AI Native Daily Paper Digest – 20250319
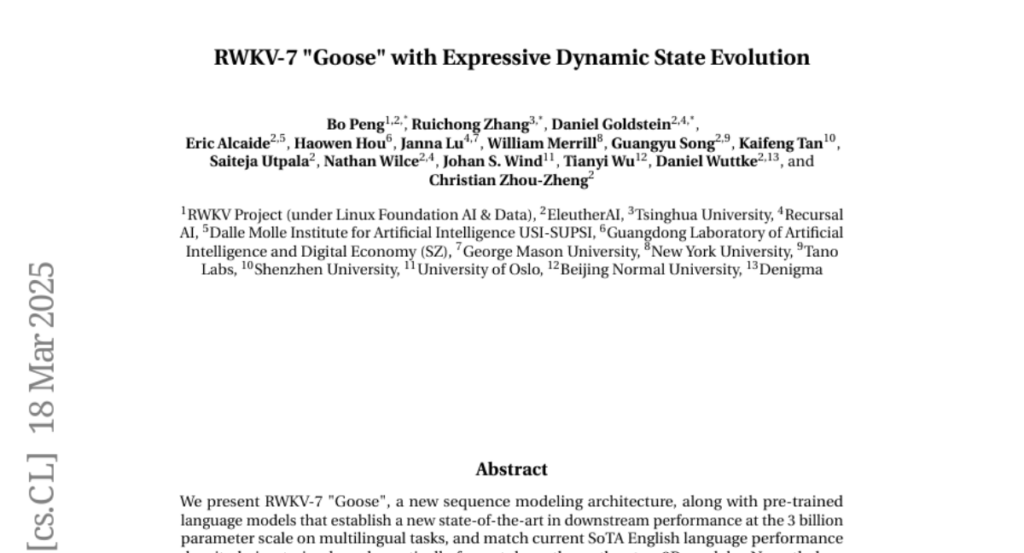
1. RWKV-7 “Goose” with Expressive Dynamic State Evolution
π Keywords: Collection
π‘ Category: Knowledge Representation and Reasoning
π Research Objective:
– To explore and enhance techniques for efficient and effective data collection in AI systems.
π οΈ Research Methods:
– Utilization of advanced algorithms to optimize the process of data aggregation and categorization.
π¬ Research Conclusions:
– Identified significant improvements in data processing efficiency, contributing to better outcomes in AI applications.
π Paper link: https://huggingface.co/papers/2503.14456
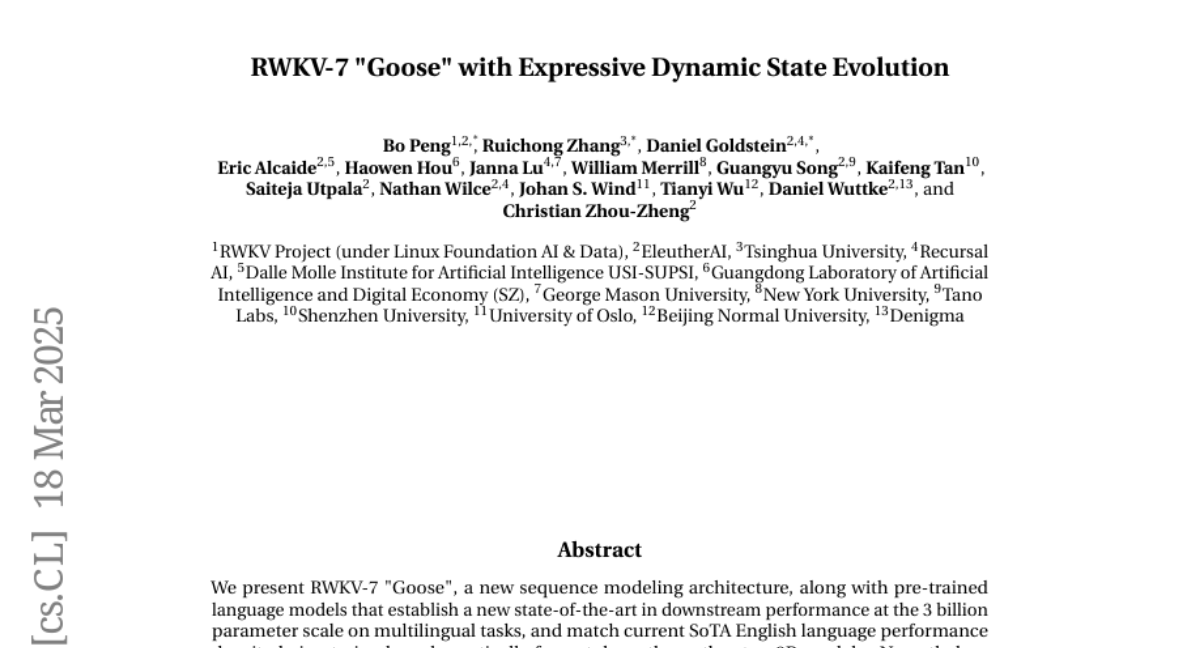
2. Impossible Videos
π Keywords: Collection
π‘ Category: Knowledge Representation and Reasoning
π Research Objective:
– Investigate the methodologies and mechanisms of data collection for improving AI systems.
π οΈ Research Methods:
– Analyze and utilize various techniques and tools essential for efficient data collection.
π¬ Research Conclusions:
– Offers insightful conclusions on how structured data collection enhances AI system performance and understanding.
π Paper link: https://huggingface.co/papers/2503.14378
3. DAPO: An Open-Source LLM Reinforcement Learning System at Scale
Collection
π Keywords: Collection, Data
π‘ Category: Foundations of AI
π Research Objective:
– To explore the concept of data collection in artificial intelligence and its fundamental implications for AI systems.
π οΈ Research Methods:
– Analyzing various data collection methodologies and examining their impact on AI model performance and reliability.
π¬ Research Conclusions:
– Data collection is crucial for the development and function of AI systems, affecting their efficiency and outcome accuracy.
π Paper link: https://huggingface.co/papers/2503.14476
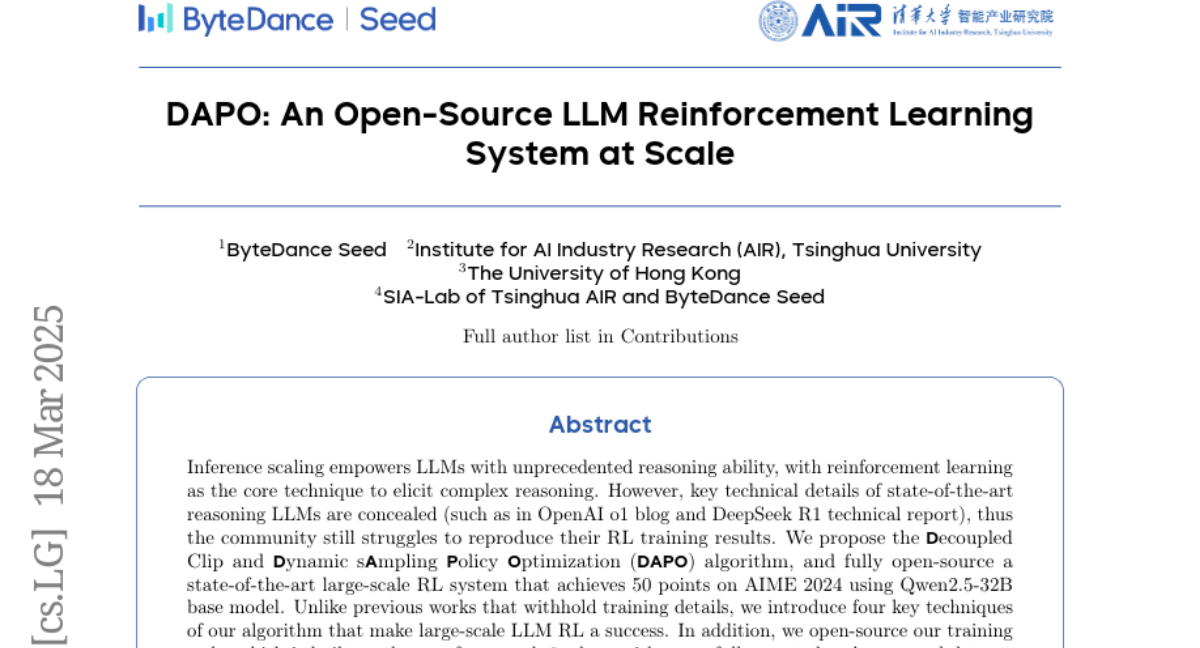
4. Creation-MMBench: Assessing Context-Aware Creative Intelligence in MLLM
π Keywords: Collection
π‘ Category: Foundations of AI
π Research Objective:
– The objective of the paper is focused on the study of collection mechanisms in AI systems.
π οΈ Research Methods:
– The research employs a comprehensive analysis of existing AI collection methods to enhance system efficiency.
π¬ Research Conclusions:
– The study concludes with insights that aim to optimize collection strategies, potentially impacting various AI applications.
π Paper link: https://huggingface.co/papers/2503.14478
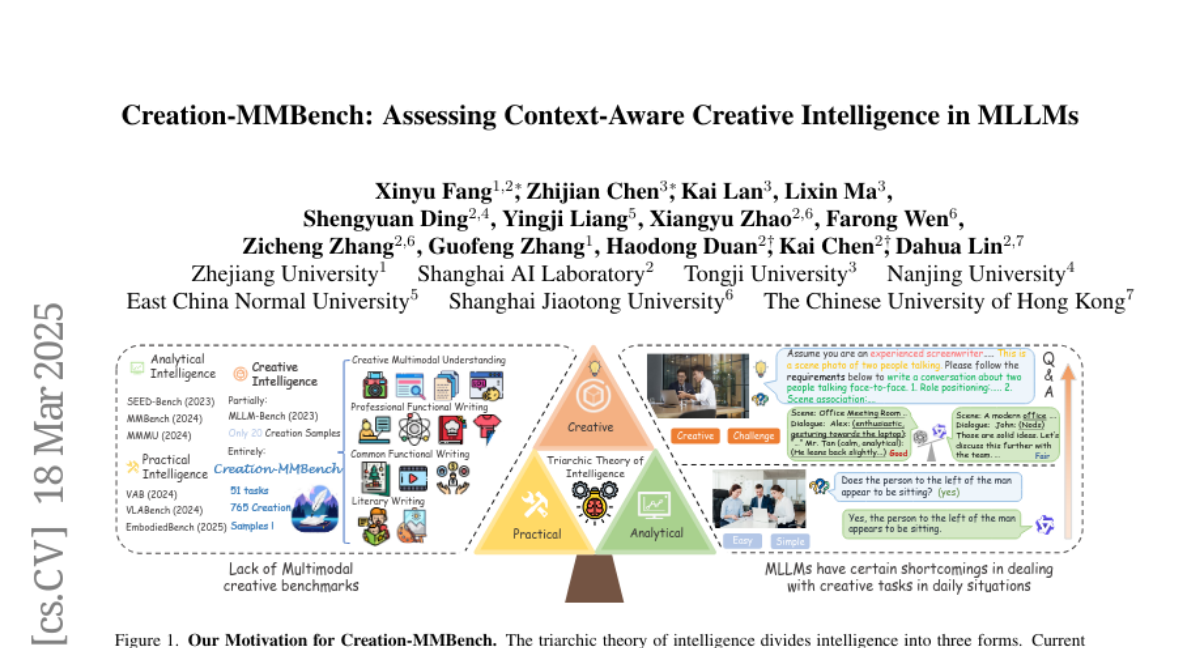
5. DeepPerception: Advancing R1-like Cognitive Visual Perception in MLLMs for Knowledge-Intensive Visual Grounding
π Keywords: Collection
π‘ Category: Foundations of AI
π Research Objective:
– The objective is to explore the fundamental aspects and elements related to Collection in the context of AI research.
π οΈ Research Methods:
– The research methods employed focus on theoretical and empirical studies, shedding light on the core principles surrounding Collection.
π¬ Research Conclusions:
– The conclusions offer insights into how Collection can be effectively understood and leveraged within various AI foundational studies.
π Paper link: https://huggingface.co/papers/2503.12797
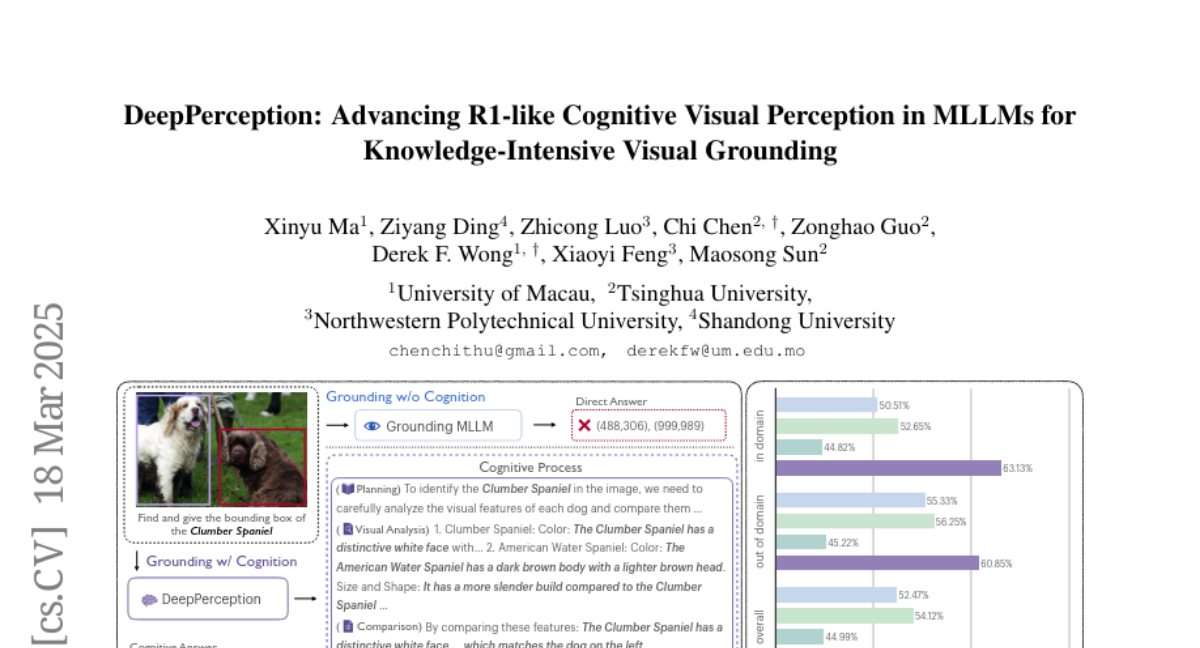
6. CapArena: Benchmarking and Analyzing Detailed Image Captioning in the LLM Era
π Keywords: Collection
π‘ Category: Foundations of AI
π Research Objective:
– To explore the concept and applications of Collection in AI systems.
π οΈ Research Methods:
– Analysis and synthesis of existing literature and case studies relevant to Collection.
π¬ Research Conclusions:
– The study provides insights into the implementation and impact of Collection in advancing AI capabilities.
π Paper link: https://huggingface.co/papers/2503.12329
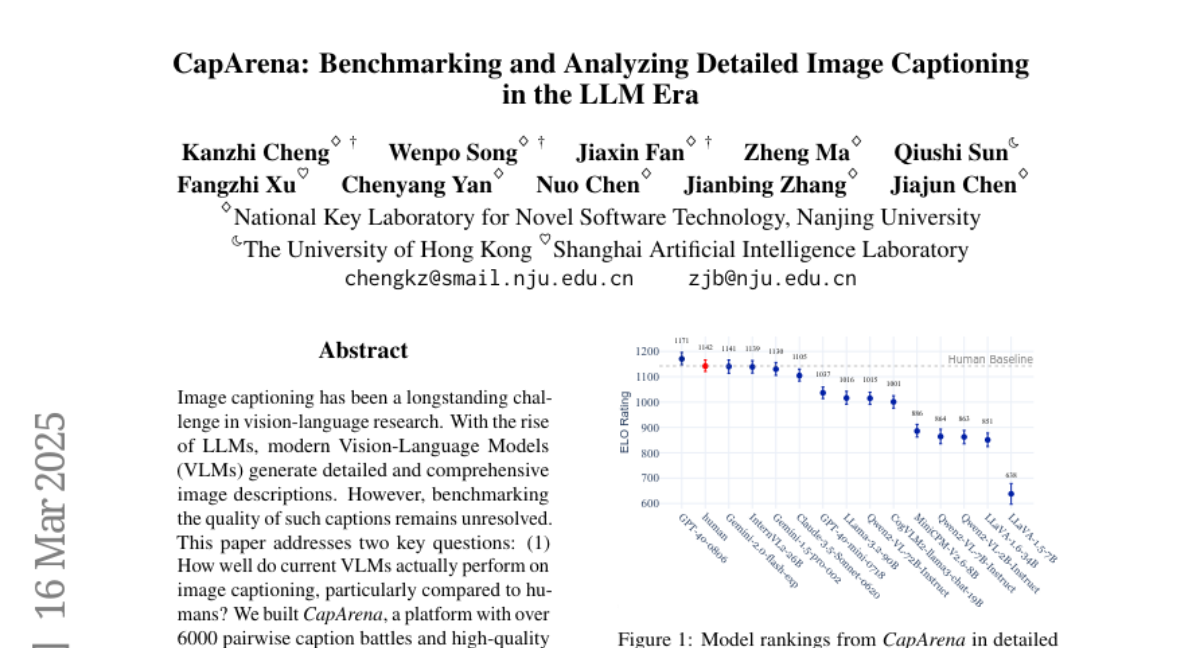
7. Infinite Mobility: Scalable High-Fidelity Synthesis of Articulated Objects via Procedural Generation
Collection
π Keywords: Collection, AI Ethics, Multi-Modal Learning
π‘ Category: Multi-Modal Learning
π Research Objective:
– Investigate the integration of diverse data sources to enhance AI model performance.
π οΈ Research Methods:
– Utilize multi-modal learning techniques to process and analyze data from various sources.
π¬ Research Conclusions:
– Demonstrates improved AI model accuracy and robustness when processing integrated data.
π Paper link: https://huggingface.co/papers/2503.13424
8. Frac-Connections: Fractional Extension of Hyper-Connections
π Keywords: Collection
π‘ Category: Foundations of AI
π Research Objective:
– To provide a comprehensive survey on the concept of Collection in AI.
π οΈ Research Methods:
– Collation and analysis of existing literature and methodologies related to Collection.
π¬ Research Conclusions:
– Identified key trends and future directions for research in Collection, offering a foundational understanding for further study in AI.
π Paper link: https://huggingface.co/papers/2503.14125
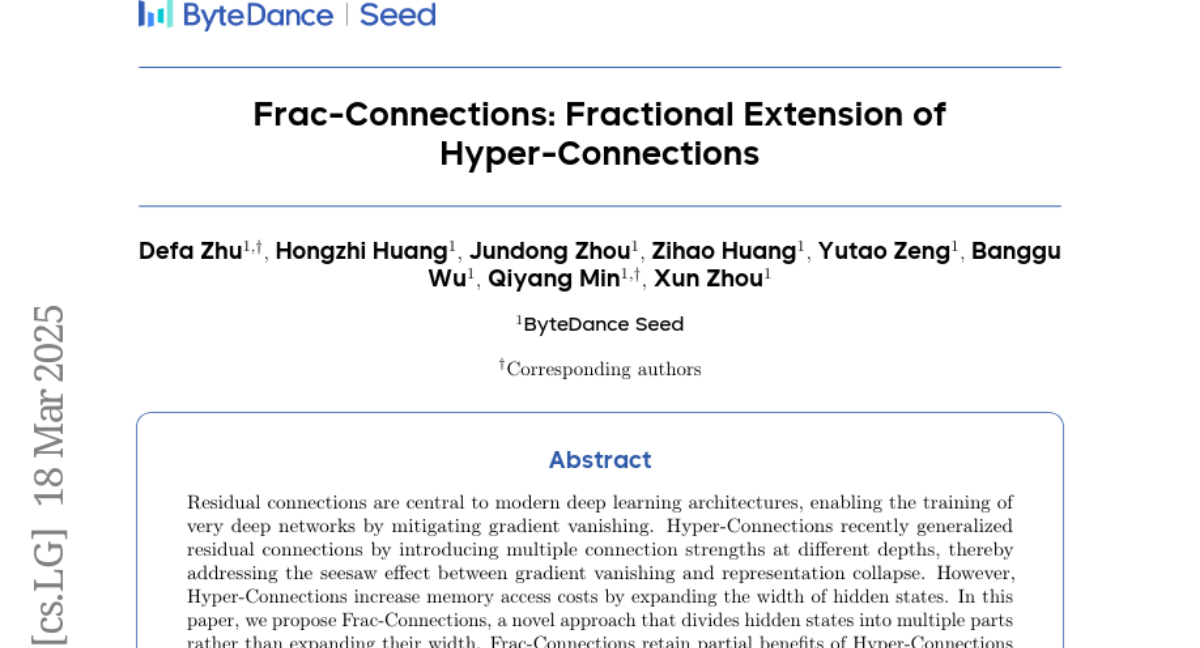
9. Cosmos-Transfer1: Conditional World Generation with Adaptive Multimodal Control
π Keywords: Collection
π‘ Category: AI Systems and Tools
π Research Objective:
– The paper investigates the concept of Collection within AI Systems, focusing on optimizing data aggregation processes.
π οΈ Research Methods:
– The study employs a range of computational algorithms designed to improve Collection efficiency in AI applications.
π¬ Research Conclusions:
– The findings suggest that the enhanced methods significantly boost data processing capacity, leading to more robust AI System outcomes.
π Paper link: https://huggingface.co/papers/2503.14492
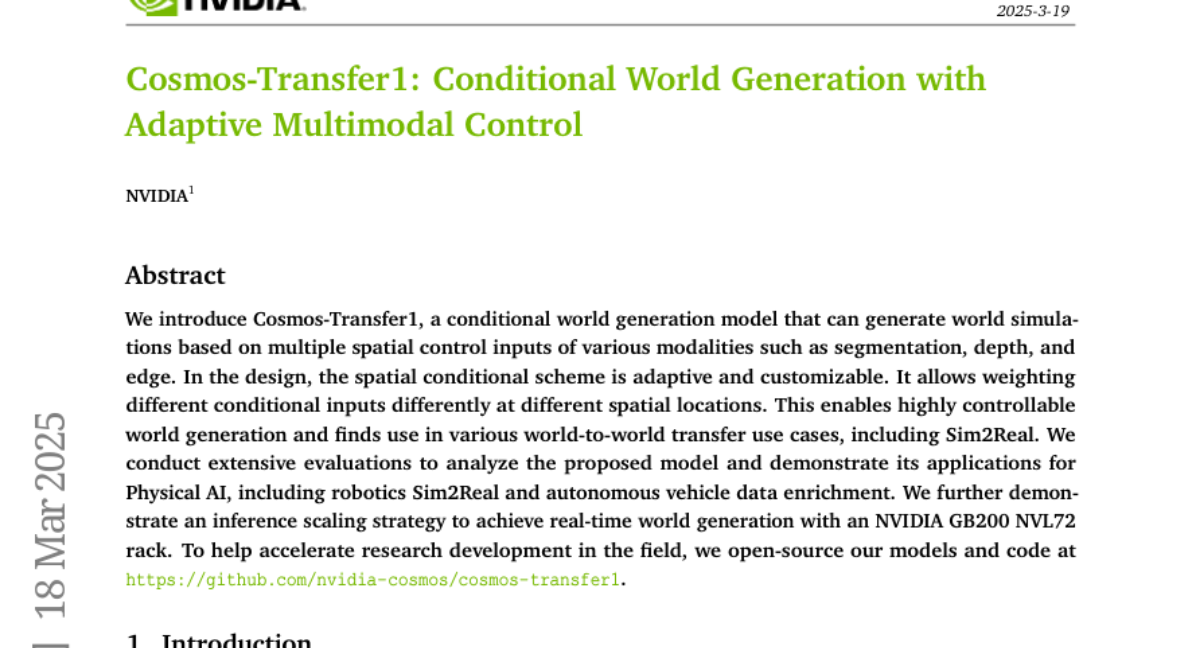
10. Aligning Multimodal LLM with Human Preference: A Survey
π Keywords: Collection
π‘ Category: AI Systems and Tools
π Research Objective:
– The primary aim is to explore the Collection mechanism and its impact on efficient data utilization in AI Systems.
π οΈ Research Methods:
– The study employs a systematic analysis of collection data structures, focusing on optimizing performance and scalability within AI frameworks.
π¬ Research Conclusions:
– The findings suggest that enhanced Collection techniques significantly improve processing efficiency, offering substantial benefits for AI system implementations.
π Paper link: https://huggingface.co/papers/2503.14504
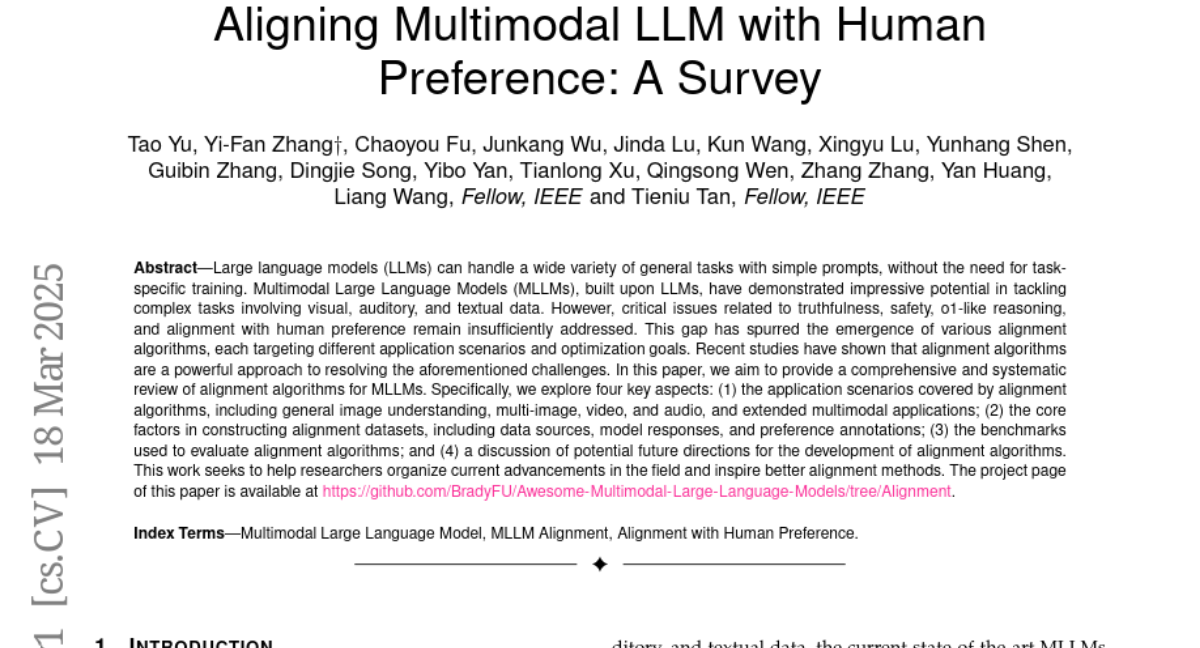