AI in Financial Spreading: Transforming Direct Lending Operations
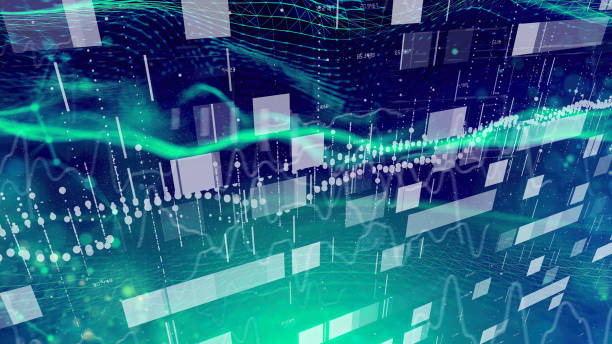
Image Credit | FinanceDerivative
This article is featured on FinanceDerivative and is written by Terence Tse, Bill McCahey and Danny Goh.
The Operational Challenge
Direct lending institutions face a critical bottleneck that constrains growth and profitability: financial spreading. This process—standardizing borrower financials for credit analysis—consumes disproportionate resources while creating delays in loan processing. For mid-sized lenders processing over 2,000 financial documents annually, traditional approaches require teams of analysts to manually review and enter data from diverse document formats, resulting in extended turnaround times, elevated costs, and inconsistent data quality.
Previous technological attempts to address this challenge through optical character recognition or robotic process automation have delivered disappointing returns on investment. These tools can read text but fail to understand context, often requiring as much human intervention as they were designed to eliminate—a frustrating outcome for organizations seeking efficiency.
The AI Solution: Capabilities and Business Impact
Today’s AI platforms represent a fundamental shift in approach, combining computer vision, natural language processing, and machine learning to transform financial spreading from an operational burden into a strategic advantage. The business impact is substantial and measurable across multiple dimensions.
From an operational perspective, leading lenders report processing time reductions from 3.2 hours to just 28 minutes per loan file—an 85% improvement. Error rates have declined by more than 70%, while document handling has become format-agnostic, allowing borrowers to submit information in whatever form they have available. When documentation is incomplete, AI systems immediately identify gaps, eliminating the costly back-and-forth that traditionally delays approvals.
For risk management, the advantages extend beyond efficiency. One direct lender discovered that AI-powered review identified significant financial discrepancies in 43 out of 500 loan files that human analysts had previously cleared—representing potential problem loans with real economic implications. Modern systems automatically calculate and benchmark financial ratios against industry standards while flagging concerning trends in borrower statements, ensuring consistent application of credit standards across all applications.
The revenue impact is equally compelling. A construction-focused lender increased loan volume by 62% without proportional staff growth after implementing AI-powered processing. This scalability allows institutions to pursue market opportunities without the traditional constraint of processing capacity, while faster turnaround times improve both the borrower experience and competitive positioning. Perhaps most importantly, by automating routine data extraction, lenders can redirect analyst talent toward relationship development and complex deal structuring—activities that directly drive new business acquisition.
Implementation Framework for Maximum Return
Business leaders considering AI implementation should focus on five key factors to maximize return on investment. First, integration with existing infrastructure is essential—systems must create seamless data flow between AI platforms and current loan origination systems to eliminate duplicate work and realize full efficiency gains.
Second, customization for specific business segments ensures the AI understands industry-specific financial presentations relevant to your lending portfolio. A tech startup’s financials differ substantially from those of a construction firm, and the system must accommodate these variations to deliver accurate results.
Third, robust security and compliance architecture with bank-grade encryption and comprehensive audit trails maintains regulatory compliance while protecting sensitive borrower information—a non-negotiable requirement in the lending industry.
Fourth, a thoughtful change management strategy develops clear analyst workflows that leverage AI for routine tasks while emphasizing human judgment for complex decisions. The most successful implementations position AI as an analyst enhancement rather than replacement.
Finally, establishing clear performance metrics for processing time, error rates, and analyst productivity creates accountability and quantifies the return on technology investment.
Strategic Advantages Beyond Cost Reduction
Forward-thinking lenders are leveraging AI not merely for efficiency but as a competitive differentiator. With streamlined processing capabilities, institutions can profitably serve smaller loan segments previously considered unprofitable due to operational costs. Advanced AI models identify borrower performance patterns, enabling proactive portfolio management and targeted cross-selling opportunities.
Enhanced borrower experiences represent another strategic advantage. Modern systems offer multi-channel assistance through text, voice, or video chat, providing tailored industry-specific guidance and interactive dashboards that display application status, document quality scores, and improvement recommendations.
Looking ahead, emerging AI technologies will further transform lending through predictive cash flow modeling incorporating real-time market trends, conversational interfaces for borrower communication, alternative data integration, and automated fraud detection. These capabilities will enable lenders to make faster, more personalized decisions at scale.
The financial spreading process hasn’t disappeared, but its transformation through AI creates a foundation for precision lending that delivers superior results for both institutions and borrowers. As competition intensifies, those who embrace this technology will position themselves to lead the market through operational excellence and enhanced decision-making.
Terence Tse is Professor Finance, Hult International Business School and cofounder and Chair in Fintech & Business AI at The Chart ThinkTank
Bill McCahey is Capital Markets Technology Leader, Client Partner at Infosys
Danny Goh is CEO of Nexus FrontierTech and is cofounder and Chair in Frontier Technology & Innovation Ecosystems at The Chart ThinkTank