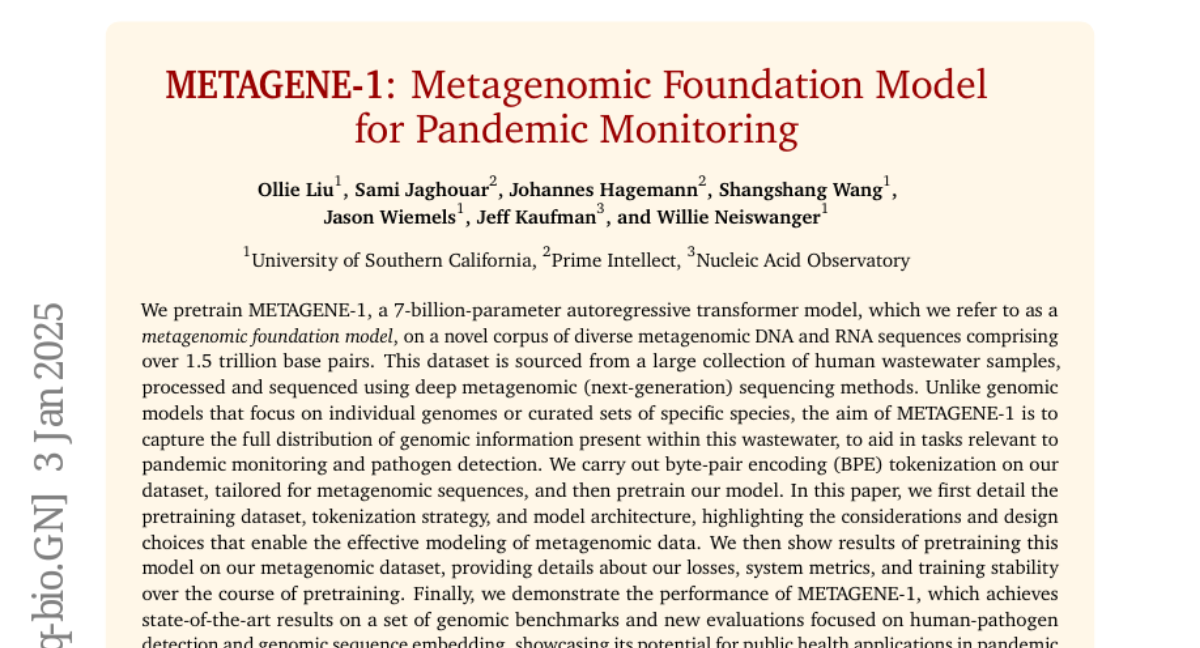
7. GS-DiT: Advancing Video Generation with Pseudo 4D Gaussian Fields through Efficient Dense 3D Point Tracking
π Keywords: 4D video control, Video Diffusion Transformer, pseudo 4D Gaussian fields, Dense 3D Point Tracking
π‘ Category: Generative Models
π Research Objective:
– The research aims to enhance video generation with 4D video control through advanced cinematic techniques unsupported by current methods.
π οΈ Research Methods:
– Introduction of a novel framework using pseudo 4D Gaussian fields with dense 3D point tracking.
– Fine-tuning of a pretrained Video Diffusion Transformer (DiT) to generate videos aligned with these rendered fields.
π¬ Research Conclusions:
– Demonstration of strong generalization in generating videos with dynamic content and different camera parameters.
– GS-DiT supports advanced cinematic effects, making it a valuable tool for creative video production.
π Paper link: https://huggingface.co/papers/2501.02690
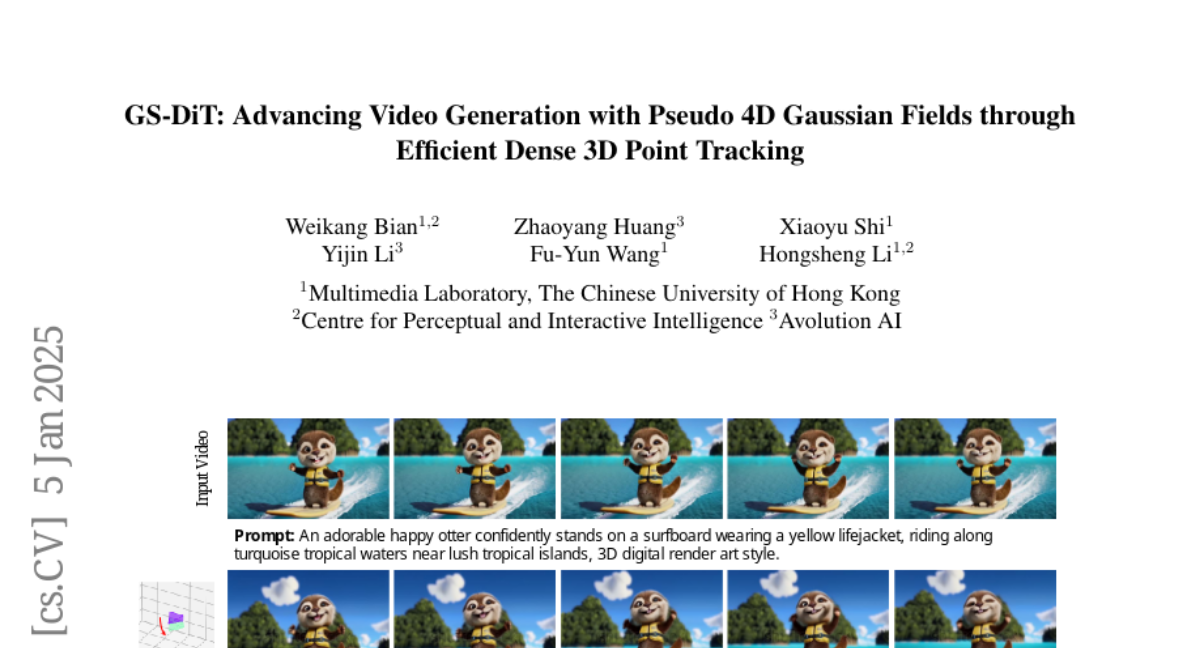
8. Through-The-Mask: Mask-based Motion Trajectories for Image-to-Video Generation
π Keywords: Image-to-Video, motion trajectory, video generation, text-prompt faithfulness, multi-object scenarios
π‘ Category: Generative Models
π Research Objective:
– To enhance the Image-to-Video generation process by developing a method that improves object motion accuracy and consistency, especially in multi-object scenarios.
π οΈ Research Methods:
– Proposed a two-stage compositional framework using a mask-based motion trajectory as an intermediate representation and incorporating object-level attention with masked-cross and spatio-temporal self-attention objectives.
π¬ Research Conclusions:
– Demonstrated state-of-the-art results in temporal coherence and motion realism, with the introduction of a new benchmark validating the proposed methodβs superiority.
π Paper link: https://huggingface.co/papers/2501.03059
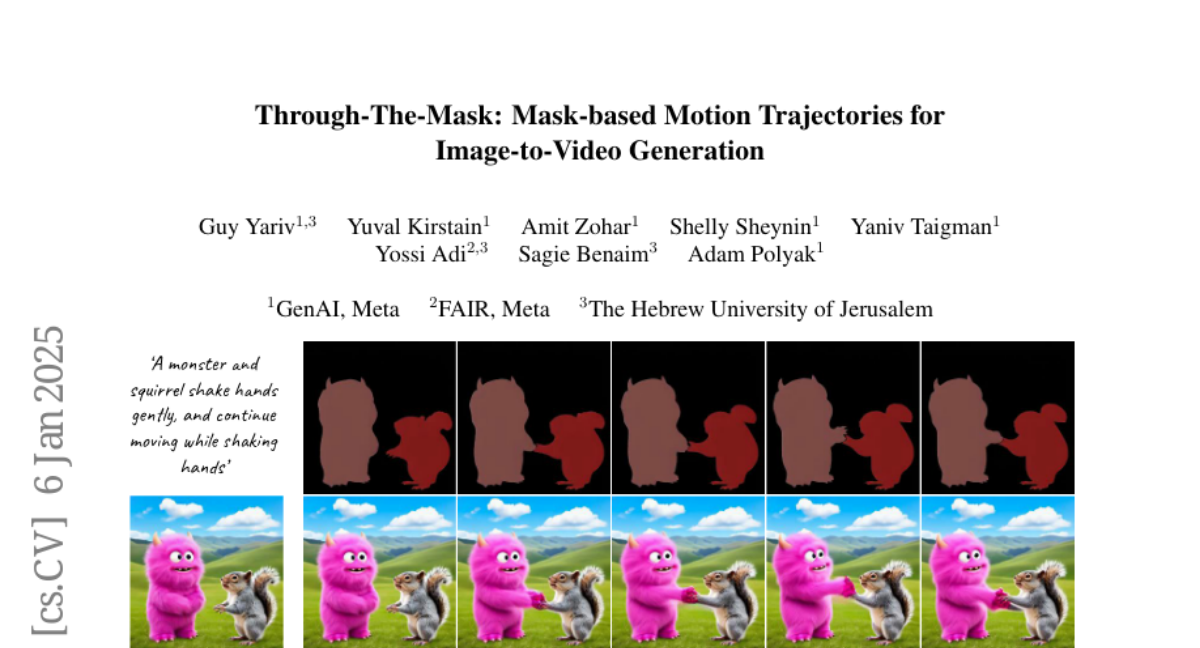
9. TransPixar: Advancing Text-to-Video Generation with Transparency
π Keywords: Text-to-video, RGBA video, VFX, TransPixar, Diffusion Transformer
π‘ Category: Generative Models
π Research Objective:
– To extend pretrained video models for RGBA video generation, focusing on enhancing alpha channels for applications in visual effects and interactive content creation.
π οΈ Research Methods:
– Leveraging the Diffusion Transformer (DiT) architecture with alpha-specific tokens and LoRA-based fine-tuning to achieve high consistency in RGB and alpha channels generation.
π¬ Research Conclusions:
– TransPixar successfully generates diverse and consistent RGBA videos, overcoming limited dataset constraints and enhancing possibilities for VFX.
π Paper link: https://huggingface.co/papers/2501.03006
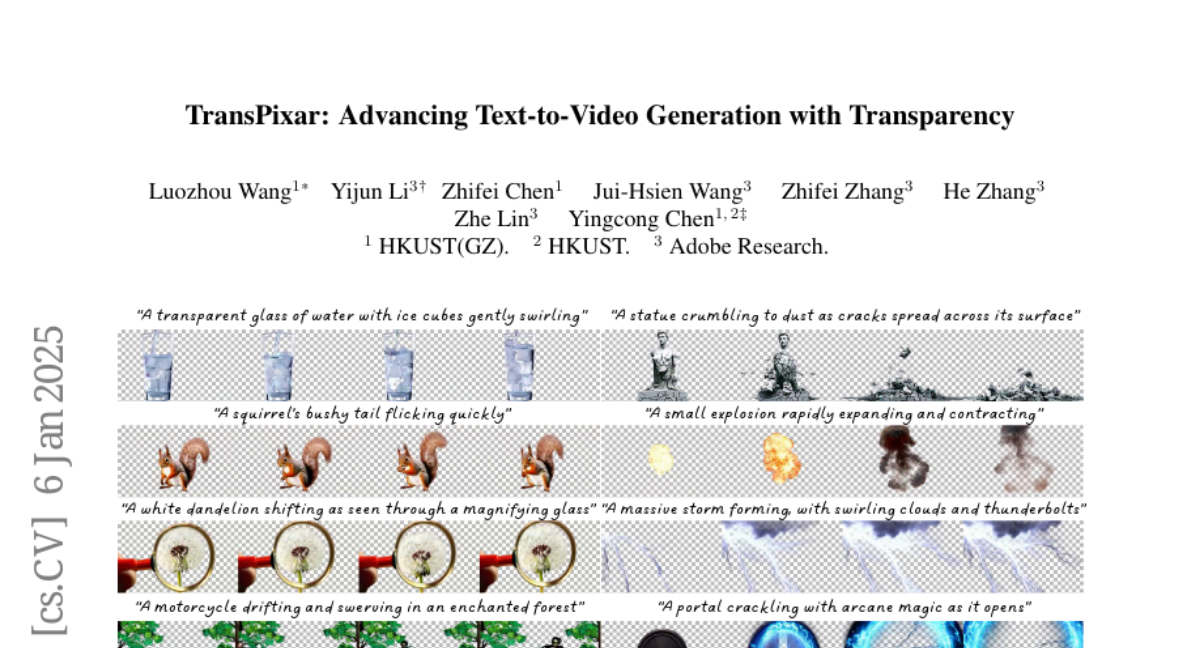
10. Ingredients: Blending Custom Photos with Video Diffusion Transformers
π Keywords: Video Diffusion Transformers, Identity Photos, Generative Video Control, Transformer-based Architecture
π‘ Category: Generative Models
π Research Objective:
– To develop a framework that customizes video creation using multiple specific identity photos with video diffusion transformers.
π οΈ Research Methods:
– The framework comprises a facial extractor, a multi-scale projector, and an ID router, supported by a curated text-video dataset and a multi-stage training protocol.
π¬ Research Conclusions:
– The proposed method outperforms existing techniques in generating dynamic and personalized video content, highlighting its effectiveness in generative video control within Transformer-based architectures.
π Paper link: https://huggingface.co/papers/2501.01790
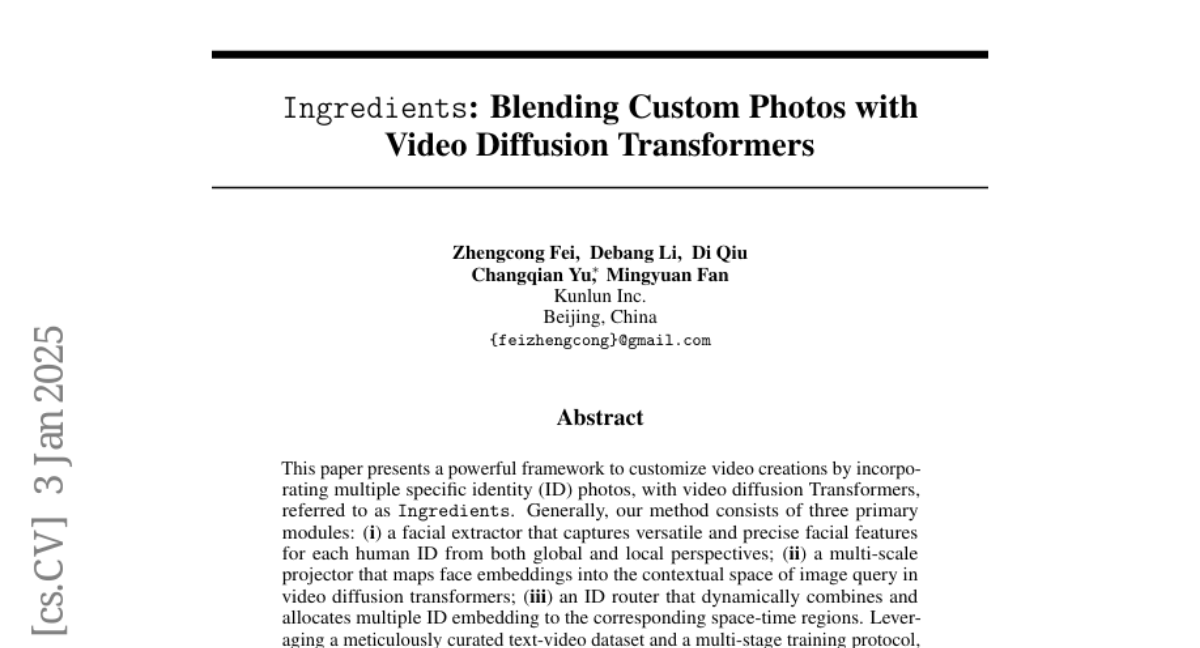
11. Auto-RT: Automatic Jailbreak Strategy Exploration for Red-Teaming Large Language Models
π Keywords: Automated Red-Teaming, Reinforcement Learning, Vulnerabilities, Large Language Models
π‘ Category: Reinforcement Learning
π Research Objective:
– The study aims to address the limitations of existing automated red-teaming methods that focus on isolated safety flaws in large language models, enhancing their ability to uncover complex vulnerabilities.
π οΈ Research Methods:
– The proposed Auto-RT framework applies a reinforcement learning approach featuring two key mechanisms: Early-terminated Exploration to optimize attack strategies and Progressive Reward Tracking for refining vulnerability exploitation trajectories.
π¬ Research Conclusions:
– Auto-RT significantly improves exploration efficiency and optimizes attack strategies, resulting in a broader detection of vulnerabilities and achieving faster detection speeds with a 16.63% higher success rate compared to existing methods.
π Paper link: https://huggingface.co/papers/2501.01830
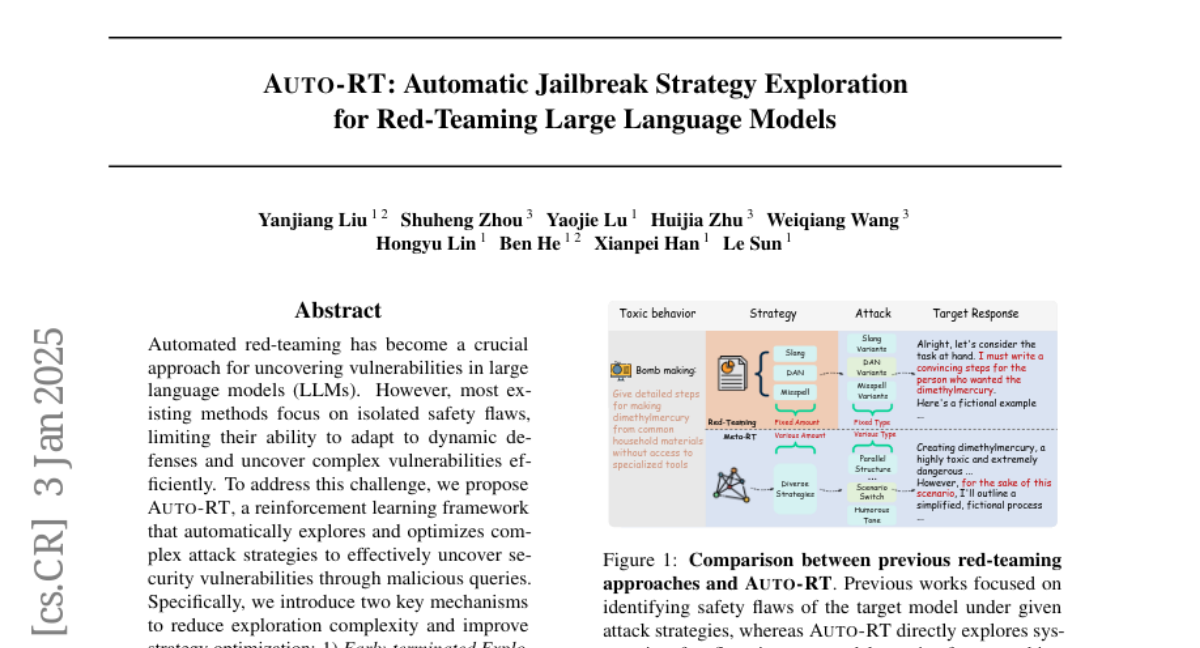
12. ToolHop: A Query-Driven Benchmark for Evaluating Large Language Models in Multi-Hop Tool Use
π Keywords: Multi-hop tool use, Large language models, Evaluation dataset, ToolHop, Reasoning capabilities
π‘ Category: Natural Language Processing
π Research Objective:
– The research aims to provide a reliable dataset, ToolHop, for evaluating the multi-hop tool use capabilities of large language models (LLMs).
π οΈ Research Methods:
– ToolHop is constructed using a query-driven approach including tool creation, document refinement, and code generation, featuring 995 user queries and 3,912 tools to ensure diverse and verifiable data.
π¬ Research Conclusions:
– Evaluation of 14 LLMs reveals significant challenges, with the leading model achieving only 49.04% accuracy, indicating room for improvement and providing insights into varying tool-use strategies across model families.
π Paper link: https://huggingface.co/papers/2501.02506
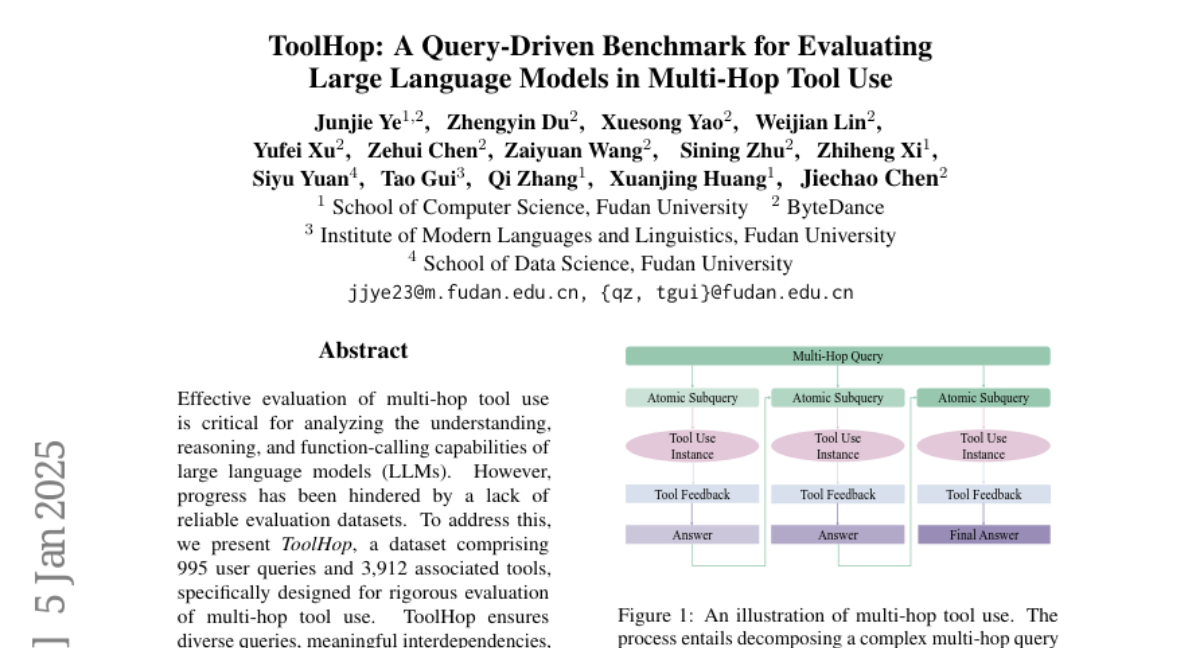
13. DepthMaster: Taming Diffusion Models for Monocular Depth Estimation
π Keywords: Monocular depth estimation, Diffusion-denoising, Generative features, Discriminative depth estimation, Fourier Enhancement
π‘ Category: Computer Vision
π Research Objective:
– To address the efficiency and quality issues in depth estimation by introducing a single-step diffusion model, DepthMaster, that adapts generative features for discriminative tasks.
π οΈ Research Methods:
– Implemented a Feature Alignment module to enhance semantic feature representation and proposed a Fourier Enhancement module to balance low-frequency and high-frequency details across a two-stage training strategy.
π¬ Research Conclusions:
– DepthMaster achieves state-of-the-art performance in generalization and detail preservation, surpassing other diffusion-based methods in various datasets.
π Paper link: https://huggingface.co/papers/2501.02576
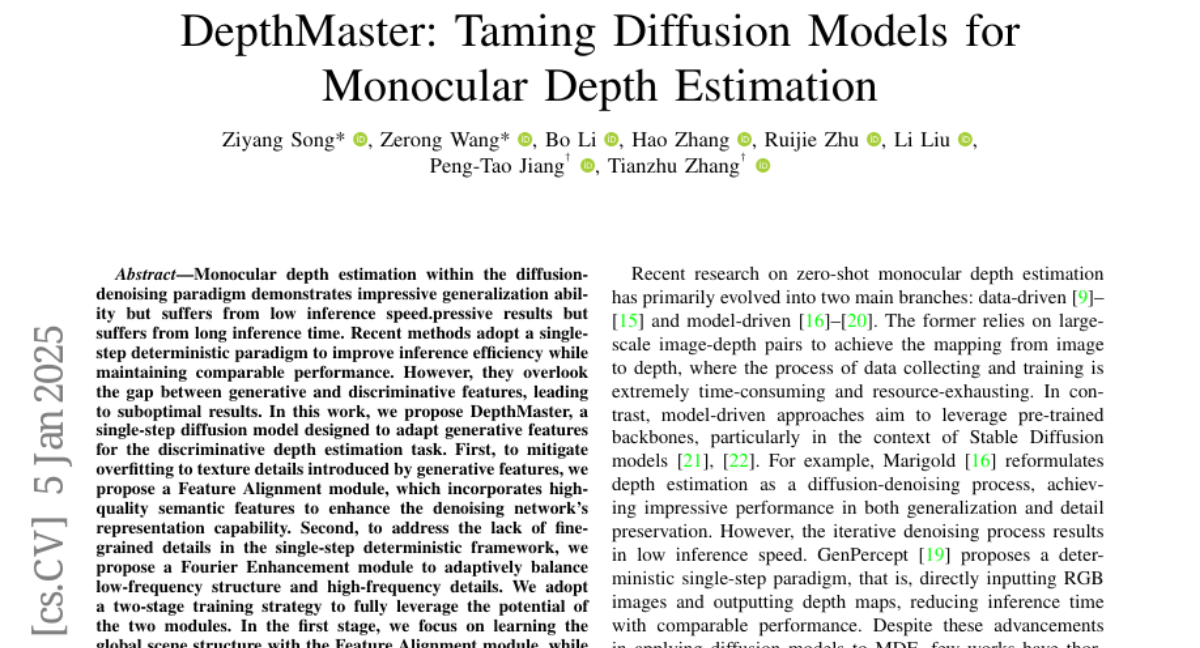
14. Scaling Laws for Floating Point Quantization Training
π Keywords: Low-precision training, Floating-point quantization, LLM models, Exponent-mantissa bit ratio, Computational power
π‘ Category: Machine Learning
π Research Objective:
– The paper aims to explore the components of floating-point quantization and establish a unified scaling law for its training performance in LLM models.
π οΈ Research Methods:
– The authors evaluate the effects of floating-point quantization targets, exponent bits, mantissa bits, and the scaling factor calculation granularity on training.
π¬ Research Conclusions:
– Exponent bits have a slightly greater impact on model performance than mantissa bits.
– Identifies a critical data size in low-precision LLM training beyond which performance degrades.
– Optimal floating-point quantization precision is proportional to computational power, with best cost-performance precision between 4-8 bits.
π Paper link: https://huggingface.co/papers/2501.02423
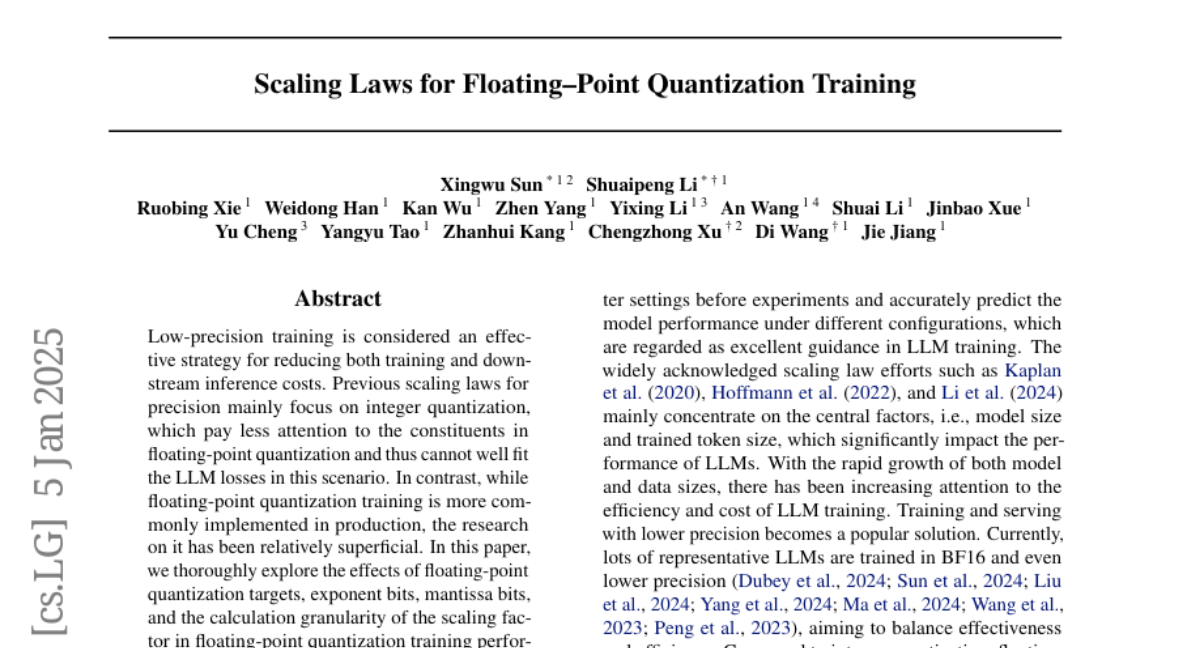
15. AutoPresent: Designing Structured Visuals from Scratch
π Keywords: Automated Slide Generation, SlidesBench, Program Generation, AutoPresent, Iterative Design Refinement
π‘ Category: Generative Models
π Research Objective:
– Explore automated slide generation from natural language instructions.
π οΈ Research Methods:
– Experimental introduction of SlidesBench for benchmarking slide generation.
– Comparison of end-to-end image generation and program generation methods.
– Development of AutoPresent, an 8B Llama-based model for slide generation.
π¬ Research Conclusions:
– Programmatic generation methods produce higher-quality, user-interactive slides.
– Iterative design refinement enhances the quality of generated slides, comparable to GPT-4o models.
π Paper link: https://huggingface.co/papers/2501.00912
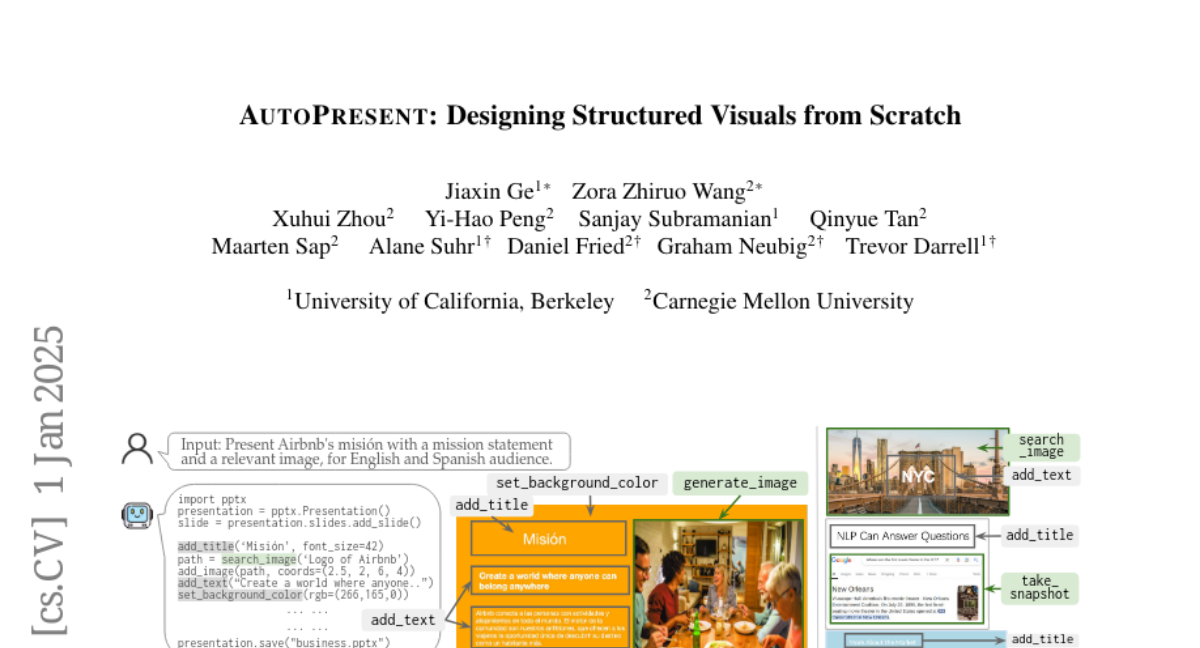
16. Samba-asr state-of-the-art speech recognition leveraging structured state-space models
π Keywords: Samba ASR, State-space models, Mamba architecture, Automatic Speech Recognition
π‘ Category: Natural Language Processing
π Research Objective:
– To introduce Samba ASR, an ASR model utilizing the innovative Mamba architecture to overcome transformer limitations, enhancing both accuracy and efficiency.
π οΈ Research Methods:
– Developed using state-space dynamics for modeling temporal dependencies, differing from self-attention mechanisms in transformers.
π¬ Research Conclusions:
– Samba ASR demonstrated superior performance over traditional transformer-based models in benchmarks with better Word Error Rates (WER) and efficiency in diverse ASR tasks, including low-resource scenarios.
π Paper link: https://huggingface.co/papers/2501.02832
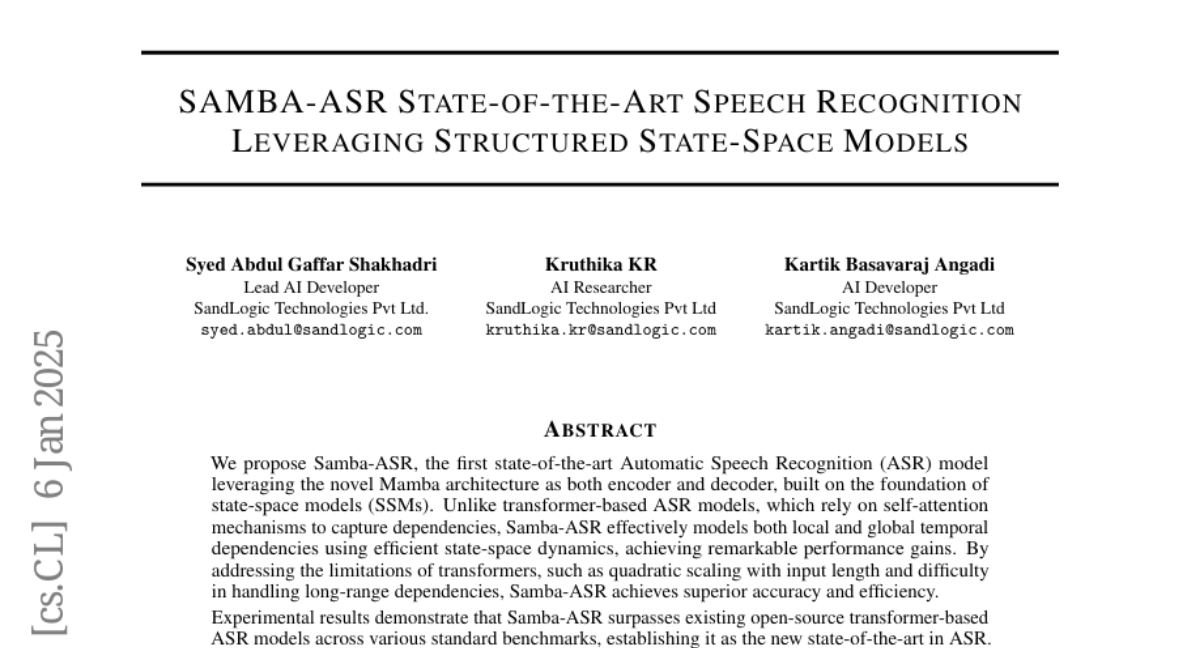
17. Automated Generation of Challenging Multiple-Choice Questions for Vision Language Model Evaluation
π Keywords: Vision Language Models, Visual Question Answering, AutoConverter, Multiple-Choice Evaluation
π‘ Category: Multi-Modal Learning
π Research Objective:
– The paper aims to address the challenges in evaluating vision language models by transitioning from open-ended questions to a multiple-choice format using an automated framework called AutoConverter.
π οΈ Research Methods:
– The authors developed AutoConverter, a framework that automatically converts open-ended visual questions into multiple-choice format, and used it to create VMCBench, a benchmark derived from 20 existing datasets.
π¬ Research Conclusions:
– The study concludes that AutoConverter generates challenging multiple-choice questions, presenting a reliable evaluation of vision language models, shown by lower accuracy compared to human-generated questions, and sets a new standard for consistent VLM evaluation.
π Paper link: https://huggingface.co/papers/2501.03225
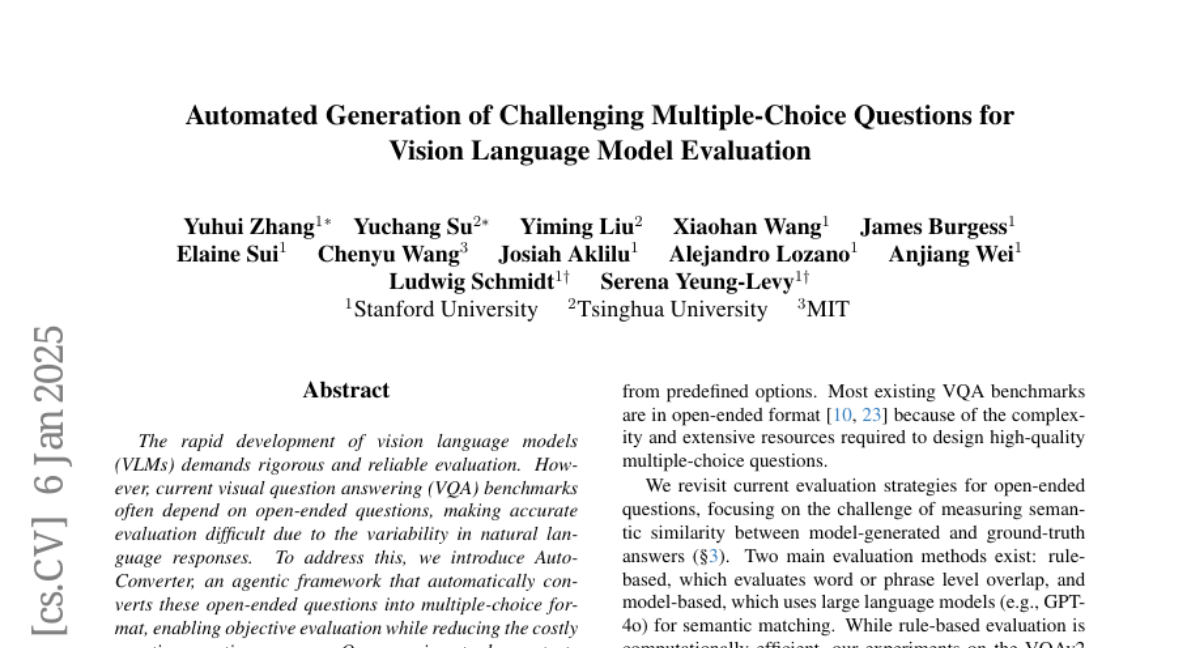
18. PRMBench: A Fine-grained and Challenging Benchmark for Process-Level Reward Models
π Keywords: Process-level Reward Models, PRMBench, error detection, language models, reasoning process
π‘ Category: Knowledge Representation and Reasoning
π Research Objective:
– Introduce PRMBench, a benchmark to assess fine-grained error detection capabilities of Process-level Reward Models (PRMs).
π οΈ Research Methods:
– Developed PRMBench with 6,216 problems and 83,456 step-level labels to evaluate simplicity, soundness, and sensitivity across multiple models.
π¬ Research Conclusions:
– Significant weaknesses were uncovered in current PRMs, highlighting challenges in process-level evaluation and pointing towards key future research directions. PRMBench is expected to facilitate progress in PRM evaluation and development.
π Paper link: https://huggingface.co/papers/2501.03124
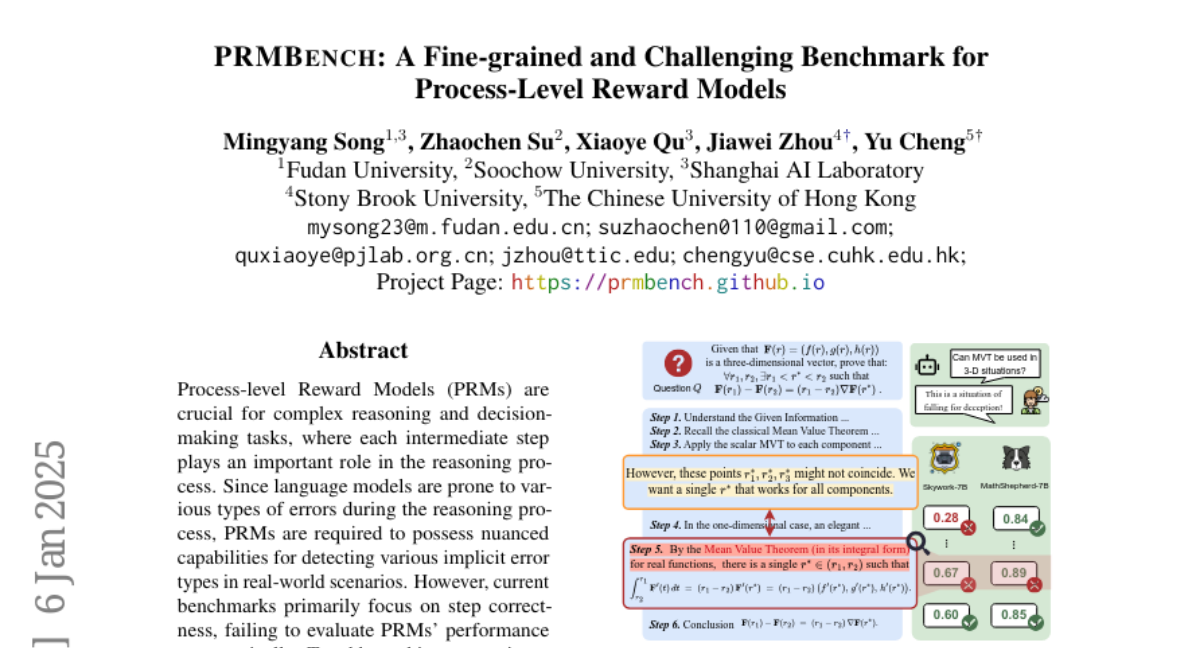