AI Native Daily Paper Digest – 20250422
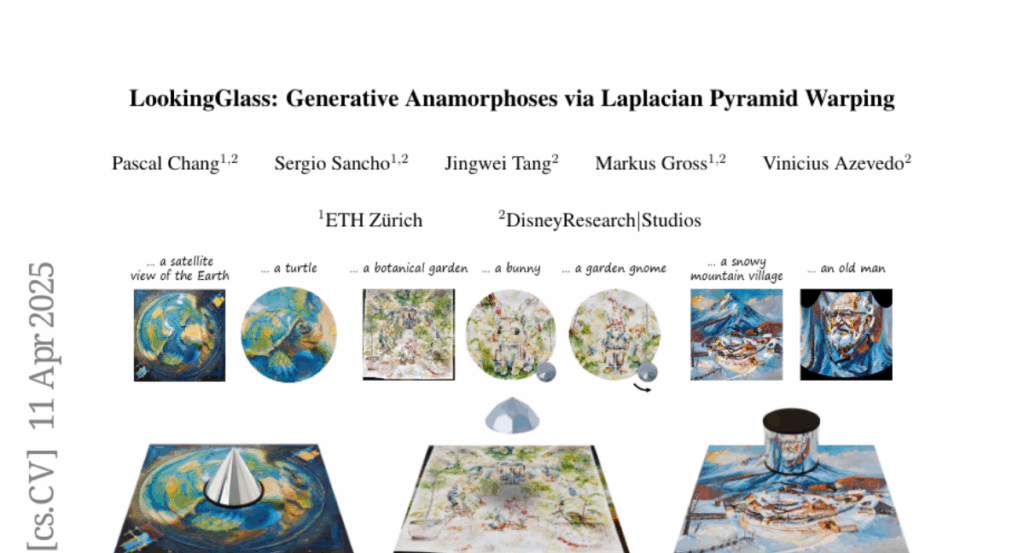
1. Learning to Reason under Off-Policy Guidance
๐ Keywords: Large Reasoning Models (LRMs), Reinforcement Learning (RL), Zero-RL, Off-Policy, LUFFY
๐ก Category: Reinforcement Learning
๐ Research Objective:
– This paper introduces LUFFY, a framework aiming to enhance zero-RL approaches by integrating off-policy reasoning traces to overcome limitations of on-policy learning.
๐ ๏ธ Research Methods:
– LUFFY dynamically mixes off-policy demonstrations with on-policy rollouts. It employs policy shaping through regularized importance sampling to balance imitation and exploration effectively during training.
๐ฌ Research Conclusions:
– LUFFY demonstrates significant improvement, with a +7.0 average gain on math benchmarks and +6.2 points in out-of-distribution tasks, offering a scalable solution for training generalizable reasoning models.
๐ Paper link: https://huggingface.co/papers/2504.14945
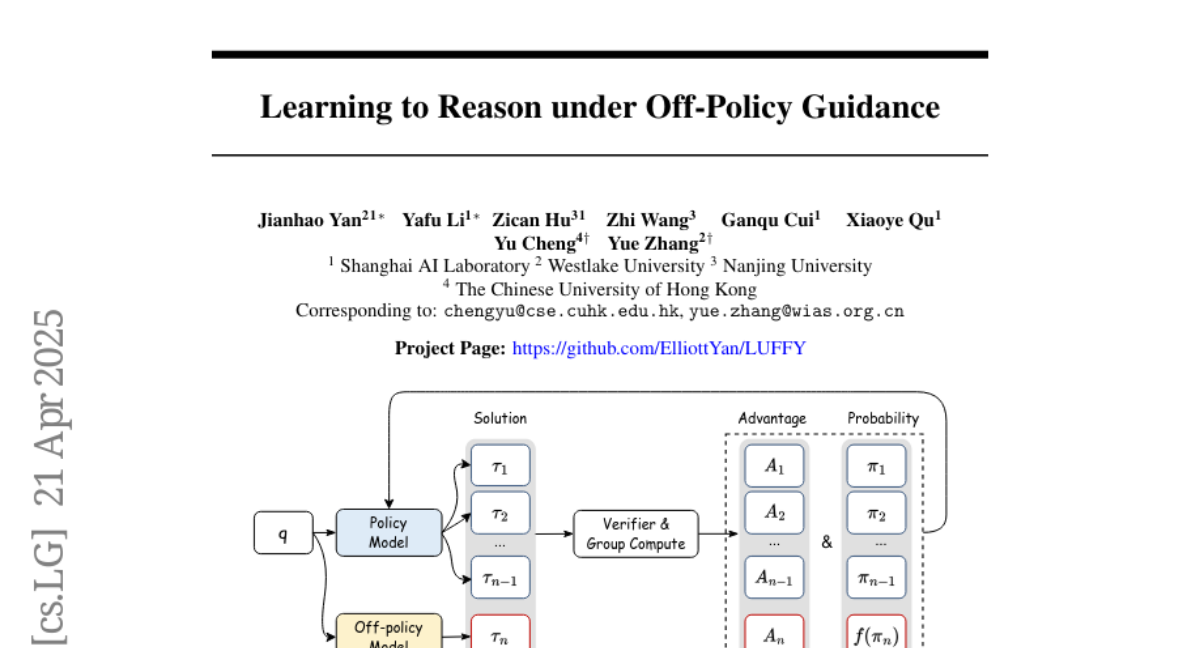
2. Eagle 2.5: Boosting Long-Context Post-Training for Frontier Vision-Language Models
๐ Keywords: Vision-Language Models (VLMs), Long-Context Multimodal Learning, High-Resolution Image Understanding, Automatic Degrade Sampling, Eagle-Video-110K
๐ก Category: Multi-Modal Learning
๐ Research Objective:
– To develop Eagle 2.5, a family of frontier VLMs capable of addressing the challenges in long video comprehension and high-resolution image understanding.
๐ ๏ธ Research Methods:
– Introduced a training framework incorporating Automatic Degrade Sampling and Image Area Preservation to maintain contextual integrity and visual details, along with various efficiency optimizations for long-context data training.
๐ฌ Research Conclusions:
– Eagle 2.5 exhibits significant improvements in long-context multimodal benchmarks, achieving similar performance to leading models such as GPT-4o and other large-scale open-source models.
๐ Paper link: https://huggingface.co/papers/2504.15271
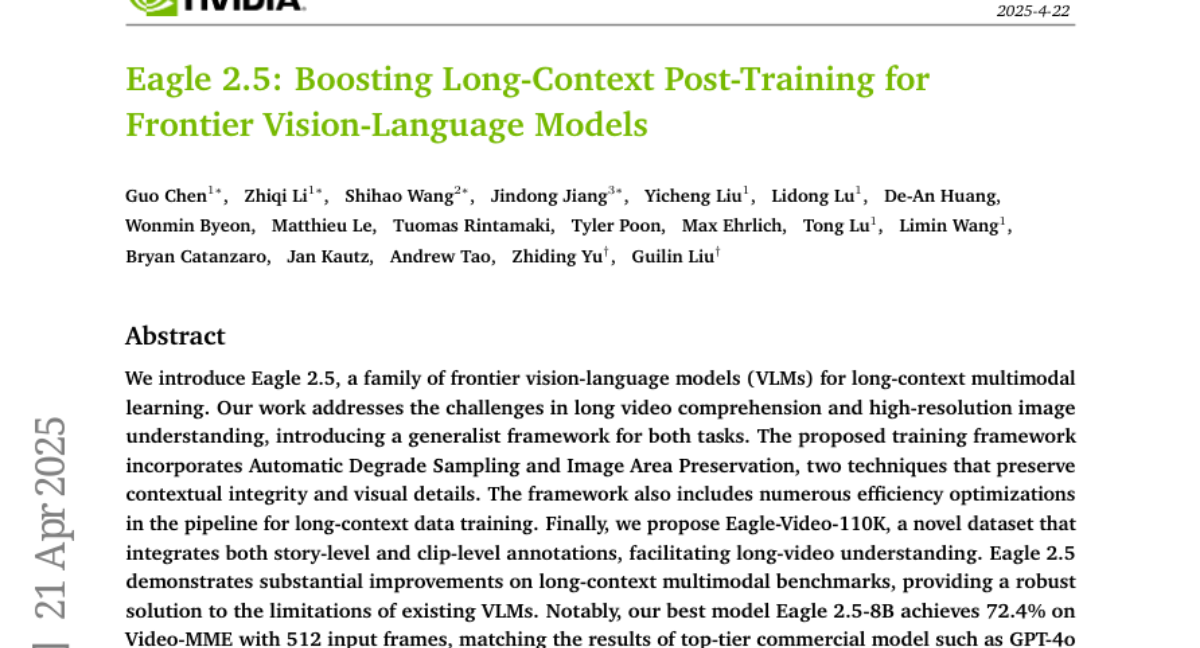
3. FlowReasoner: Reinforcing Query-Level Meta-Agents
๐ Keywords: Meta-agent, Multi-agent systems, Reinforcement Learning, DeepSeek R1, Deliberative reasoning
๐ก Category: AI Systems and Tools
๐ Research Objective:
– The research aims to automate the design of query-level multi-agent systems, creating one system per user query through a meta-agent approach named FlowReasoner.
๐ ๏ธ Research Methods:
– The study employs DeepSeek R1 to instill basic reasoning abilities in FlowReasoner and further enhances it using reinforcement learning with external execution feedback. Additionally, a multi-purpose reward is designed to guide the training process focusing on performance, complexity, and efficiency.
๐ฌ Research Conclusions:
– Experiments demonstrate FlowReasoner’s superiority over existing models, achieving a 10.52% higher accuracy than o1-mini across three engineering and competition code benchmarks. The code is available at the provided GitHub link.
๐ Paper link: https://huggingface.co/papers/2504.15257
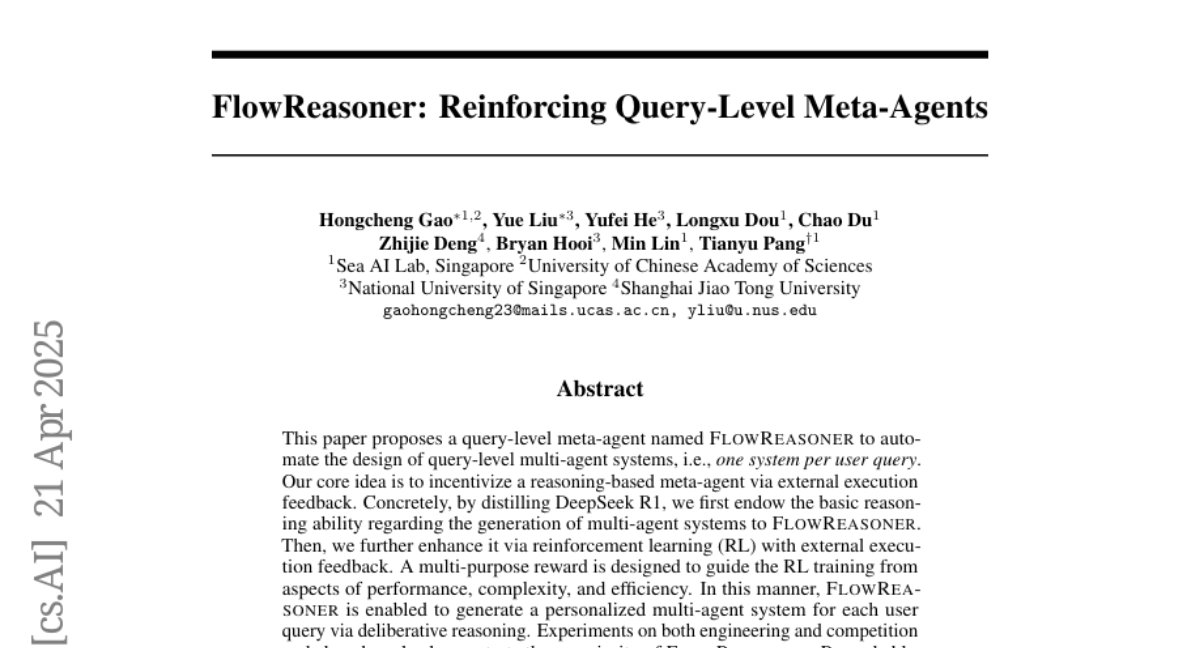
4. ToolRL: Reward is All Tool Learning Needs
๐ Keywords: Large Language Models, Supervised Fine-Tuning, Reinforcement Learning, Reward Design, Group Relative Policy Optimization
๐ก Category: Reinforcement Learning
๐ Research Objective:
– To conduct a comprehensive study on reward design for tool use tasks within the reinforcement learning framework.
๐ ๏ธ Research Methods:
– Systematic exploration of various reward strategies with a focus on types, scales, granularity, and temporal dynamics.
– Implementation of a principled reward design in training LLMs using Group Relative Policy Optimization (GRPO).
๐ฌ Research Conclusions:
– The proposed approach improves training stability and scalability, achieving a 17% performance increase over base models and a 15% improvement over SFT models.
– The study emphasizes the crucial role of detailed reward design in enhancing tool use capabilities and generalization of LLMs.
๐ Paper link: https://huggingface.co/papers/2504.13958
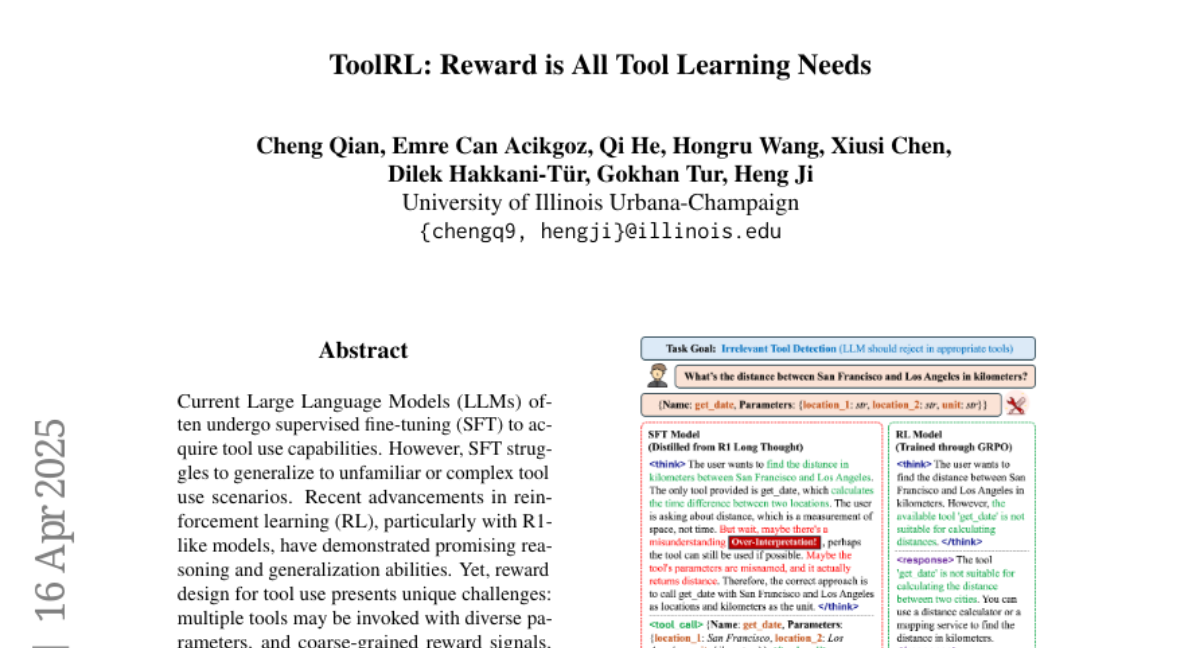
5. X-Teaming: Multi-Turn Jailbreaks and Defenses with Adaptive Multi-Agents
๐ Keywords: Multi-turn interactions, Language models, X-Teaming, Attack scenarios, Safety alignment
๐ก Category: Natural Language Processing
๐ Research Objective:
– The paper aims to address safety risks in multi-turn interactions with language models by introducing a scalable framework called X-Teaming.
๐ ๏ธ Research Methods:
– X-Teaming employs collaborative agents for planning, attack optimization, and verification to achieve effective and diverse multi-turn jailbreak scenarios.
๐ฌ Research Conclusions:
– X-Teaming demonstrates high success rates, achieving up to 98.1% across various models, and a 96.2% success rate against the Claude 3.7 Sonnet model. Additionally, the introduction of XGuard-Train provides a comprehensive dataset for enhancing multi-turn safety in LMs.
๐ Paper link: https://huggingface.co/papers/2504.13203
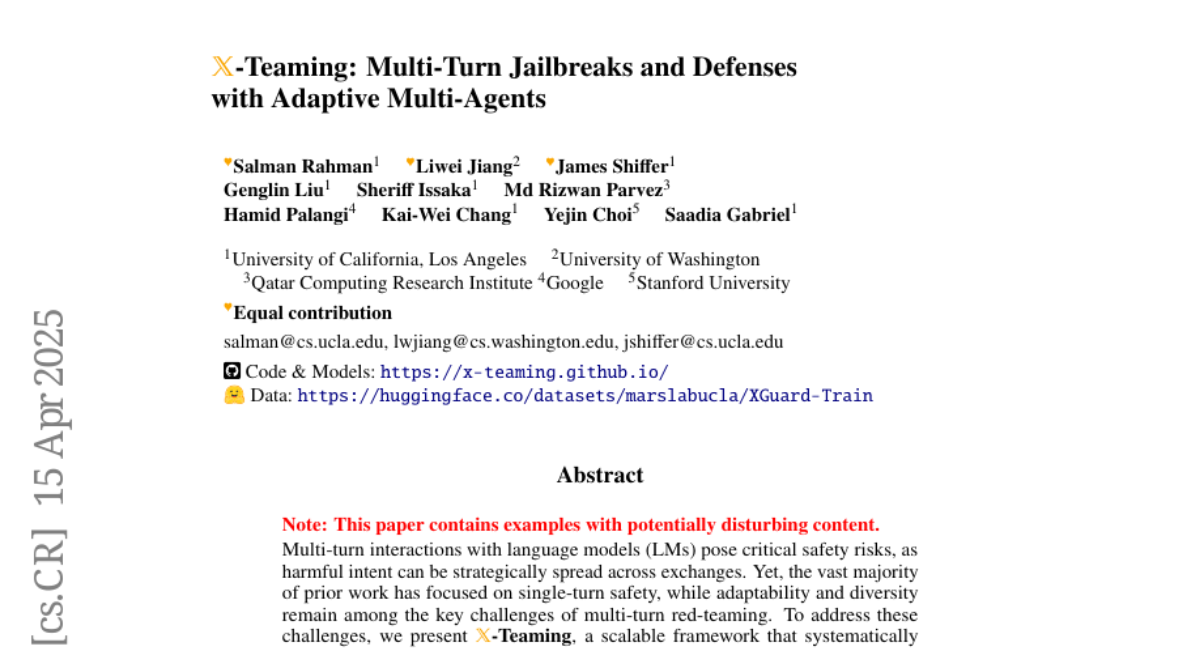
6. SphereDiff: Tuning-free Omnidirectional Panoramic Image and Video Generation via Spherical Latent Representation
๐ Keywords: SphereDiff, 360-degree panoramic, Diffusion models, Spherical latent representation, Immersive AR/VR applications
๐ก Category: Generative Models
๐ Research Objective:
– To introduce a novel approach called SphereDiff for seamless generation of high-quality 360-degree panoramic images and videos using diffusion models.
๐ ๏ธ Research Methods:
– Defined a spherical latent representation to ensure uniform distribution, mitigating ERP distortions.
– Extended MultiDiffusion into spherical latent space and proposed a spherical latent sampling method.
– Introduced distortion-aware weighted averaging to enhance generation quality.
๐ฌ Research Conclusions:
– SphereDiff outperforms existing methods in generating 360-degree panoramic content, maintaining high fidelity, and provides a robust solution for immersive AR/VR applications.
๐ Paper link: https://huggingface.co/papers/2504.14396
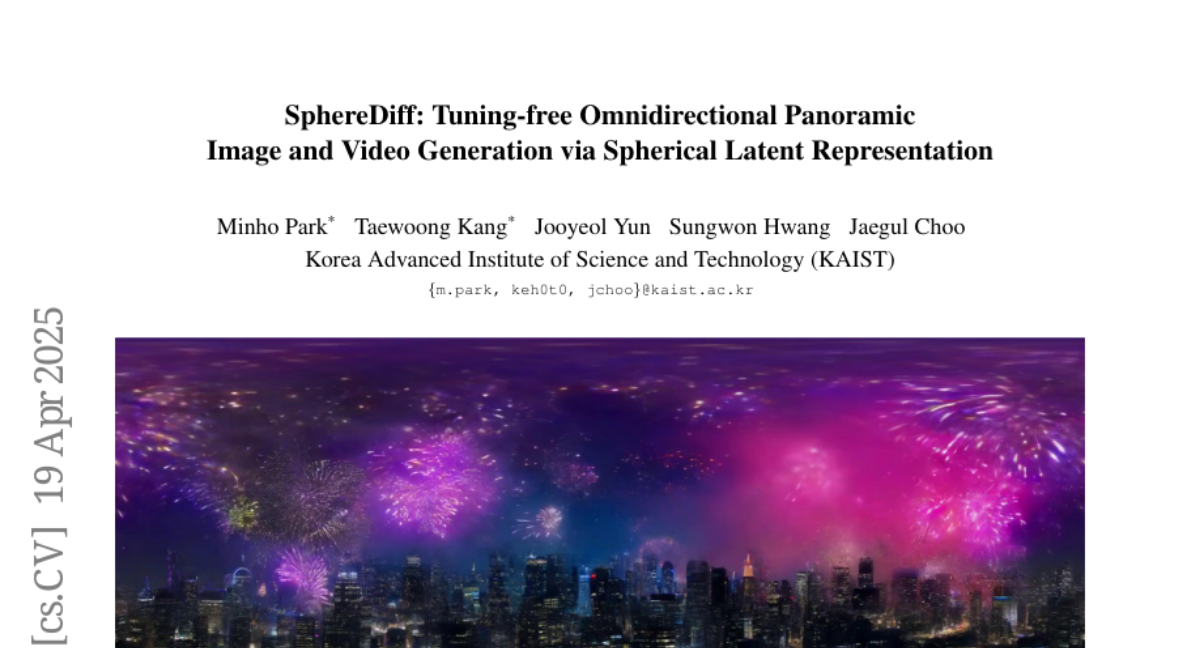
7. UFO2: The Desktop AgentOS
๐ Keywords: CUAs, multimodal large language models, native APIs, task decomposition, OS integration
๐ก Category: AI Systems and Tools
๐ Research Objective:
– The study aims to enhance CUAs by improving desktop automation through deep OS integration and advanced multiagent architectures.
๐ ๏ธ Research Methods:
– Developed UFO2, which features a centralized HostAgent for task coordination and multiple AppAgents with native APIs, utilizing a hybrid control detection pipeline and speculative multi-action planning for efficiency.
๐ฌ Research Conclusions:
– UFO2 demonstrates significant improvements in robustness and execution accuracy, offering a scalable path for reliable desktop automation through enhanced OS integration.
๐ Paper link: https://huggingface.co/papers/2504.14603
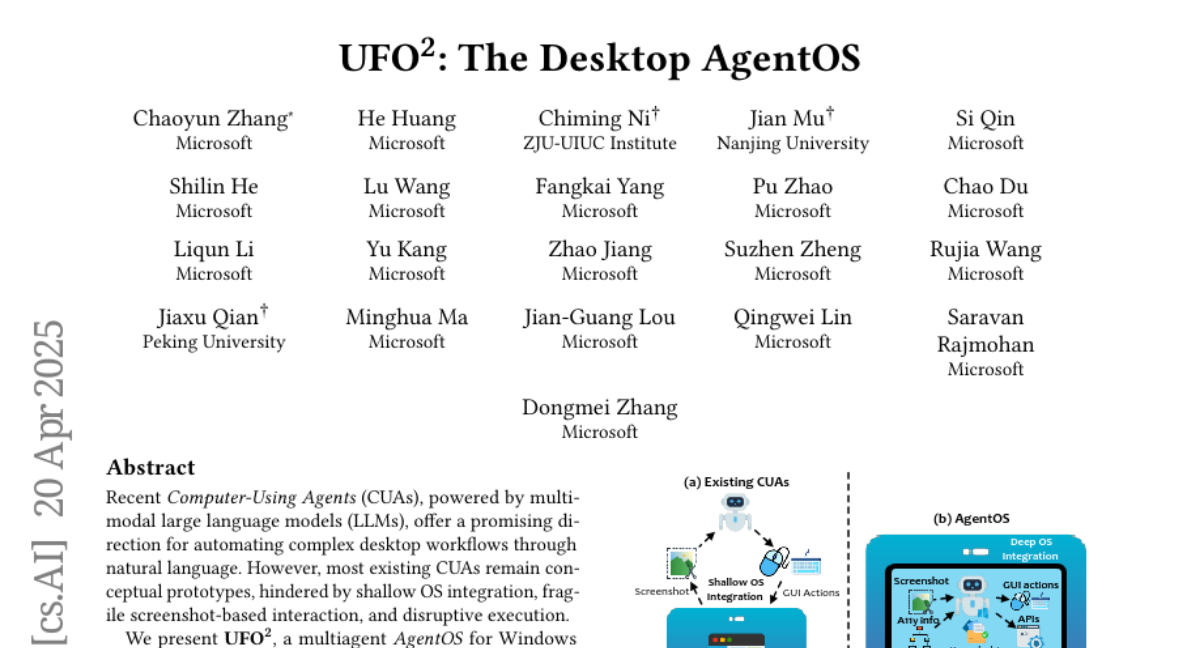
8. OTC: Optimal Tool Calls via Reinforcement Learning
๐ Keywords: Tool-integrated reasoning, large language models, reinforcement learning, efficiency, tool calls
๐ก Category: Reinforcement Learning
๐ Research Objective:
– To develop a framework, OTC-PO, that enhances the accuracy and efficiency of Tool-integrated reasoning in large language models by minimizing unnecessary tool calls.
๐ ๏ธ Research Methods:
– Introduction of an RL-based framework, Optimized Tool Call-controlled Policy Optimization (OTC-PO), incorporating Proximal Policy Optimization (PPO) and Group Relative Preference Optimization (GRPO).
– Utilization of a tool-integrated reward system that balances correctness and tool efficiency.
๐ฌ Research Conclusions:
– The proposed framework OTC-PPO and OTC-GRPO significantly reduce tool calls by up to 73.1% while enhancing tool productivity by up to 229.4%, without sacrificing accuracy.
– Marks the first RL-based approach focusing on optimizing tool-use efficiency in tool-integrated reasoning.
๐ Paper link: https://huggingface.co/papers/2504.14870
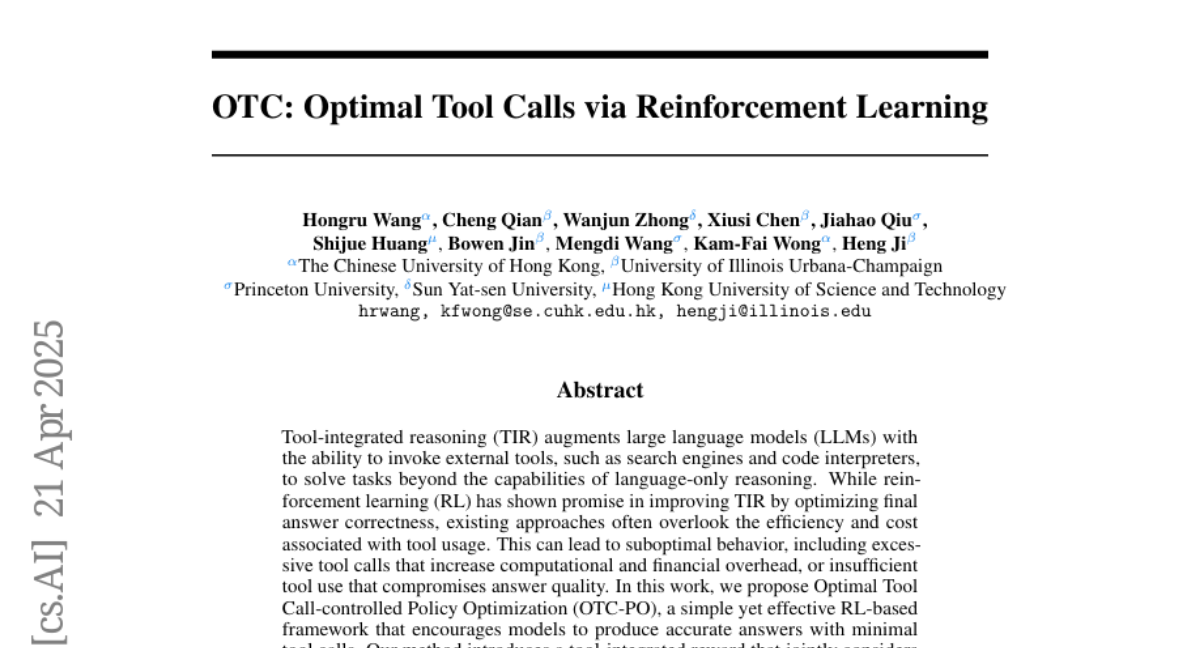
9. StyleMe3D: Stylization with Disentangled Priors by Multiple Encoders on 3D Gaussians
๐ Keywords: 3D Gaussian Splatting, StyleMe3D, multi-level semantic alignment, perceptual quality enhancement, Dynamic Style Score Distillation
๐ก Category: Generative Models
๐ Research Objective:
– The paper aims to address challenges in stylized 3D scene reconstruction by proposing a framework, StyleMe3D, for effective 3D GS style transfer.
๐ ๏ธ Research Methods:
– The proposed method includes multi-modal style conditioning, multi-level semantic alignment, and specific innovations such as Dynamic Style Score Distillation, Contrastive Style Descriptor, Simultaneously Optimized Scale, and 3D Gaussian Quality Assessment to enhance stylistic and perceptual quality.
๐ฌ Research Conclusions:
– The framework significantly improves upon existing methods by preserving geometric details and achieving stylistic consistency in both isolated objects and complex scenes, facilitating real-time rendering applications in areas like gaming and digital art.
๐ Paper link: https://huggingface.co/papers/2504.15281
10. THOUGHTTERMINATOR: Benchmarking, Calibrating, and Mitigating Overthinking in Reasoning Models
๐ Keywords: Reasoning models, Overthinking, Problem-level difficulty, DUMB500, THOUGHTTERMINATOR
๐ก Category: Knowledge Representation and Reasoning
๐ Research Objective:
– The study aims to address the issue of overthinking in reasoning models by examining the relationship between problem difficulty and token spending.
๐ ๏ธ Research Methods:
– The authors introduce approximate measures of problem-level difficulty and evaluate reasoning models on their efficiency in token allocation. They use the DUMB500 dataset to test model calibration on easy problems and employ THOUGHTTERMINATOR, a training-free black box decoding technique, to improve calibration.
๐ฌ Research Conclusions:
– It was found that reasoning models are often poorly calibrated, especially on easier problems. The introduction of THOUGHTTERMINATOR significantly enhances the calibration of reasoning models.
๐ Paper link: https://huggingface.co/papers/2504.13367
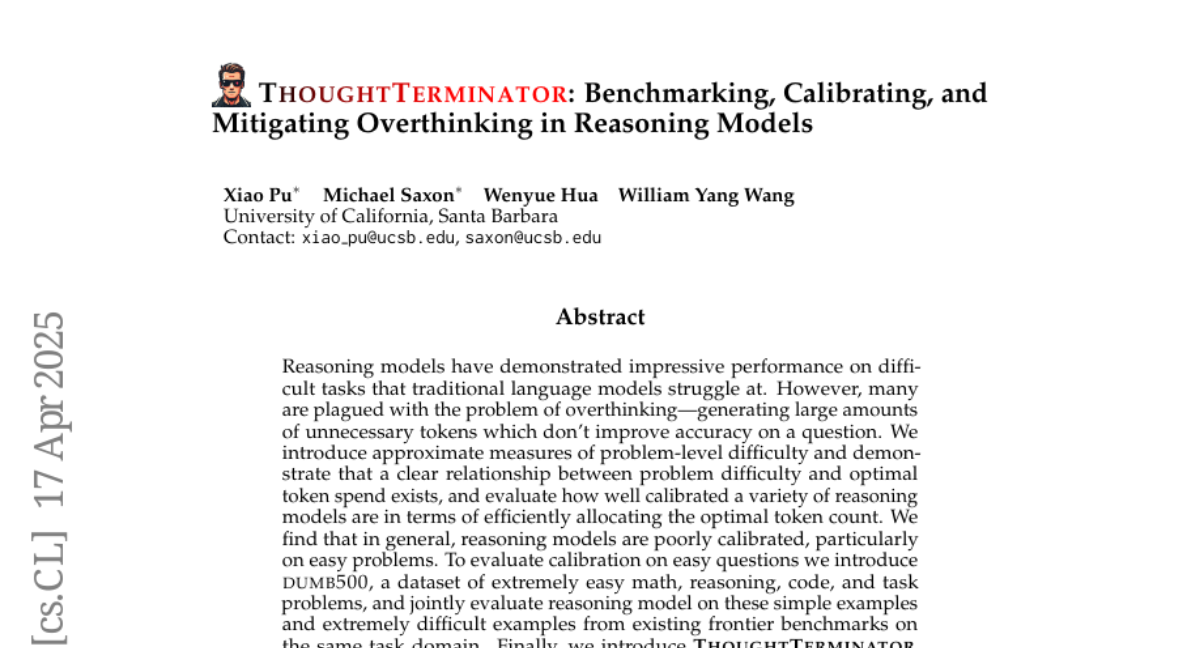
11. EasyEdit2: An Easy-to-use Steering Framework for Editing Large Language Models
๐ Keywords: EasyEdit2, Large Language Model, test-time interventions, seamless model steering
๐ก Category: Natural Language Processing
๐ Research Objective:
– The objective is to introduce EasyEdit2, a framework designed for plug-and-play adjustability to control Large Language Model behaviors without modifying its parameters.
๐ ๏ธ Research Methods:
– EasyEdit2 employs modules like the steering vector generator and the steering vector applier to automatically generate and apply steering vectors for model behavior adjustment.
๐ฌ Research Conclusions:
– EasyEdit2 provides a user-friendly approach for precise control of model responses, demonstrating effective model steering performance across different LLMs; source code and demonstration available on GitHub.
๐ Paper link: https://huggingface.co/papers/2504.15133
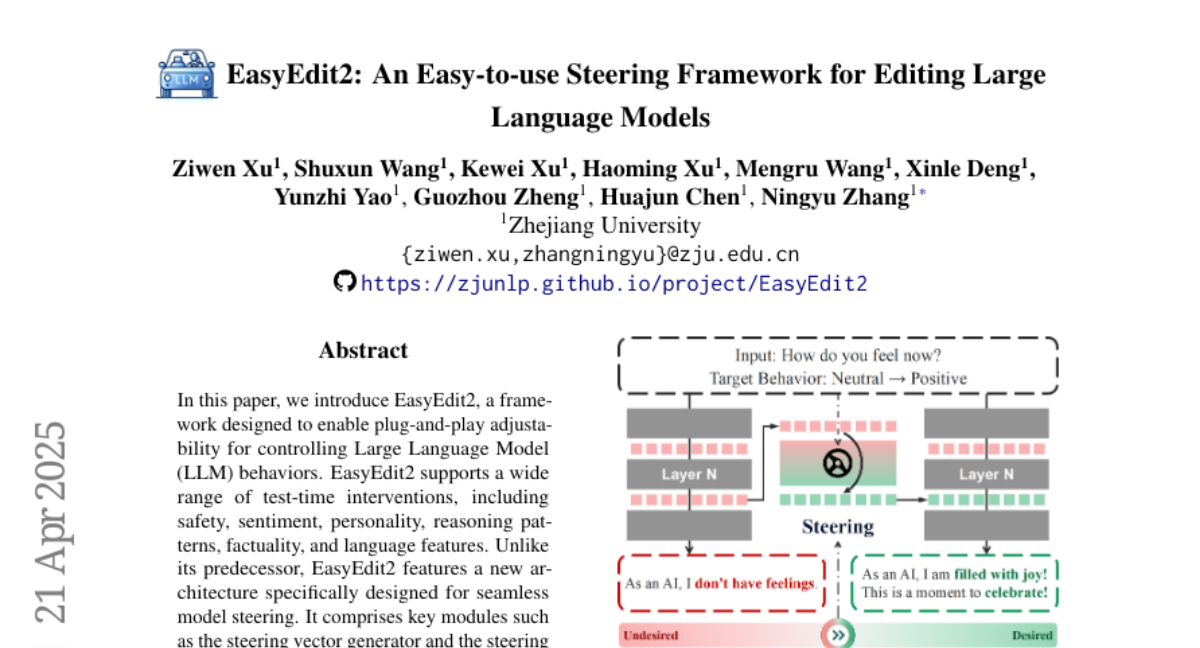
12. LeetCodeDataset: A Temporal Dataset for Robust Evaluation and Efficient Training of Code LLMs
๐ Keywords: LeetCodeDataset, code-generation models, LLM research, evaluation, supervised fine-tuning
๐ก Category: Natural Language Processing
๐ Research Objective:
– Introduce LeetCodeDataset as a benchmark for evaluating and training code-generation models to enhance reasoning-focused coding benchmarks and self-contained training testbeds.
๐ ๏ธ Research Methods:
– Curated LeetCode Python problems with rich metadata, broad coverage, 100+ test cases per problem, and temporal splits for contamination-free evaluation and efficient supervised fine-tuning.
๐ฌ Research Conclusions:
– Reasoning models significantly outperform non-reasoning counterparts, and supervised fine-tuning with 2.6K model-generated solutions achieves comparable performance to 110K-sample counterparts.
๐ Paper link: https://huggingface.co/papers/2504.14655
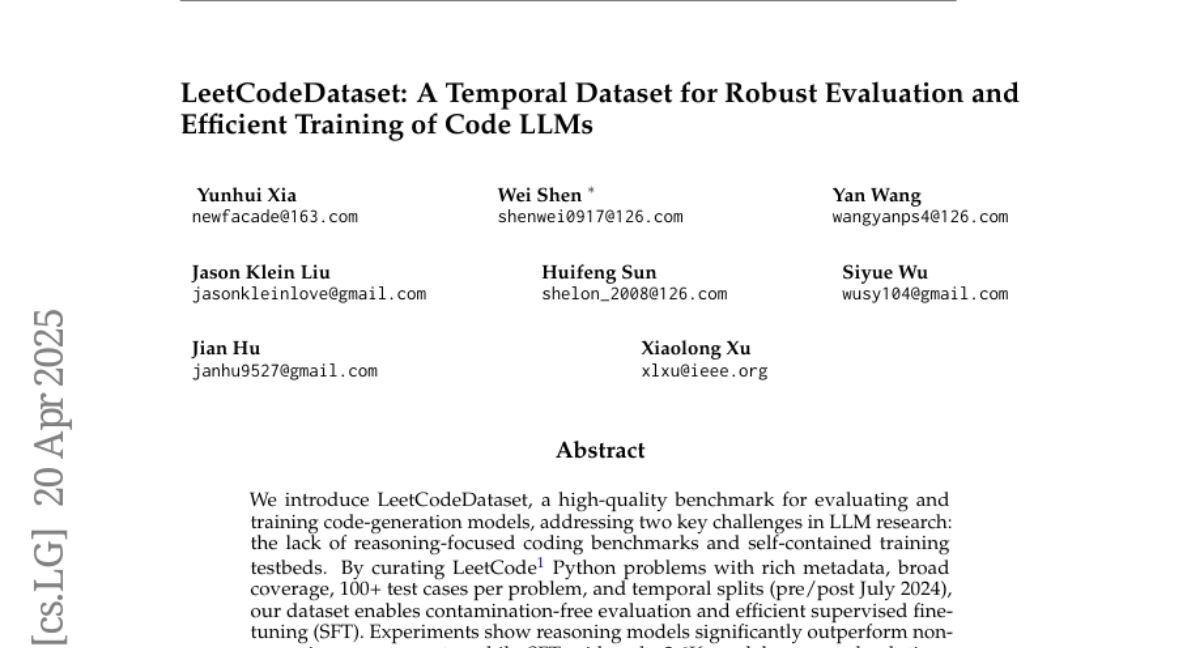
13. Seeing from Another Perspective: Evaluating Multi-View Understanding in MLLMs
๐ Keywords: Multi-view Understanding, Multi-Modal Large Language Models, All-Angles Bench, Geometric Consistency, Cross-view Correspondence
๐ก Category: Multi-Modal Learning
๐ Research Objective:
– To evaluate the challenges faced by Multi-Modal Large Language Models (MLLMs) in multi-view scene reasoning and enhance their performance as embodied agents.
๐ ๏ธ Research Methods:
– Developed the All-Angles Bench, a benchmark comprising over 2,100 human-annotated multi-view question-answer pairs across 90 diverse real-world scenes, designed to specifically test models on six tasks related to geometric correspondence and multi-view alignment.
๐ฌ Research Conclusions:
– Current MLLMs demonstrate a substantial performance gap compared to human evaluators, particularly in cross-view correspondence and establishing coarse camera poses, indicating the need for domain-specific refinements to improve multi-view awareness.
๐ Paper link: https://huggingface.co/papers/2504.15280
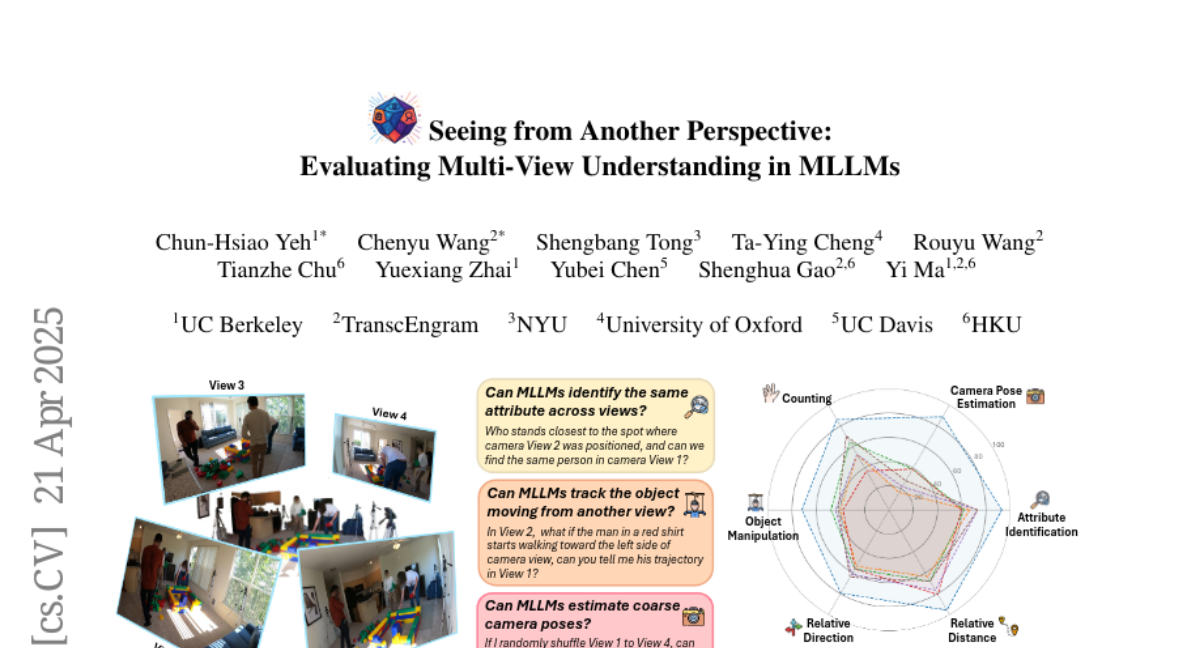
14. InfiGUI-R1: Advancing Multimodal GUI Agents from Reactive Actors to Deliberative Reasoners
๐ Keywords: Multimodal Large Language Models, GUI Agents, Actor2Reasoner framework, Reinforcement Learning
๐ก Category: Multi-Modal Learning
๐ Research Objective:
– The main aim is to advance GUI Agents from Reactive Actors to Deliberative Reasoners using the Actor2Reasoner framework.
๐ ๏ธ Research Methods:
– The methodology includes a two-stage training approach with Reasoning Injection to establish basic reasoning and Deliberation Enhancement to refine reasoning skills using Spatial Reasoning Distillation and Reinforcement Learning.
๐ฌ Research Conclusions:
– The introduction of InfiGUI-R1 shows strong performance in GUI grounding and trajectory tasks, demonstrating the feasibility of progressing agents toward deliberate reasoning.
๐ Paper link: https://huggingface.co/papers/2504.14239
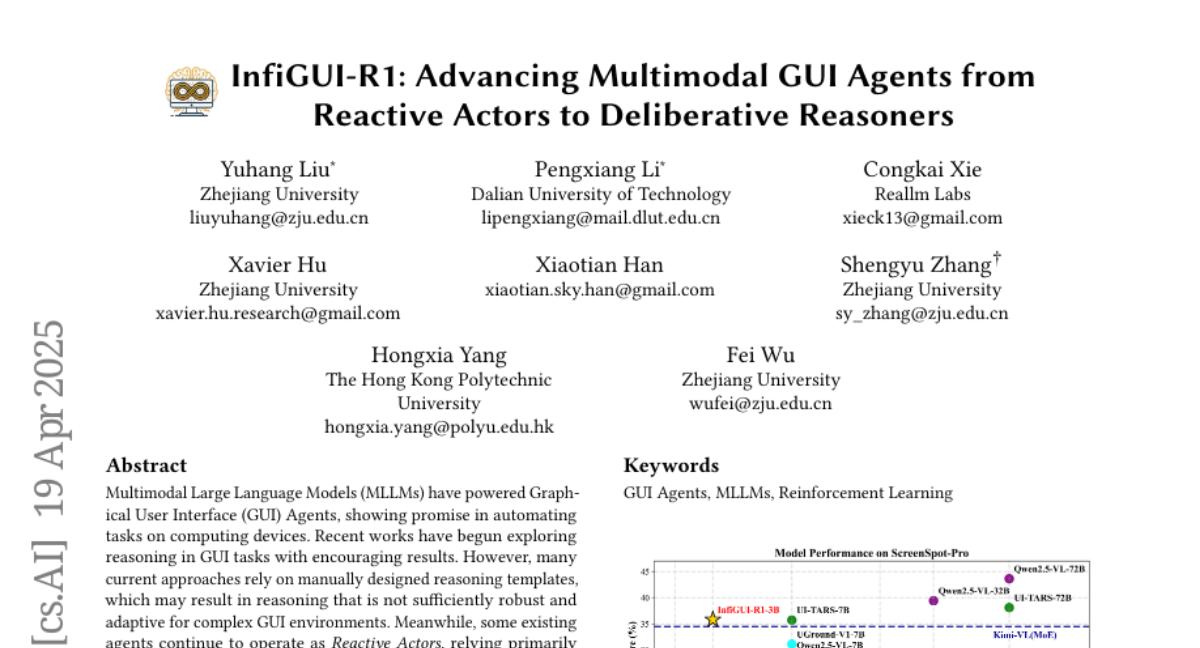
15. Uni3C: Unifying Precisely 3D-Enhanced Camera and Human Motion Controls for Video Generation
๐ Keywords: Uni3C, plug-and-play control module, PCDController, 3D priors, camera controllability
๐ก Category: Computer Vision
๐ Research Objective:
– The research introduces Uni3C, a unified 3D-enhanced framework for precise control of both camera and human motion in video generation.
๐ ๏ธ Research Methods:
– A plug-and-play control module, PCDController, utilizing unprojected point clouds from monocular depth is proposed to achieve accurate camera control.
๐ฌ Research Conclusions:
– Uni3C offers superior robustness and performance in camera controllability and human motion quality, outperforming competitors and validated with tailored challenging tests.
๐ Paper link: https://huggingface.co/papers/2504.14899
16. LearnAct: Few-Shot Mobile GUI Agent with a Unified Demonstration Benchmark
๐ Keywords: Mobile GUI agents, human demonstrations, LearnGUI, LearnAct, demonstration-based learning
๐ก Category: AI Systems and Tools
๐ Research Objective:
– To enhance mobile GUI agent capabilities through human demonstrations, prioritizing performance in unseen scenarios over universal generalization.
๐ ๏ธ Research Methods:
– Introduced LearnGUI, the first comprehensive dataset for demonstration-based learning in mobile GUI agents.
– Developed LearnAct, a multi-agent framework that uses specific agents for knowledge extraction, retrieval, and task execution.
๐ฌ Research Conclusions:
– Demonstration-based learning significantly improves task performance of mobile GUI agents, with substantial accuracy increases in both offline and online evaluations.
๐ Paper link: https://huggingface.co/papers/2504.13805
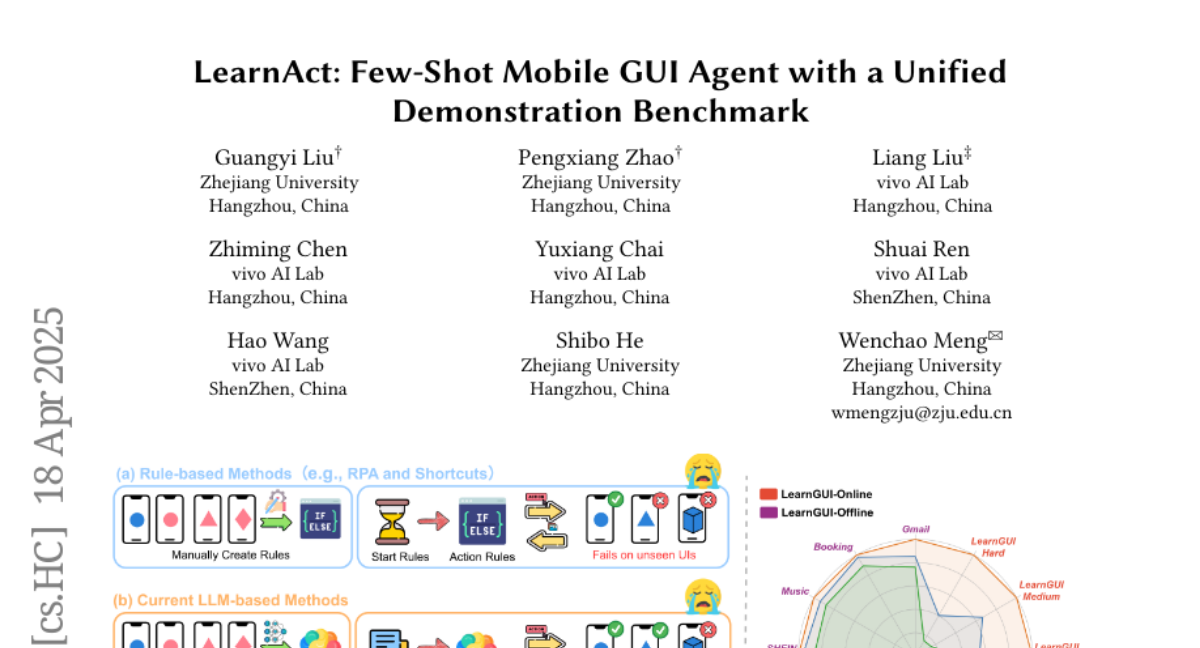
17. LookingGlass: Generative Anamorphoses via Laplacian Pyramid Warping
๐ Keywords: Anamorphosis, Generative perceptual illusions, Latent rectified flow models, Laplacian Pyramid Warping
๐ก Category: Generative Models
๐ Research Objective:
– The study aims to revisit anamorphic images with a generative twist, making them interpretable both directly and from specific viewpoints.
๐ ๏ธ Research Methods:
– The researchers utilized latent rectified flow models and introduced a frequency-aware technique called Laplacian Pyramid Warping to generate high-quality anamorphic images.
๐ฌ Research Conclusions:
– The work extends Visual Anagrams to latent space models, enabling the creation of novel generative perceptual illusions through diverse spatial transforms.
๐ Paper link: https://huggingface.co/papers/2504.08902
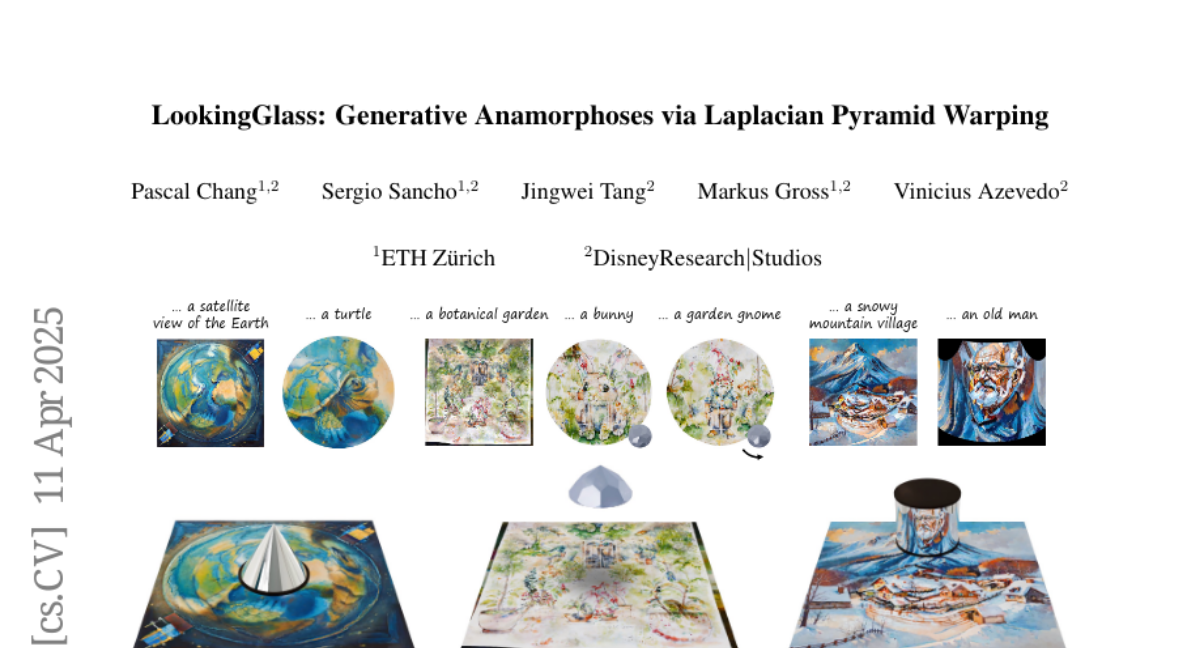
18. An LMM for Efficient Video Understanding via Reinforced Compression of Video Cubes
๐ Keywords: Large Multimodal Models, Gumbel Softmax, Spatiotemporal Redundancy, Video-MME, Cubing Network
๐ก Category: Multi-Modal Learning
๐ Research Objective:
– Introduce Quicksviewer, a new paradigm for efficient video understanding by partitioning videos with varying temporal density.
๐ ๏ธ Research Methods:
– Utilizes a Gumbel Softmax approach to dynamically compress video data, followed by unified resampling, leveraging a language backbone through progressive training stages.
๐ฌ Research Conclusions:
– Achieves 45x compression rate in video spatiotemporal data, outperforms baselines by up to 8.72 accuracy, and demonstrates SOTA performance in Video-MME with minimal resource usage.
๐ Paper link: https://huggingface.co/papers/2504.15270
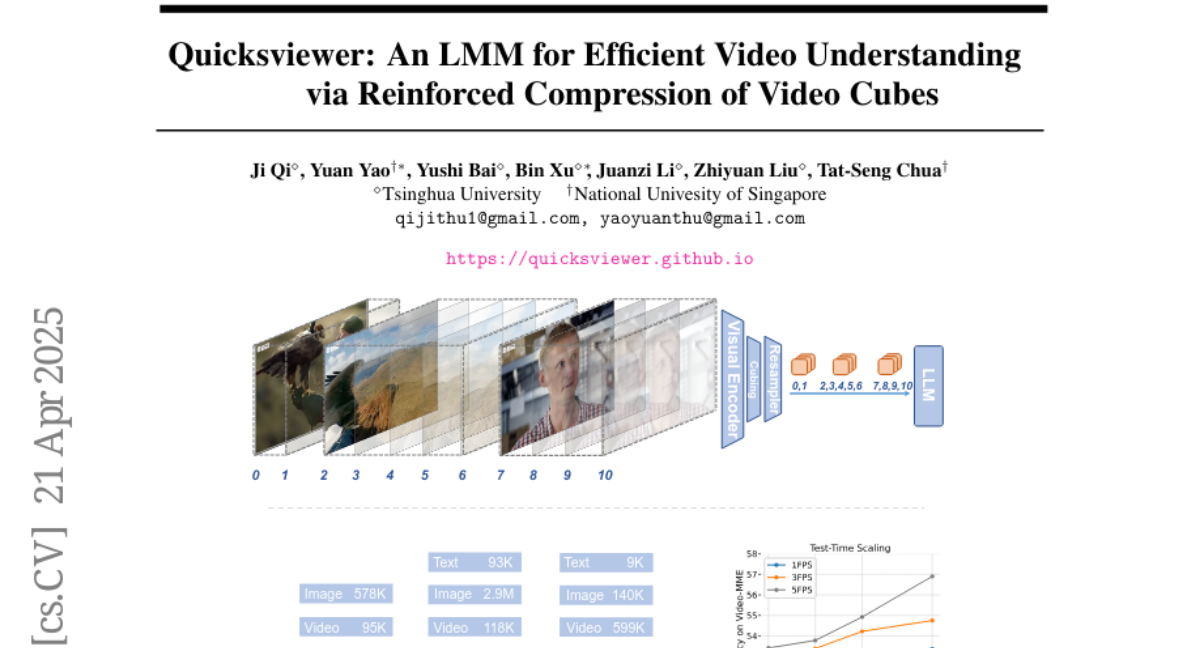
19. NEMOTRON-CROSSTHINK: Scaling Self-Learning beyond Math Reasoning
๐ Keywords: Large Language Models, Reinforcement Learning, Generalization, NEMOTRON-CROSSTHINK, Verifiable Reward Modeling
๐ก Category: Reinforcement Learning
๐ Research Objective:
– To enhance the generalization capabilities of Large Language Models (LLMs) for diverse reasoning tasks beyond mathematical reasoning, using a new framework called NEMOTRON-CROSSTHINK.
๐ ๏ธ Research Methods:
– Incorporation of multi-domain corpora, application of structured templates, filtering for verifiable answers, and optimization of data blending strategies in Reinforcement Learning training.
๐ฌ Research Conclusions:
– The NEMOTRON-CROSSTHINK framework enables scalable and verifiable reward modeling, leading to significant improvements in accuracy and response efficiency across both mathematical and non-mathematical reasoning tasks.
๐ Paper link: https://huggingface.co/papers/2504.13941
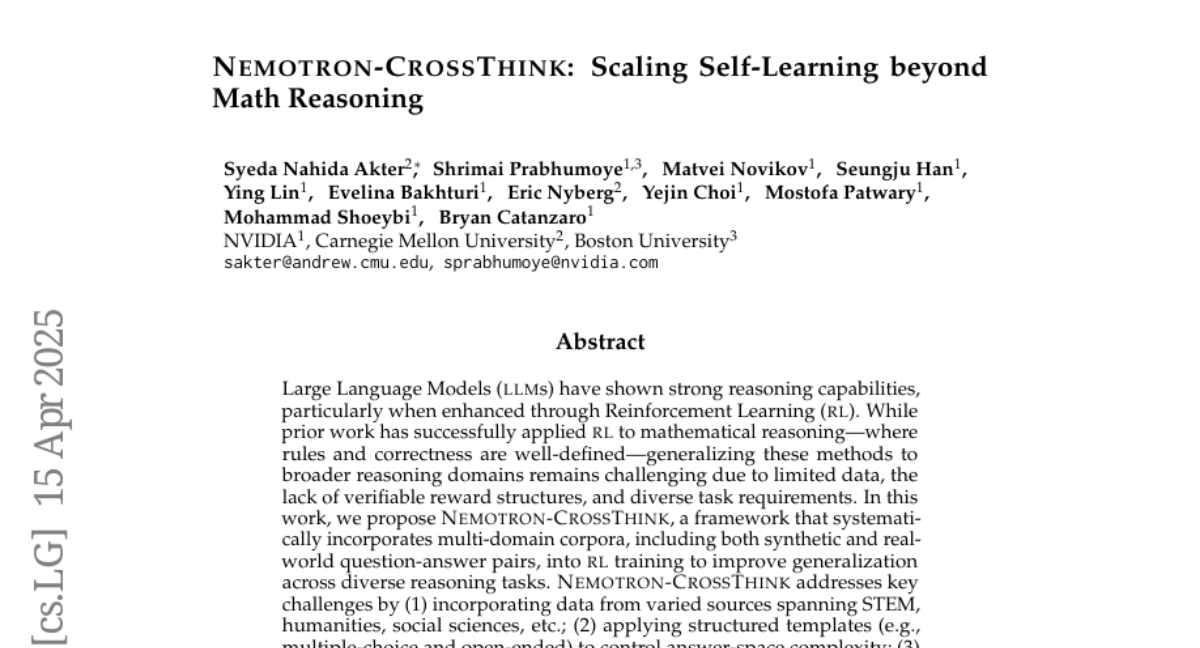
20. DRAGON: Distributional Rewards Optimize Diffusion Generative Models
๐ Keywords: Generative Optimization, Fine-Tuning, Media Generation Models, Reward Functions
๐ก Category: Generative Models
๐ Research Objective:
– The aim is to present a new framework, DRAGON, for optimizing media generation models towards desired outcomes using versatile reward functions.
๐ ๏ธ Research Methods:
– Utilizes a flexible framework that evaluates reward functions on individual examples or their distributions and applies various cross-modality encoders.
– Conducts experiments with an audio-domain text-to-music diffusion model using 20 different reward functions to assess performance.
๐ฌ Research Conclusions:
– DRAGON demonstrates high performance with an 81.45% average win rate across rewards and enhances music generation quality without human preference annotations.
– Exemplar-based reward functions are effective and comparable to model-based approaches in improving human-perceived quality.
๐ Paper link: https://huggingface.co/papers/2504.15217
21. TAPIP3D: Tracking Any Point in Persistent 3D Geometry
๐ Keywords: TAPIP3D, 3D point tracking, 3D trajectory estimation, camera-stabilized, Local Pair Attention mechanism
๐ก Category: Computer Vision
๐ Research Objective:
– Introduce TAPIP3D, a novel method for robust long-term 3D point tracking in monocular RGB and RGB-D videos.
๐ ๏ธ Research Methods:
– Represent videos as camera-stabilized spatio-temporal feature clouds, leveraging depth and camera motion.
– Propose a Local Pair Attention mechanism to handle 3D point distribution irregularities and estimate precise 3D trajectories.
๐ฌ Research Conclusions:
– TAPIP3D outperforms existing 3D and 2D tracking methods by stabilizing camera motion and forming better feature neighborhoods.
– Enhanced tracking accuracy in both 3D and 2D, supporting inference in both camera and world coordinates.
๐ Paper link: https://huggingface.co/papers/2504.14717
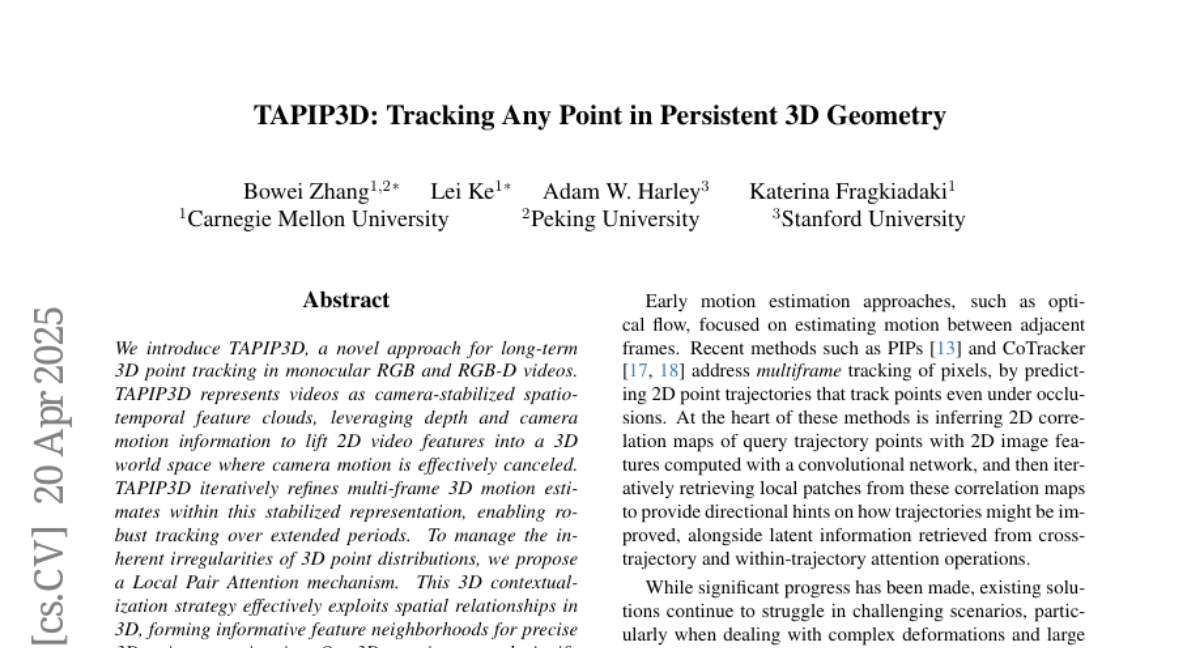
22. RainbowPlus: Enhancing Adversarial Prompt Generation via Evolutionary Quality-Diversity Search
๐ Keywords: Large Language Models, Adversarial Prompts, Evolutionary Computation, Red-Teaming, Vulnerability Assessment
๐ก Category: Natural Language Processing
๐ Research Objective:
– Introduce RainbowPlus, a novel red-teaming framework, to enhance the generation of adversarial prompts through adaptive quality-diversity search and evolutionary computation.
๐ ๏ธ Research Methods:
– Utilized evolutionary algorithms like MAP-Elites, employing a multi-element archive and a comprehensive fitness function to store and evaluate diverse high-quality prompts.
๐ฌ Research Conclusions:
– RainbowPlus demonstrated superior attack success rates and prompt diversity compared to existing QD methods across multiple datasets and LLMs, achieving faster performance and generating significantly more unique prompts.
๐ Paper link: https://huggingface.co/papers/2504.15047
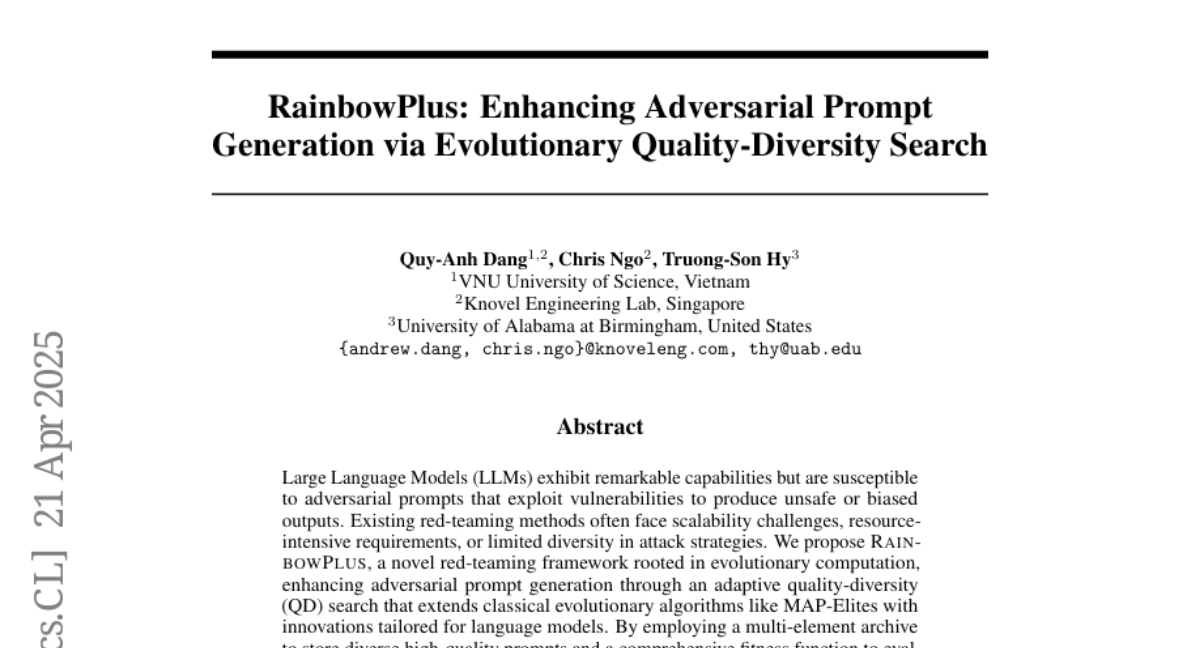
23. RF-DETR Object Detection vs YOLOv12 : A Study of Transformer-based and CNN-based Architectures for Single-Class and Multi-Class Greenfruit Detection in Complex
Orchard Environments Under Label Ambiguity
๐ Keywords: RF-DETR, YOLOv12, Object Detection, Precision Agriculture, Transformer-based Architectures
๐ก Category: Computer Vision
๐ Research Objective:
– To compare RF-DETR and YOLOv12 object detection models for detecting greenfruits in complex orchard environments.
๐ ๏ธ Research Methods:
– Developed a custom dataset with single-class and multi-class annotations.
– Analyzed model performance under real-world conditions marked by label ambiguity and occlusion using metrics such as mean Average Precision.
๐ฌ Research Conclusions:
– RF-DETR excels in global context modeling, effectively identifying partially occluded or ambiguous greenfruits, achieving a high mAP50 of 0.9464 in single-class detection.
– YOLOv12 optimizes for computational efficiency, excelling in edge deployment scenarios, with high mAP@50:95 scores indicating better classification in detailed contexts.
– RF-DETR’s swift convergence in training demonstrates the efficiency of transformer-based architectures for precision agricultural applications.
๐ Paper link: https://huggingface.co/papers/2504.13099
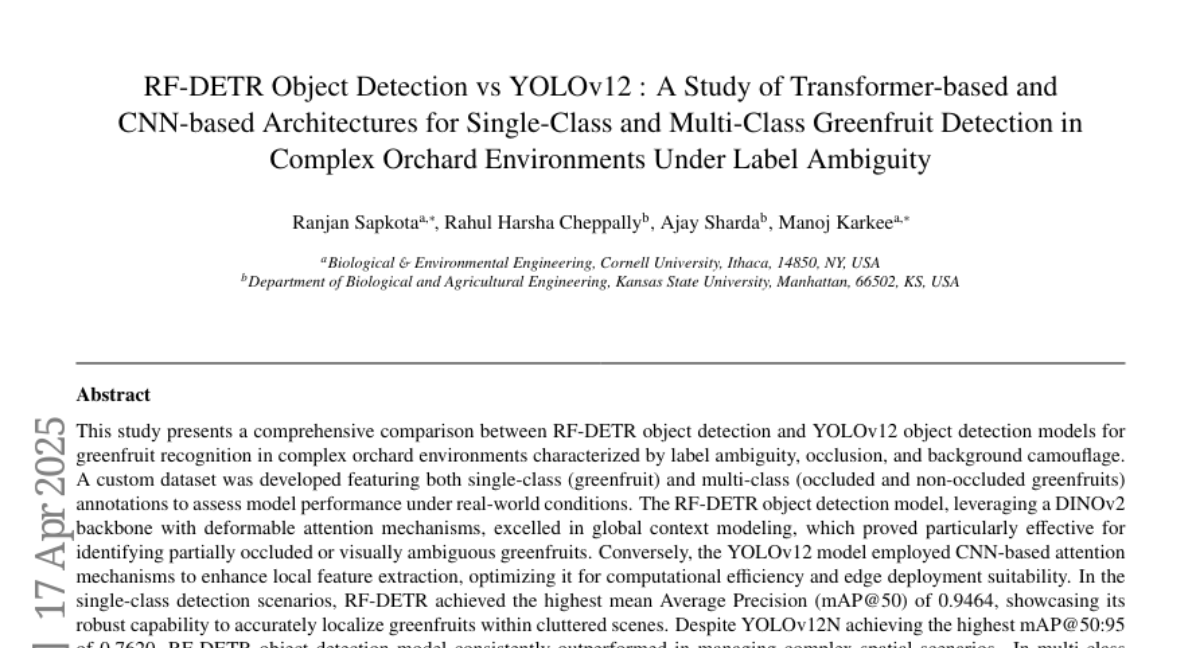
24. CoMotion: Concurrent Multi-person 3D Motion
๐ Keywords: 3D poses, monocular camera, temporally coherent predictions, online tracking, pseudo-labeled annotations
๐ก Category: Computer Vision
๐ Research Objective:
– Introduce a method for detecting and tracking detailed 3D poses of multiple people using a single monocular camera.
๐ ๏ธ Research Methods:
– Utilize temporally coherent predictions and direct pose updates from new input images to enhance tracking in crowded scenes with occlusions.
– Leverage pseudo-labeled annotations from numerous datasets to train the model.
๐ฌ Research Conclusions:
– Developed a model that matches state-of-the-art 3D pose estimation accuracy but offers improved speed and accuracy in tracking multiple people over time.
๐ Paper link: https://huggingface.co/papers/2504.12186
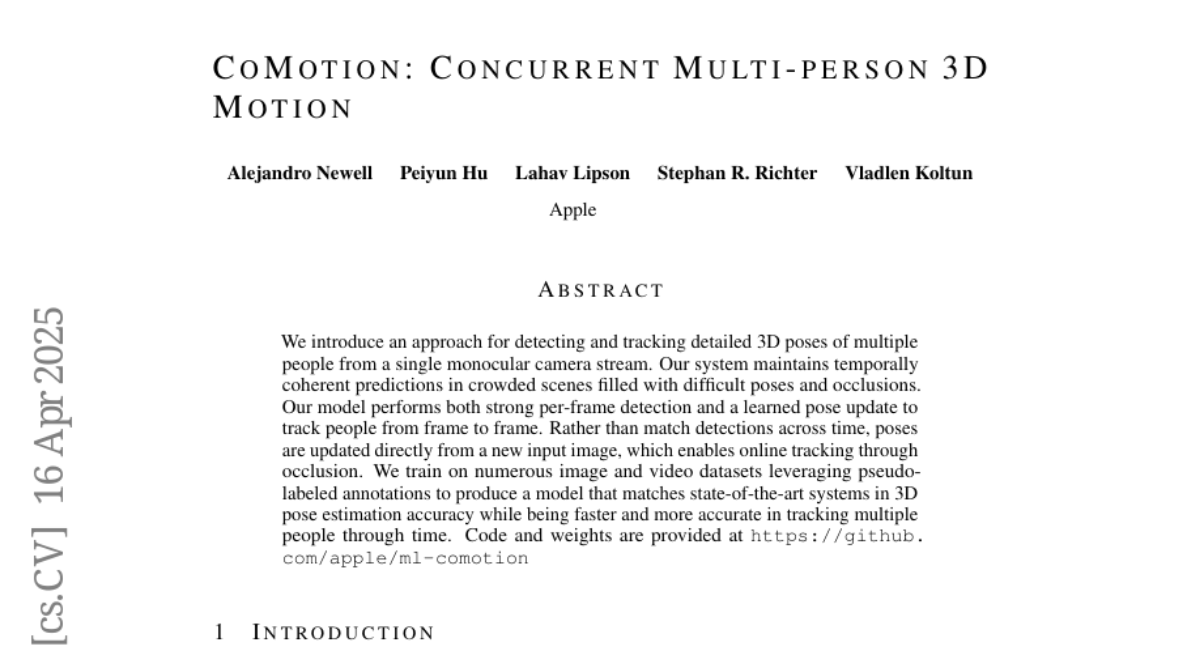
25. SilVar-Med: A Speech-Driven Visual Language Model for Explainable Abnormality Detection in Medical Imaging
๐ Keywords: Medical Visual Language Models, Speech-driven, Multimodal, Medical image analysis, Voice-based communication
๐ก Category: AI in Healthcare
๐ Research Objective:
– To address the limitations of text-based interactions in medical Visual Language Models (VLM) and to introduce an end-to-end speech-driven medical VLM called SilVar-Med for improved usability in clinical environments.
๐ ๏ธ Research Methods:
– Developed a multimodal medical image assistant integrating speech interaction with Visual Language Models, and introduced a reasoning dataset for interpreting medical image predictions.
๐ฌ Research Conclusions:
– Demonstrated the potential for more transparent, interactive, and clinically viable diagnostic support systems, offering proof-of-concept for reasoning-driven medical image interpretation using end-to-end speech interaction.
๐ Paper link: https://huggingface.co/papers/2504.10642
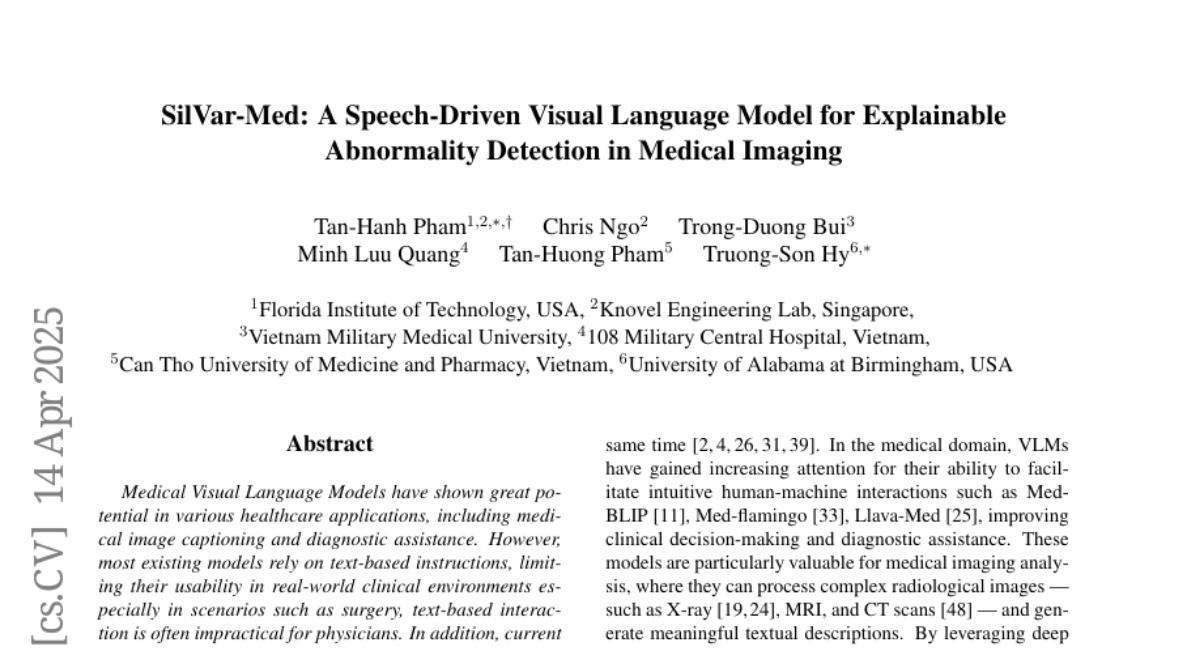
26. LoftUp: Learning a Coordinate-Based Feature Upsampler for Vision Foundation Models
๐ Keywords: Vision Foundation Models, Feature Upsampling, Cross-Attention Transformer, High-Resolution Images, Self-Distillation
๐ก Category: Computer Vision
๐ Research Objective:
– To enhance feature upsampling in Vision Foundation Models for improved pixel-level understanding by investigating upsampler architecture and training objectives.
๐ ๏ธ Research Methods:
– Introduced a coordinate-based cross-attention transformer integrating high-resolution images with coordinates and low-resolution features.
– Proposed using high-resolution pseudo-groundtruth features through class-agnostic masks and self-distillation.
๐ฌ Research Conclusions:
– The proposed approach captures fine-grained details effectively and outperforms existing feature upsampling techniques across various downstream tasks.
๐ Paper link: https://huggingface.co/papers/2504.14032
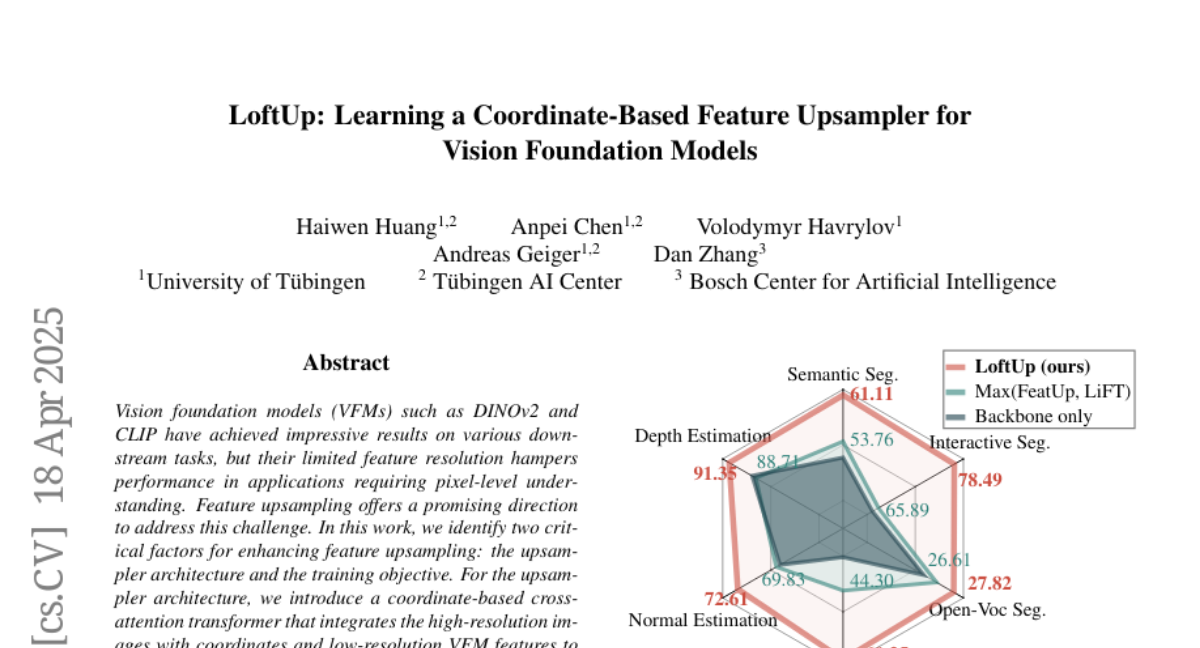
27. PROMPTEVALS: A Dataset of Assertions and Guardrails for Custom Production Large Language Model Pipelines
๐ Keywords: LLMs, PROMPTEVALS, Assertion Criteria, Fine-tuned Mistral, Reliability
๐ก Category: AI Systems and Tools
๐ Research Objective:
– The objective is to improve the reliability of Large Language Models (LLMs) in production data processing pipelines by developing assertions or guardrails for model outputs.
๐ ๏ธ Research Methods:
– The study introduces PROMPTEVALS, a dataset significantly larger than previous ones, used to evaluate both closed- and open-source models in generating assertions, with a benchmark test on a hold-out split.
๐ฌ Research Conclusions:
– Fine-tuned Mistral and Llama 3 models surpass GPT-4o by 20.93% in performance, demonstrating both lower latency and increased effectiveness, contributing to advancements in LLM reliability and prompt engineering.
๐ Paper link: https://huggingface.co/papers/2504.14738
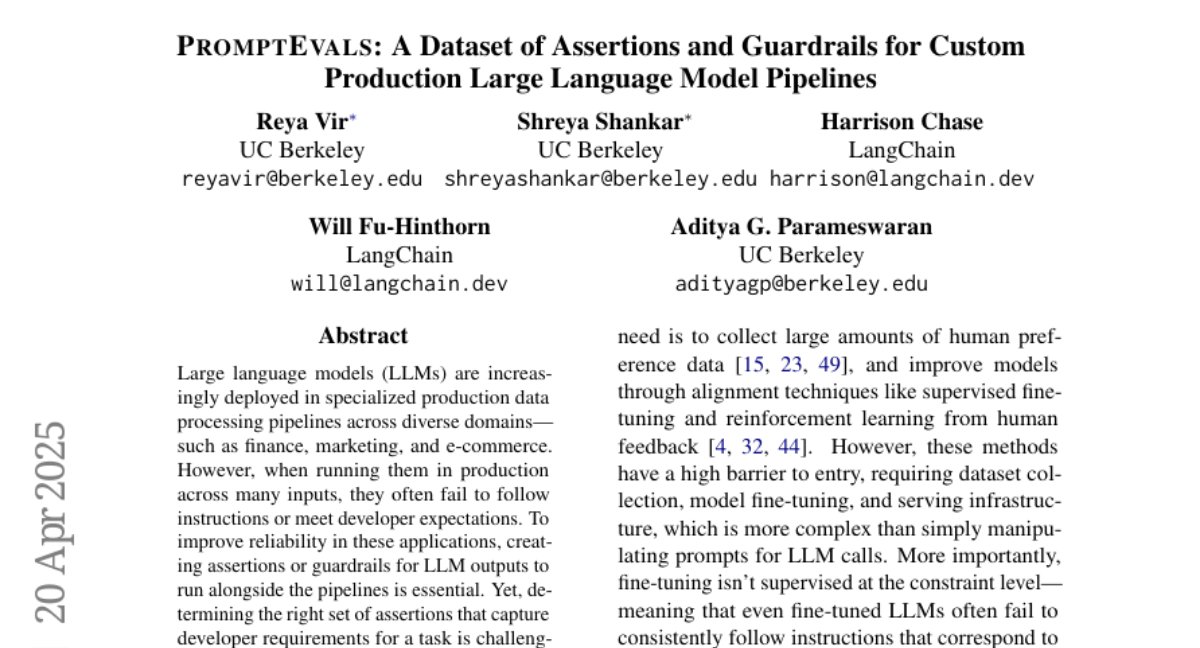
28. Roll the dice & look before you leap: Going beyond the creative limits of next-token prediction
๐ Keywords: open-ended tasks, stochastic planning, abstract knowledge graph, next-token learning, diffusion models
๐ก Category: Generative Models
๐ Research Objective:
– The study aims to quantify the creative limits of present-day language models through minimal algorithmic tasks designed as abstractions of open-ended real-world tasks.
๐ ๏ธ Research Methods:
– Investigates the efficiency of multi-token approaches like teacherless training and diffusion models in comparison to next-token learning.
– Proposes a method called hash-conditioning to inject randomness in the input layer to maintain coherence in creativity tasks.
๐ฌ Research Conclusions:
– Multi-token approaches are more effective in producing diverse and original outputs than next-token learning.
– Introduces a new testing environment to analyze open-ended creative skills and suggests advancements beyond softmax-based sampling.
๐ Paper link: https://huggingface.co/papers/2504.15266
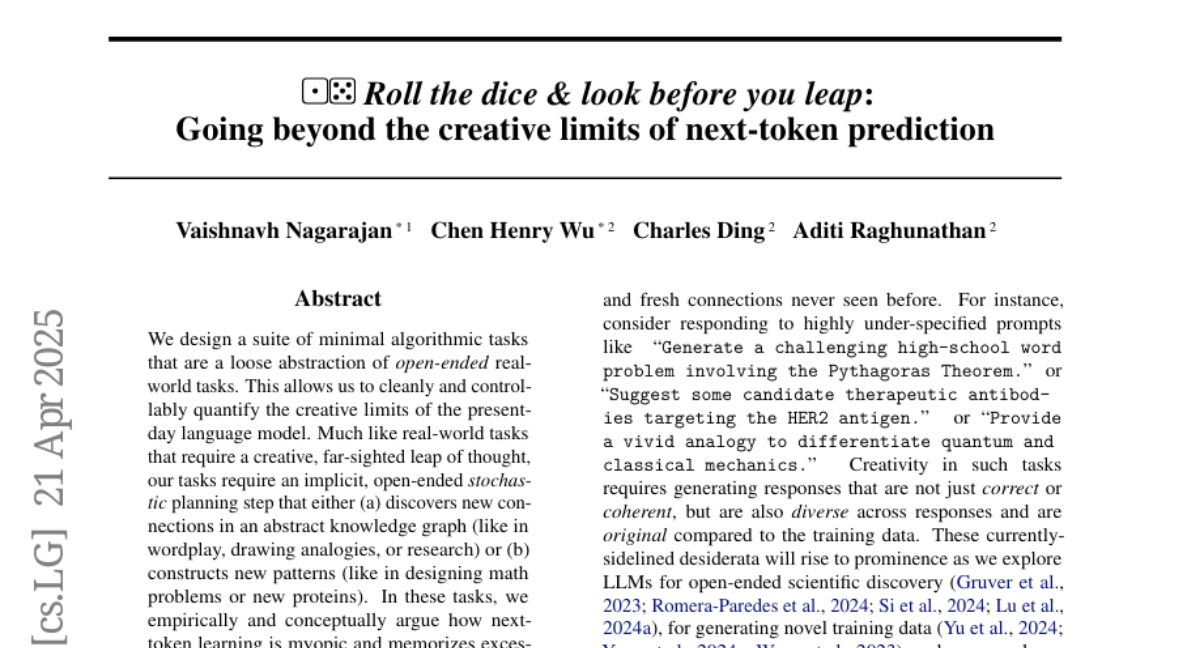
29.
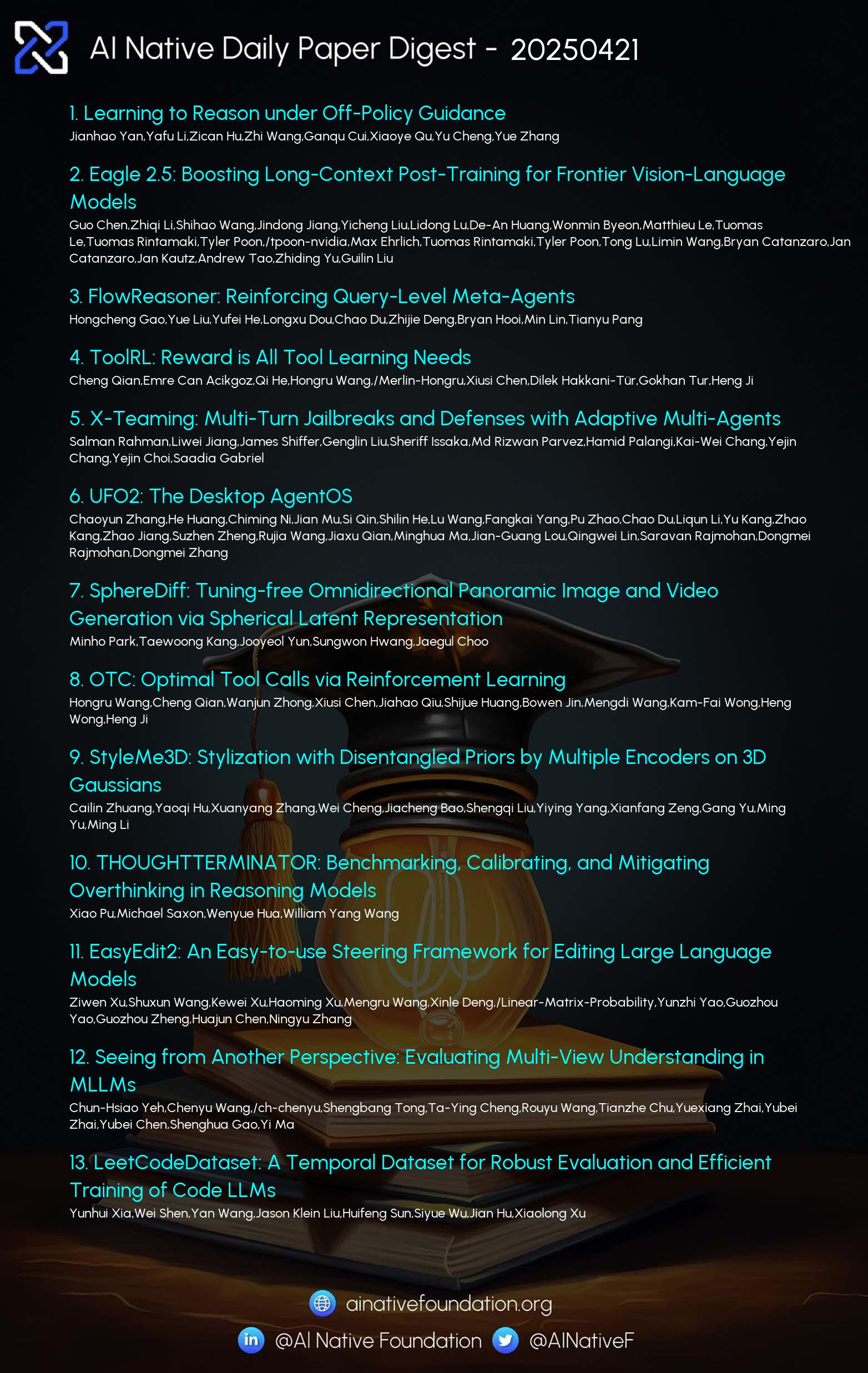