AI Native Daily Paper Digest – 20250509
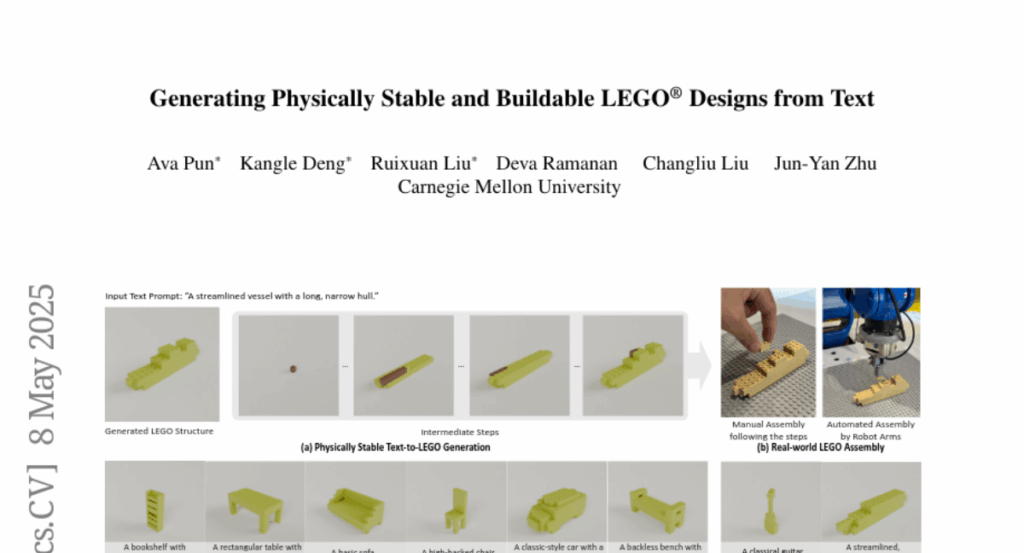
1. Perception, Reason, Think, and Plan: A Survey on Large Multimodal Reasoning Models
๐ Keywords: Large Multimodal Reasoning Models, Multimodal Reasoning, Cross-modal Understanding, Multimodal Chain-of-Thought, Native Large Multimodal Reasoning Models
๐ก Category: Multi-Modal Learning
๐ Research Objective:
– The paper aims to present a comprehensive survey of multimodal reasoning research, focusing on the development and capabilities of Large Multimodal Reasoning Models (LMRMs).
๐ ๏ธ Research Methods:
– The research is structured around a four-stage developmental roadmap that reflects shifts in design philosophies, reviewing early task-specific module efforts to more recent unified multimodal reasoning approaches, such as Multimodal Chain-of-Thought (MCoT) and multimodal reinforcement learning.
๐ฌ Research Conclusions:
– Empirical insights are drawn from challenging benchmarks to discuss the conceptual direction of native large multimodal reasoning models (N-LMRMs), aiming to support scalable, agentic, and adaptive reasoning in complex, real-world environments.
๐ Paper link: https://huggingface.co/papers/2505.04921
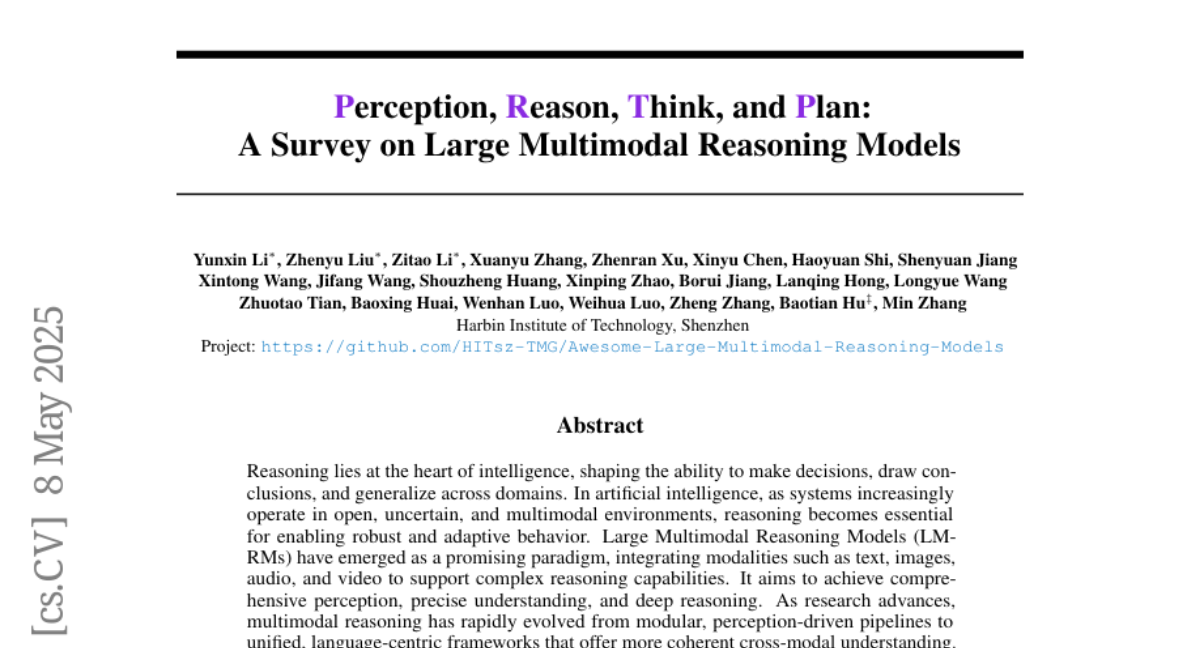
2. On Path to Multimodal Generalist: General-Level and General-Bench
๐ Keywords: Multimodal Large Language Model, Multimodal Generalist, Synergy, General-Level, General-Bench
๐ก Category: Multi-Modal Learning
๐ Research Objective:
– This research aims to evaluate and rank Multimodal Large Language Models (MLLMs) to assess their progress towards becoming more robust multimodal generalists, ultimately aspiring to achieve AGI.
๐ ๏ธ Research Methods:
– Introduction of the General-Level framework to define a 5-scale performance and generality level for MLLMs, using a new benchmark called General-Bench, which evaluates models across over 700 tasks and 325,800 instances.
๐ฌ Research Conclusions:
– The analysis reveals insights into the capability rankings of existing state-of-the-art MLLMs and highlights the challenges in achieving genuine AI, providing groundwork for future research on next-generation multimodal foundation models.
๐ Paper link: https://huggingface.co/papers/2505.04620
3. Flow-GRPO: Training Flow Matching Models via Online RL
๐ Keywords: Flow-GRPO, flow matching models, ODE-to-SDE conversion, RL-tuned SD3.5, GenEval accuracy
๐ก Category: Generative Models
๐ Research Objective:
– Introduce Flow-GRPO, a novel approach integrating online reinforcement learning into flow matching models.
๐ ๏ธ Research Methods:
– Utilizes an ODE-to-SDE conversion for maintaining model distribution while enabling RL exploration.
– Implements a Denoising Reduction strategy to enhance sampling efficiency without losing performance.
๐ฌ Research Conclusions:
– Demonstrated effectiveness in text-to-image tasks with significant improvements in object counts, spatial relations, and attributes.
– Achieved accuracy boosts in visual text rendering and exhibited gains in human preference alignment without compromising image quality.
๐ Paper link: https://huggingface.co/papers/2505.05470
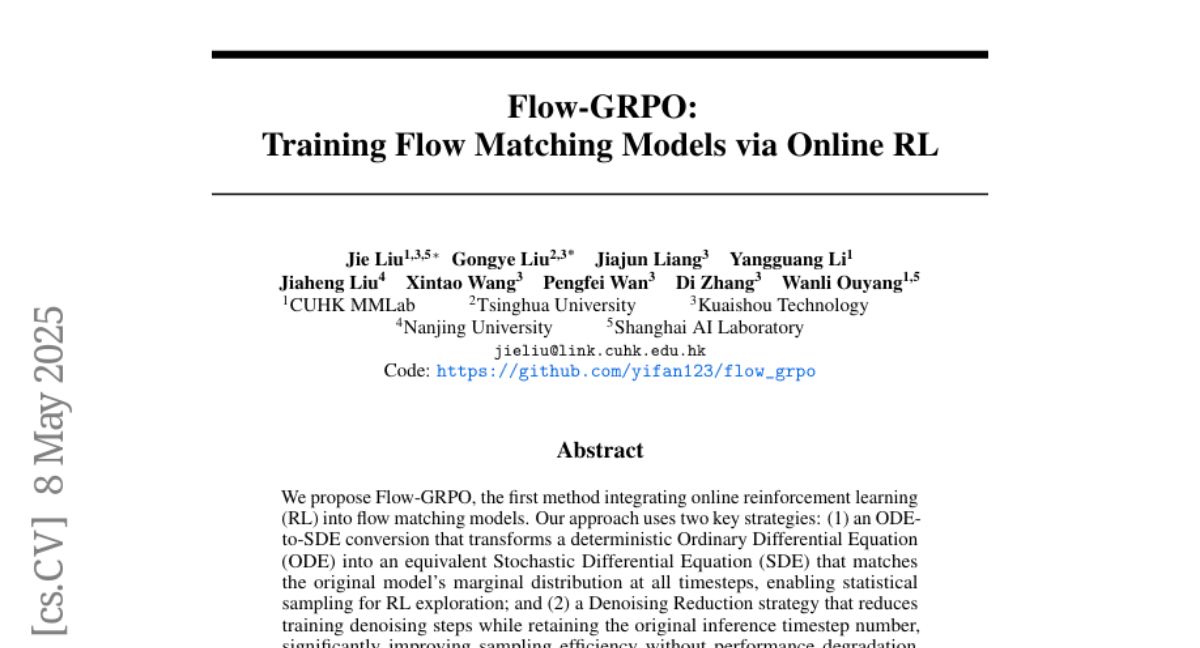
4. Sentient Agent as a Judge: Evaluating Higher-Order Social Cognition in Large Language Models
๐ Keywords: Sentient Agent as a Judge (SAGE), higher-order social cognition, psychological fidelity, empathetic, socially adept language agents
๐ก Category: Natural Language Processing
๐ Research Objective:
– To introduce SAGE as an evaluation framework measuring large language models’ understanding by simulating human-like emotional changes and inner thoughts.
๐ ๏ธ Research Methods:
– Utilization of Sentient Agent to simulate multi-turn conversations with human-like reasoning, yielding emotion trajectories and interpretable inner thoughts.
๐ฌ Research Conclusions:
– SAGE’s Sentient emotion scores correlate strongly with psychological metrics, revealing gaps between advanced and baseline models, not captured by conventional leaderboards.
๐ Paper link: https://huggingface.co/papers/2505.02847
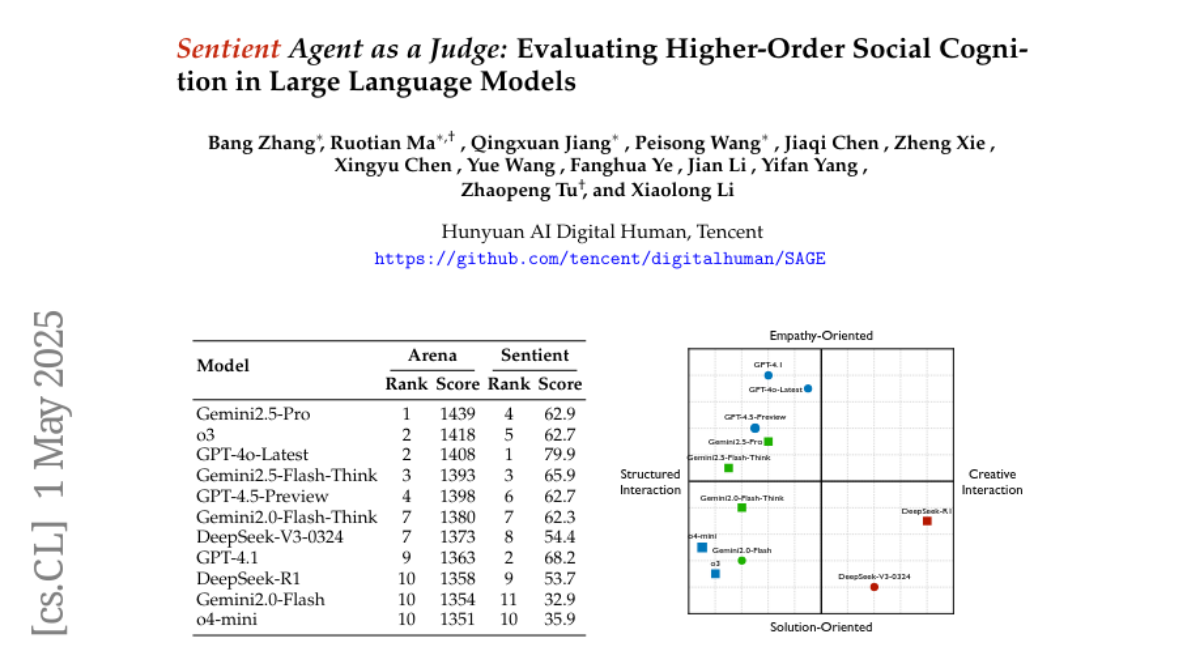
5. Scalable Chain of Thoughts via Elastic Reasoning
๐ Keywords: Large reasoning models, Elastic Reasoning, scalable chain of thoughts, GRPO, budget constraints
๐ก Category: Knowledge Representation and Reasoning
๐ Research Objective:
– Introducing Elastic Reasoning, a framework to manage the length of chains of thought in large reasoning models to meet real-world deployment constraints.
๐ ๏ธ Research Methods:
– Elastic Reasoning separates reasoning into thinking and solution phases, each with independent budgets, combined with a lightweight budget-constrained rollout strategy within the GRPO framework.
๐ฌ Research Conclusions:
– Elastic Reasoning enhances reliability under resource limitations and produces concise reasoning, outperforming baselines in both constrained and unconstrained scenarios, with reduced training costs.
๐ Paper link: https://huggingface.co/papers/2505.05315
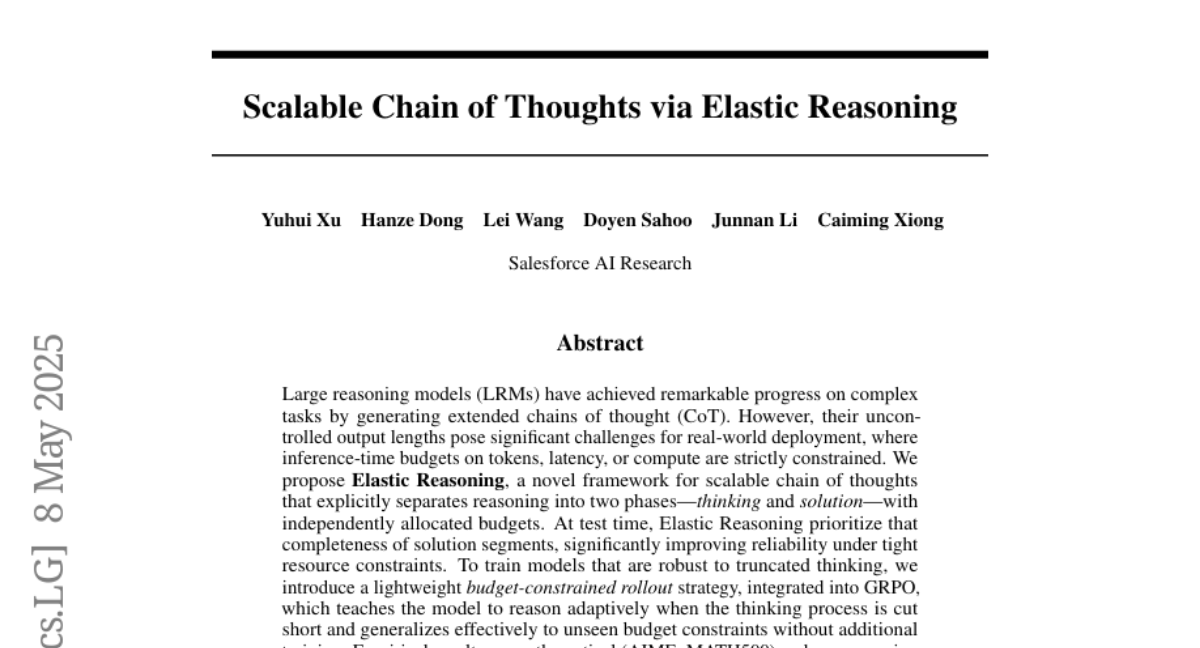
6. FG-CLIP: Fine-Grained Visual and Textual Alignment
๐ Keywords: Fine-Grained CLIP, Multimodal Models, Fine-Grained Understanding, Image-Text Retrieval, Open-Vocabulary Object Detection
๐ก Category: Multi-Modal Learning
๐ Research Objective:
– To enhance fine-grained understanding in multimodal tasks by proposing the Fine-Grained CLIP (FG-CLIP) model.
๐ ๏ธ Research Methods:
– Utilized large multimodal models to generate 1.6 billion long caption-image pairs.
– Constructed a high-quality dataset with 12 million images and 40 million region-specific bounding boxes with detailed captions.
– Incorporated 10 million hard fine-grained negative samples to improve semantic distinction.
๐ฌ Research Conclusions:
– FG-CLIP outperforms the original CLIP and other state-of-the-art methods in various downstream tasks, highlighting its effectiveness in capturing fine-grained image details.
๐ Paper link: https://huggingface.co/papers/2505.05071
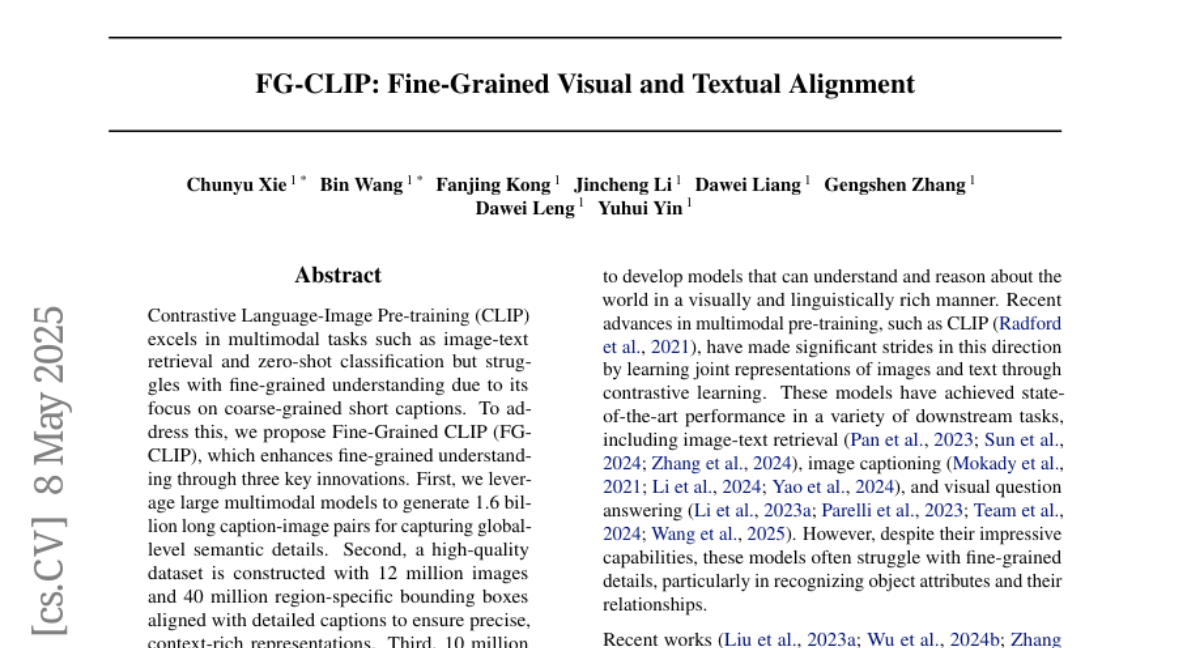
7. 3D Scene Generation: A Survey
๐ Keywords: 3D scene generation, deep generative models, diffusion models, photorealism, embodied AI
๐ก Category: Generative Models
๐ Research Objective:
– This paper aims to provide a systematic overview of state-of-the-art approaches to 3D scene generation, focusing on synthesizing spatially structured, semantically meaningful, and photorealistic environments.
๐ ๏ธ Research Methods:
– The survey organizes approaches into four paradigms: procedural generation, neural 3D-based generation, image-based generation, and video-based generation. It analyzes their technical foundations, trade-offs, and representative results.
๐ฌ Research Conclusions:
– The paper discusses key challenges in areas such as generation capacity, 3D representation, and evaluation. It outlines promising directions, including higher fidelity, physics-aware and interactive generation, and unified perception-generation models.
๐ Paper link: https://huggingface.co/papers/2505.05474
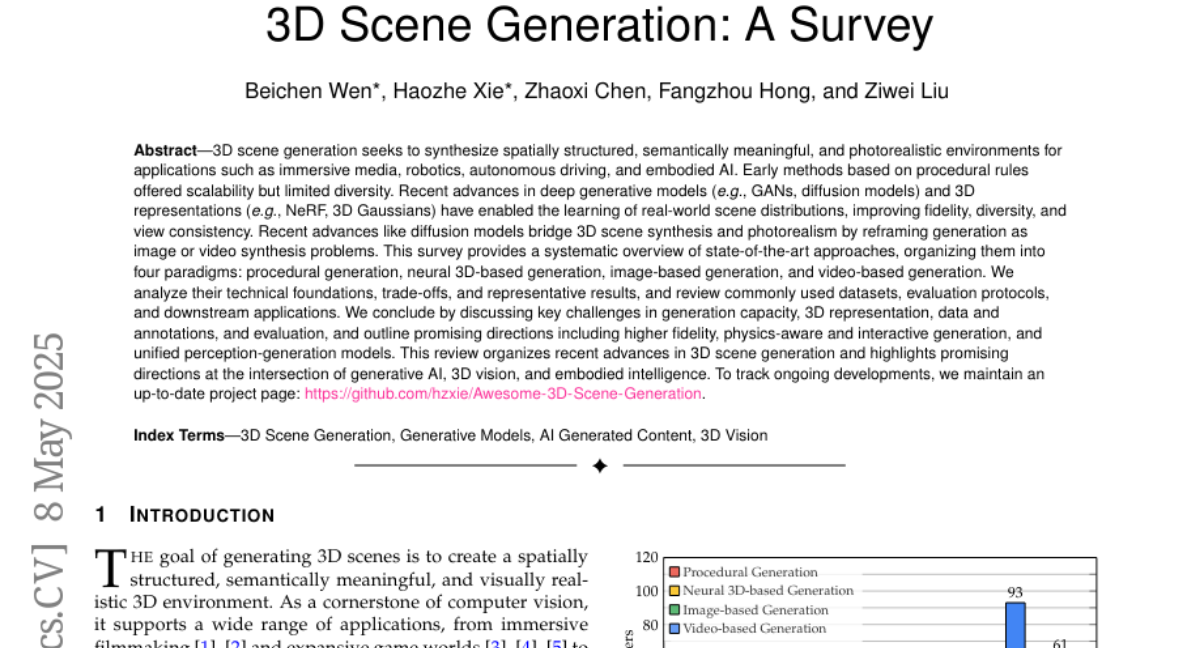
8. ICon: In-Context Contribution for Automatic Data Selection
๐ Keywords: In-context Learning, ICon, Large Language Models, Gradient-Free, Data Selection
๐ก Category: Natural Language Processing
๐ Research Objective:
– The study aims to improve the performance of Large Language Models (LLMs) through effective data selection methods for instruction tuning.
๐ ๏ธ Research Methods:
– Introduces ICon, a novel gradient-free method leveraging in-context learning to measure sample contribution without computationally expensive gradient measures or manual heuristic engineering.
– Evaluates ICon across three LLMs using 12 benchmarks and 5 pairwise evaluation sets.
๐ฌ Research Conclusions:
– ICon provided a computationally efficient alternative and outperformed existing methods by selecting high-contribution data, with models demonstrating significant performance improvements.
– The data selected by ICon encompasses diverse tasks and appropriate difficulty levels, leading to better model performance.
๐ Paper link: https://huggingface.co/papers/2505.05327
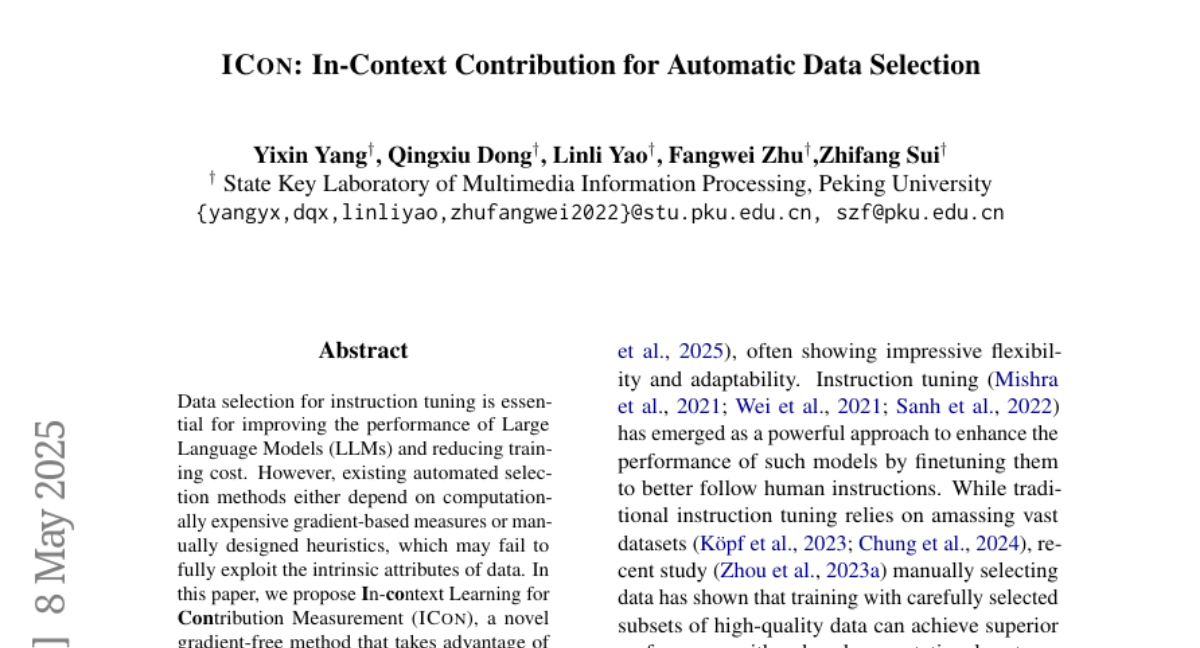
9. X-Reasoner: Towards Generalizable Reasoning Across Modalities and Domains
๐ Keywords: Multimodal reasoning, X-Reasoner, Vision-language model, Reinforcement learning, Generalizable reasoning
๐ก Category: Multi-Modal Learning
๐ Research Objective:
– The paper investigates if reasoning capabilities can be generalized across different modalities and domains.
๐ ๏ธ Research Methods:
– The study introduces X-Reasoner, a vision-language model, using a two-stage post-training approach: supervised fine-tuning with long chain-of-thoughts and reinforcement learning with verifiable rewards.
๐ฌ Research Conclusions:
– X-Reasoner demonstrates superior reasoning transferability in multimodal and out-of-domain settings, outperforming state-of-the-art models. Its specialized variant, X-Reasoner-Med, sets a new benchmark in medical domains.
๐ Paper link: https://huggingface.co/papers/2505.03981
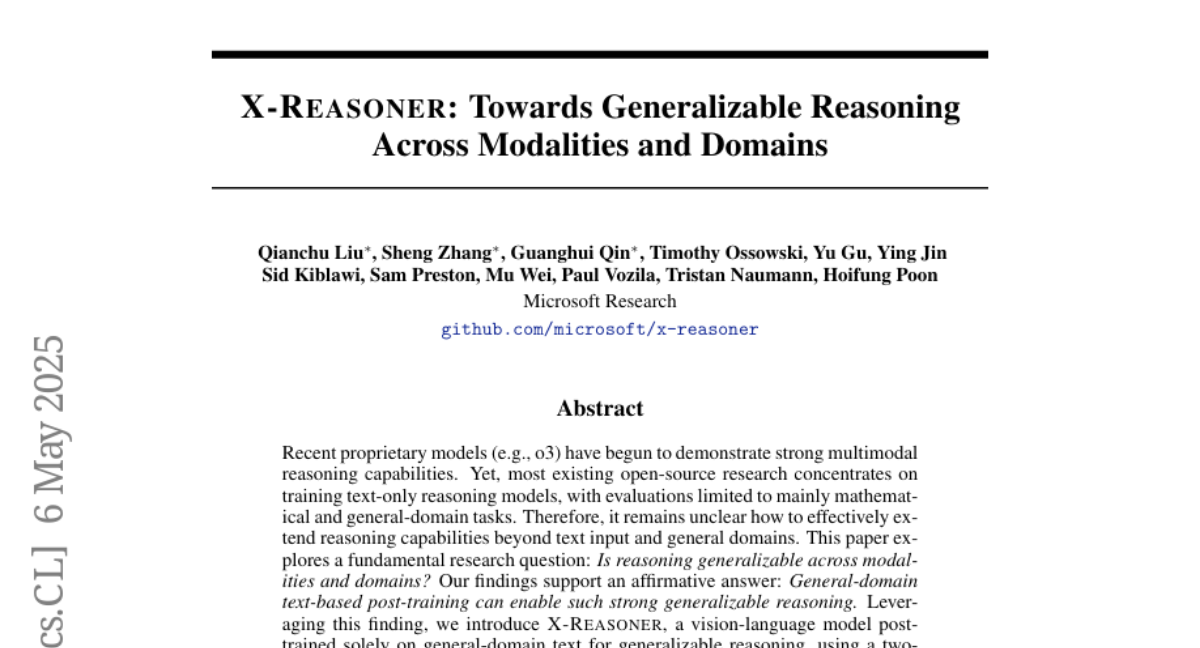
10. StreamBridge: Turning Your Offline Video Large Language Model into a Proactive Streaming Assistant
๐ Keywords: Video-LLMs, streaming-capable models, multi-turn real-time understanding, proactive response mechanisms, memory buffer
๐ก Category: Multi-Modal Learning
๐ Research Objective:
– StreamBridge aims to transform offline Video-LLMs into streaming-capable models by addressing real-time understanding and proactive response challenges.
๐ ๏ธ Research Methods:
– Integrates a memory buffer with a round-decayed compression strategy for long-context interactions and introduces a lightweight activation model for proactive responses.
– Constructs Stream-IT, a dataset for streaming video understanding with video-text sequences and diverse instruction formats.
๐ฌ Research Conclusions:
– StreamBridge significantly enhances streaming understanding capabilities, outperforming proprietary models like GPT-4o and Gemini 1.5 Pro, while maintaining competitive performance on standard benchmarks.
๐ Paper link: https://huggingface.co/papers/2505.05467
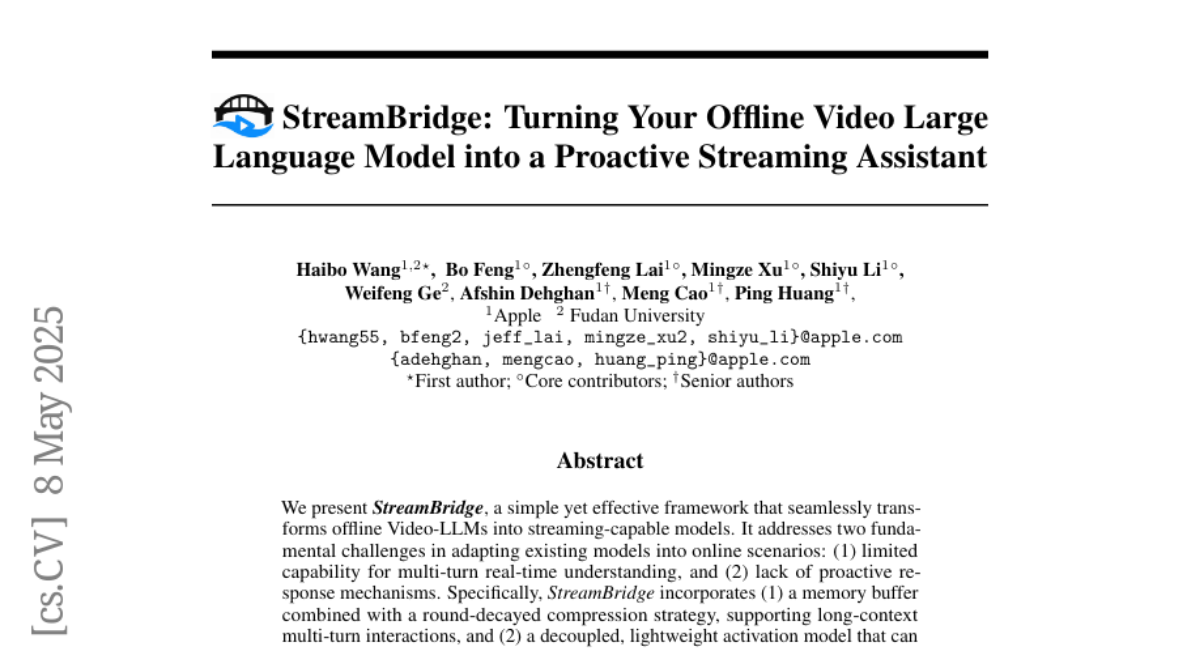
11. Generating Physically Stable and Buildable LEGO Designs from Text
๐ Keywords: LegoGPT, autoregressive large language model, physics laws, robotic arms, StableText2Lego
๐ก Category: Generative Models
๐ Research Objective:
– Introduction of LegoGPT, a system designed to generate physically stable LEGO models from text prompts.
๐ ๏ธ Research Methods:
– Utilized a large dataset of LEGO designs and trained an autoregressive large language model with techniques like next-token prediction, validity checks, and physics-aware rollback.
๐ฌ Research Conclusions:
– LegoGPT successfully produces stable, diverse LEGO designs, which can be assembled both manually and by robotic arms. The new dataset, StableText2Lego, has been released to support further research.
๐ Paper link: https://huggingface.co/papers/2505.05469
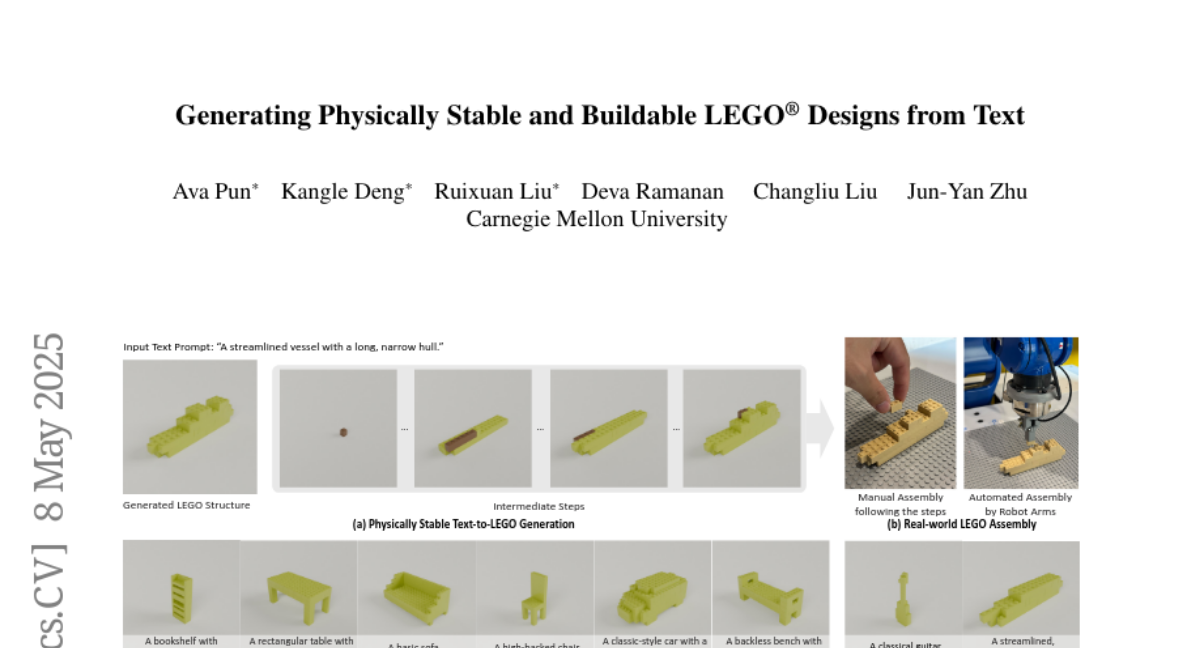
12. LiftFeat: 3D Geometry-Aware Local Feature Matching
๐ Keywords: SLAM, LiftFeat, 3D geometric feature, monocular depth estimation model, visual localization
๐ก Category: Computer Vision
๐ Research Objective:
– To enhance local feature matching robustness in scenarios with lighting changes and low-texture areas by developing a lightweight network called LiftFeat.
๐ ๏ธ Research Methods:
– Utilized a pre-trained monocular depth estimation model to predict pseudo surface normal labels.
– Designed a 3D geometry-aware feature lifting module to integrate surface normal features with 2D descriptors.
๐ฌ Research Conclusions:
– LiftFeat significantly improves the discriminative ability of 2D features in extreme conditions, outperforming state-of-the-art lightweight methods in tasks like pose and homography estimation.
๐ Paper link: https://huggingface.co/papers/2505.03422
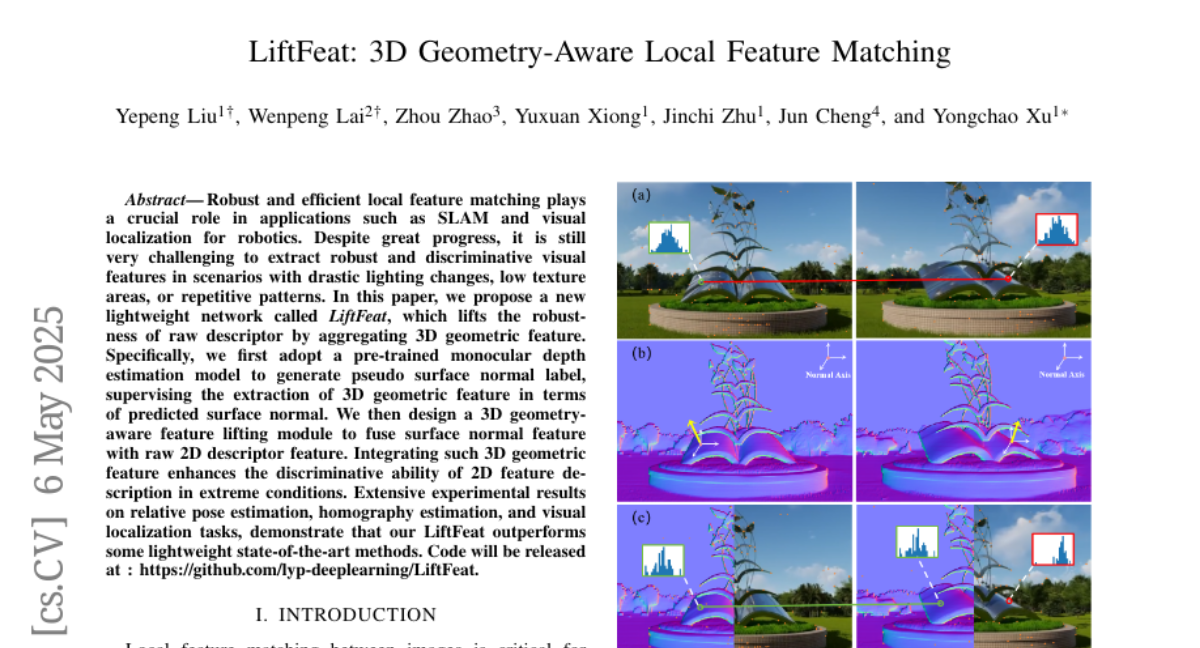
13. WaterDrum: Watermarking for Data-centric Unlearning Metric
๐ Keywords: Large Language Model, Unlearning, WaterDrum, Text Watermarking, AI Ethics
๐ก Category: Natural Language Processing
๐ Research Objective:
– To present a novel data-centric unlearning metric for Large Language Models (LLMs) that addresses current limitations by leveraging text watermarking.
๐ ๏ธ Research Methods:
– Development and application of WaterDrum, a robust text watermarking technique as a new metric for unlearning in LLMs.
– Introduction of benchmark datasets with varying levels of data similarity to test unlearning algorithms.
๐ฌ Research Conclusions:
– The paper introduces WaterDrum, a pioneering approach to improving LLM unlearning evaluation, and provides public access to both the code and benchmark datasets, enhancing the evaluative process for unlearning in LLMs.
๐ Paper link: https://huggingface.co/papers/2505.05064
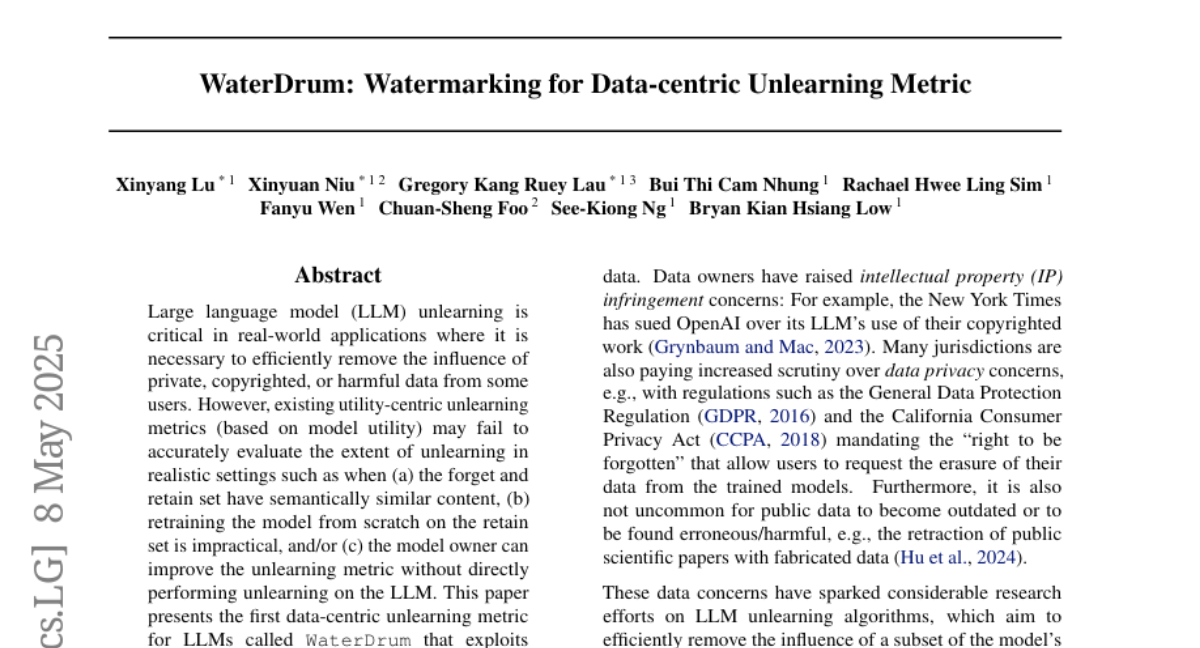
14. PlaceIt3D: Language-Guided Object Placement in Real 3D Scenes
๐ Keywords: Language-Guided Object Placement, 3D Asset, 3D LLMs, Benchmark, Point Cloud
๐ก Category: Computer Vision
๐ Research Objective:
– Introduce the novel task of Language-Guided Object Placement in real 3D scenes, requiring the placement of a 3D asset based on a textual prompt.
๐ ๏ธ Research Methods:
– Proposal of a new benchmark and evaluation protocol and development of a new dataset for training 3D LLMs.
๐ฌ Research Conclusions:
– The introduction of this task and its benchmark could become integral in evaluating and comparing generalist 3D LLM models.
๐ Paper link: https://huggingface.co/papers/2505.05288
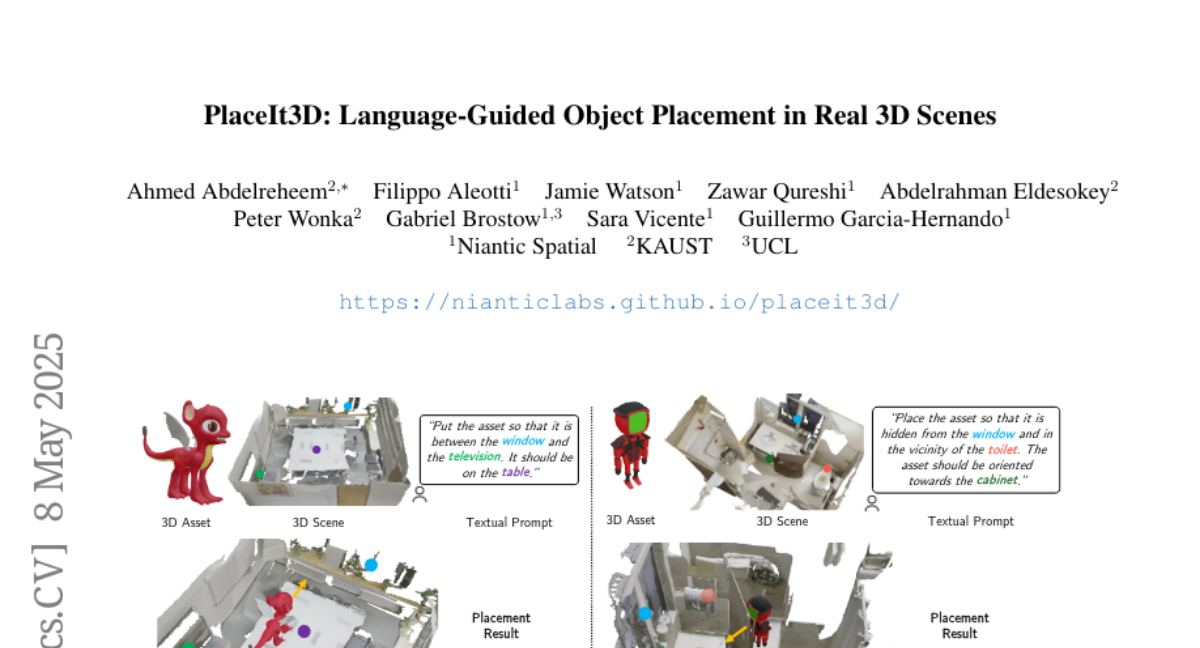
15. Crosslingual Reasoning through Test-Time Scaling
๐ Keywords: English-centric reasoning language models, chain-of-thoughts, multilingual mathematical reasoning, low-resource languages, crosslingual generalization
๐ก Category: Natural Language Processing
๐ Research Objective:
– To investigate the extent to which English reasoning finetuning with long chain-of-thoughts (CoTs) can generalize across different languages.
๐ ๏ธ Research Methods:
– Scaling up inference compute for English-centric reasoning language models (RLMs) and analyzing their multilingual mathematical reasoning capabilities.
– Examining the CoT patterns and the effectiveness of controlling the language of reasoning.
๐ฌ Research Conclusions:
– English-centric RLMs show improved reasoning in multiple languages, outperforming larger models, especially in high-resource languages.
– Ineffective reasoning is observed in low-resource languages and across out-of-domain contexts, highlighting the need for further development in these areas.
๐ Paper link: https://huggingface.co/papers/2505.05408
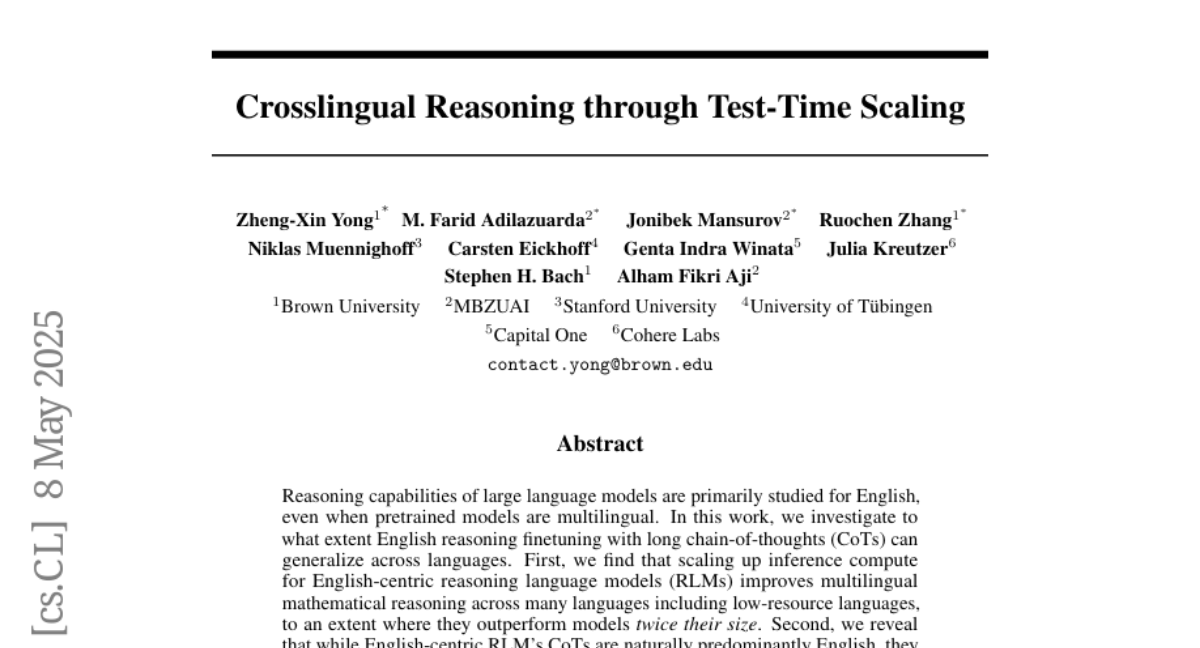
16. BrowseComp-ZH: Benchmarking Web Browsing Ability of Large Language Models in Chinese
๐ Keywords: Large Language Models, Real-Time Web Browsing, Information Ecosystems, Reasoning and Retrieval, Chinese Web
๐ก Category: Natural Language Processing
๐ Research Objective:
– This study aims to bridge the gap in existing benchmarks by introducing BrowseComp-ZH, a high-difficulty benchmark designed to evaluate large language models (LLMs) on the Chinese web.
๐ ๏ธ Research Methods:
– The study employs a two-stage quality control protocol to construct BrowseComp-ZH, consisting of 289 multi-hop questions across 11 domains, designed to challenge the reasoning and retrieval capabilities of LLMs.
๐ฌ Research Conclusions:
– The benchmark reveals that most state-of-the-art models struggle with BrowseComp-ZH, as demonstrated by low accuracy rates, highlighting the need for improved retrieval strategies and advanced reasoning to succeed with this dataset. The best performance was 42.9% accuracy, achieved by OpenAI’s DeepResearch.
๐ Paper link: https://huggingface.co/papers/2504.19314
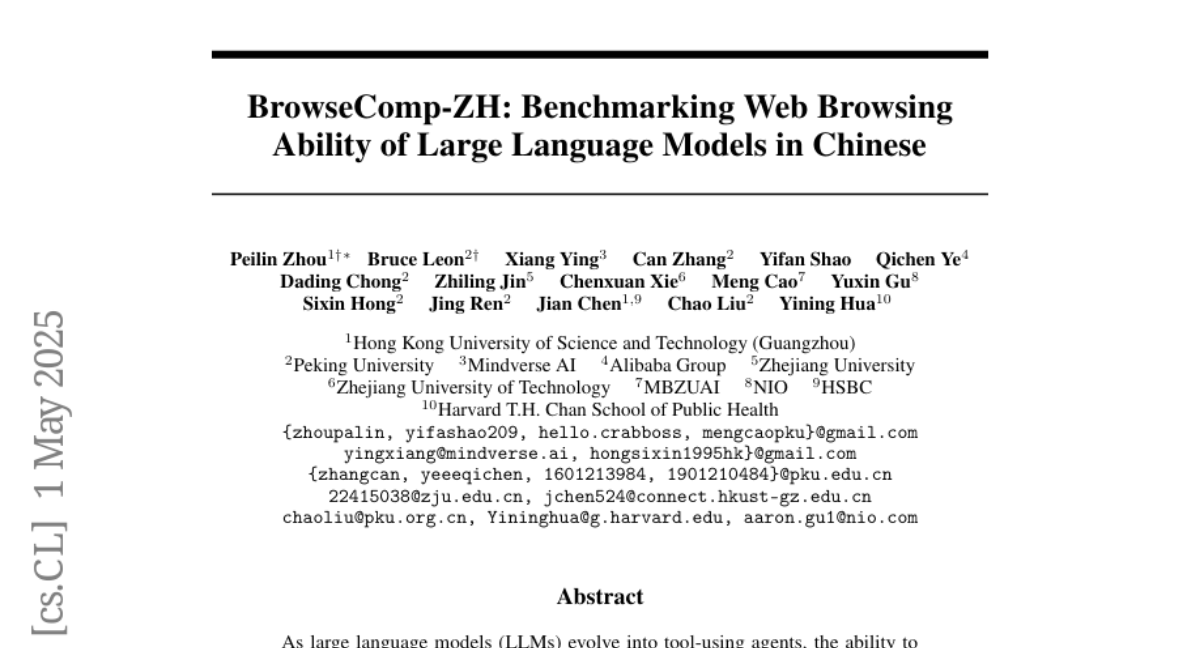
17. Putting the Value Back in RL: Better Test-Time Scaling by Unifying LLM Reasoners With Verifiers
๐ Keywords: Reinforcement Learning, Value Function, Generative Verifier, Test-time Compute Scaling
๐ก Category: Reinforcement Learning
๐ Research Objective:
– To enhance reinforcement learning for large language models by integrating generative verification, improving efficiency and accuracy.
๐ ๏ธ Research Methods:
– Proposing RL^V, which augments any “value-free” RL method through joint training of both a reasoner and a generative verifier using RL-generated data.
๐ฌ Research Conclusions:
– RL^V significantly boosts MATH accuracy by over 20% and enables highly efficient test-time compute scaling, demonstrating strong generalization across various tasks.
๐ Paper link: https://huggingface.co/papers/2505.04842
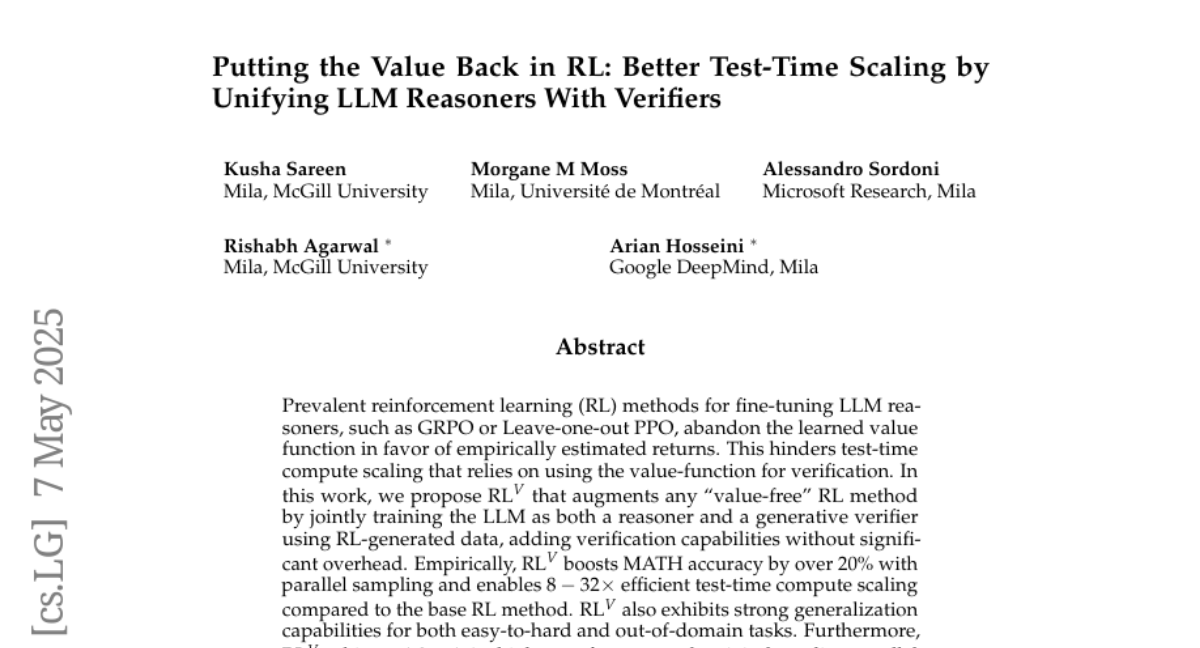
18. SIMPLEMIX: Frustratingly Simple Mixing of Off- and On-policy Data in Language Model Preference Learning
๐ Keywords: Language models, Preference optimization, On-policy data, Off-policy data, SIMPLEMIX
๐ก Category: Natural Language Processing
๐ Research Objective:
– This study aims to explore the complementary strengths of on-policy and off-policy data in optimizing preferences for language models.
๐ ๏ธ Research Methods:
– The introduction of SIMPLEMIX, a method that combines on-policy and off-policy preference learning data to harness their respective strengths.
๐ฌ Research Conclusions:
– SIMPLEMIX significantly enhances language model alignment, improving results on benchmarks like Alpaca Eval 2.0 and outperforming more complex methods such as HyPO and DPO-Mix-P by notable margins.
๐ Paper link: https://huggingface.co/papers/2505.02363
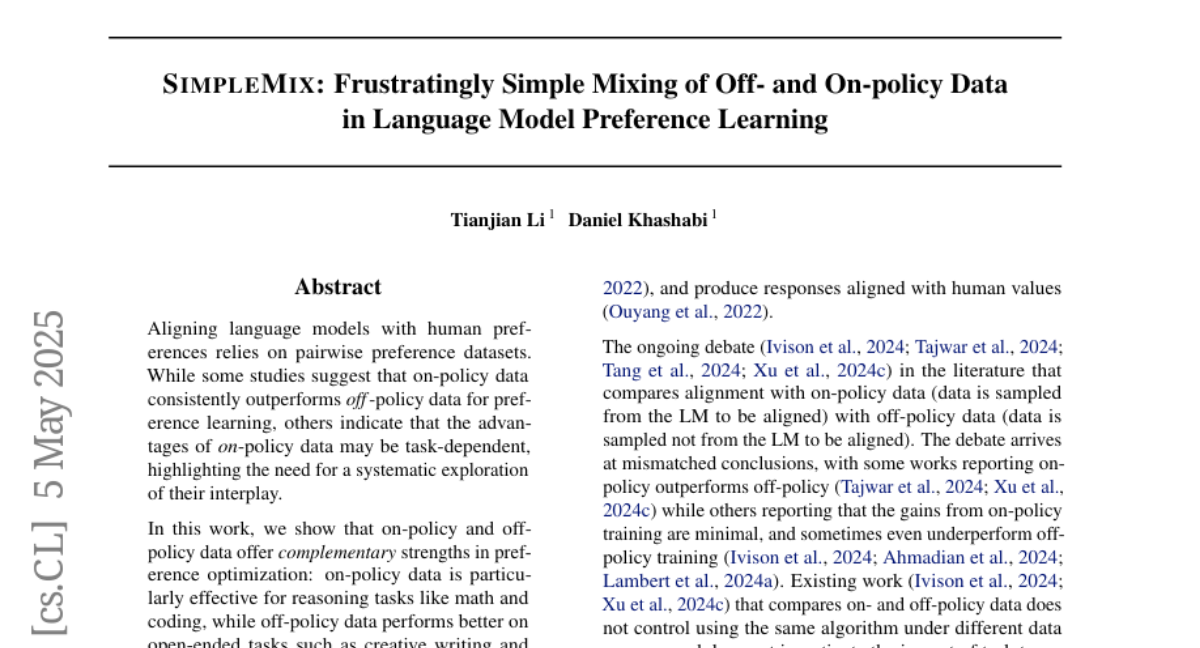
19. Vision-Language-Action Models: Concepts, Progress, Applications and Challenges
๐ Keywords: Vision-language-action, Vision-language Models, Agentic AI, Ethical Deployment Risks
๐ก Category: Robotics and Autonomous Systems
๐ Research Objective:
– The paper aims to unify perception, natural language understanding, and embodied action through Vision-Language-Action models, transforming AI capabilities.
๐ ๏ธ Research Methods:
– The authors employ a comprehensive literature review of over 80 VLA models, examining advancements like architectural innovations and parameter-efficient training strategies.
๐ฌ Research Conclusions:
– The study identifies key progress and challenges in the field, proposing solutions such as agentic AI adaptation and cross-embodiment generalization for future advancements in intelligent robotics and artificial general intelligence.
๐ Paper link: https://huggingface.co/papers/2505.04769
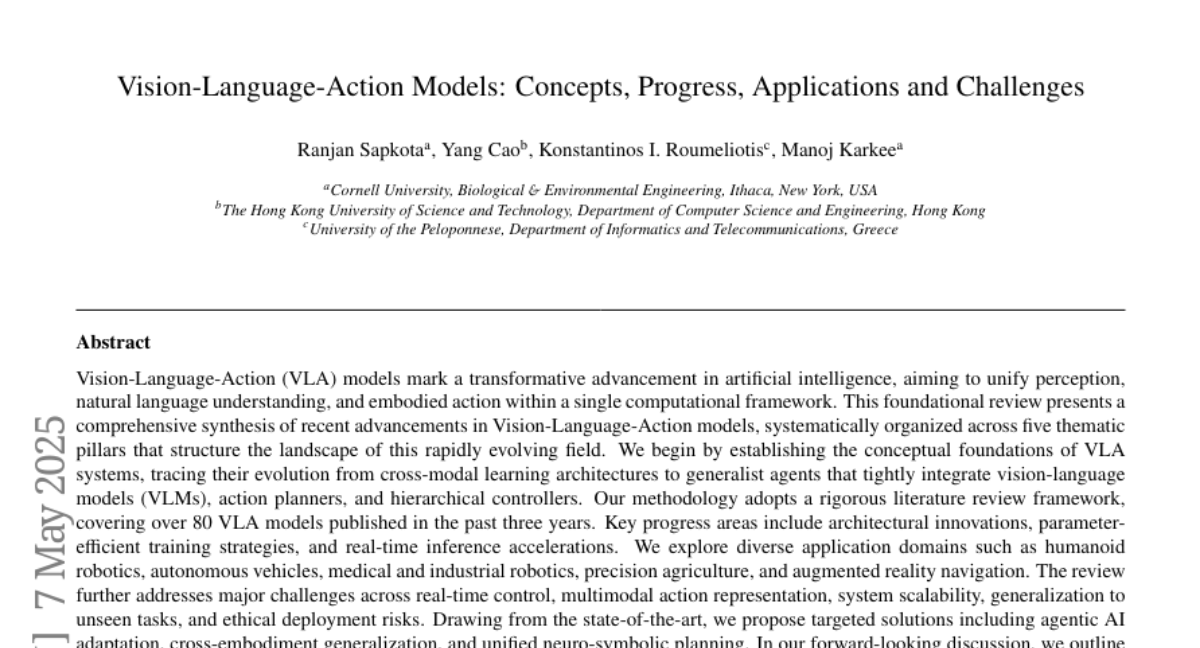
20. Chain-of-Thought Tokens are Computer Program Variables
๐ Keywords: Chain-of-thoughts, Large Language Models, Intermediate Steps, Compositional Tasks, Variables
๐ก Category: Knowledge Representation and Reasoning
๐ Research Objective:
– To empirically study the role of Chain-of-thoughts (CoT) tokens in Large Language Models (LLMs) on solving compositional tasks.
๐ ๏ธ Research Methods:
– Analyzed the performance of LLMs on multi-digit multiplication and dynamic programming by examining CoT tokens and their storage forms.
๐ฌ Research Conclusions:
– Preserving only the tokens that store intermediate results achieves comparable performance to full CoT.
– Storing results in alternative latent forms does not affect performance.
– CoT tokens may function like variables with potential drawbacks such as unintended shortcuts and computational complexity limits.
๐ Paper link: https://huggingface.co/papers/2505.04955
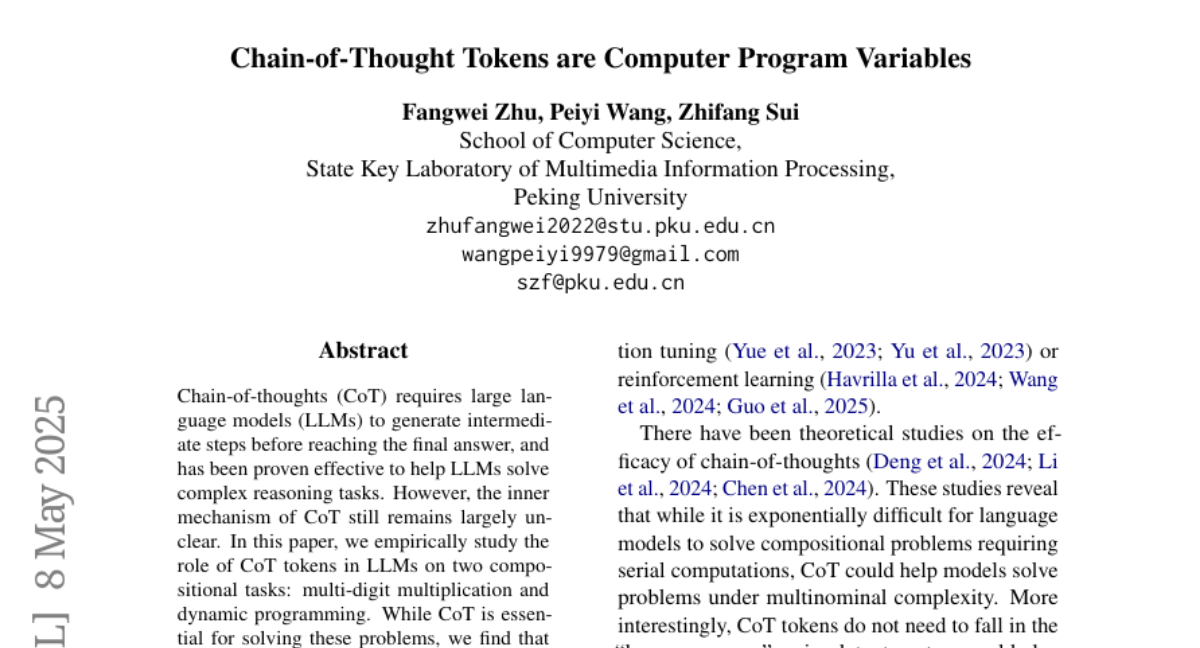
21.
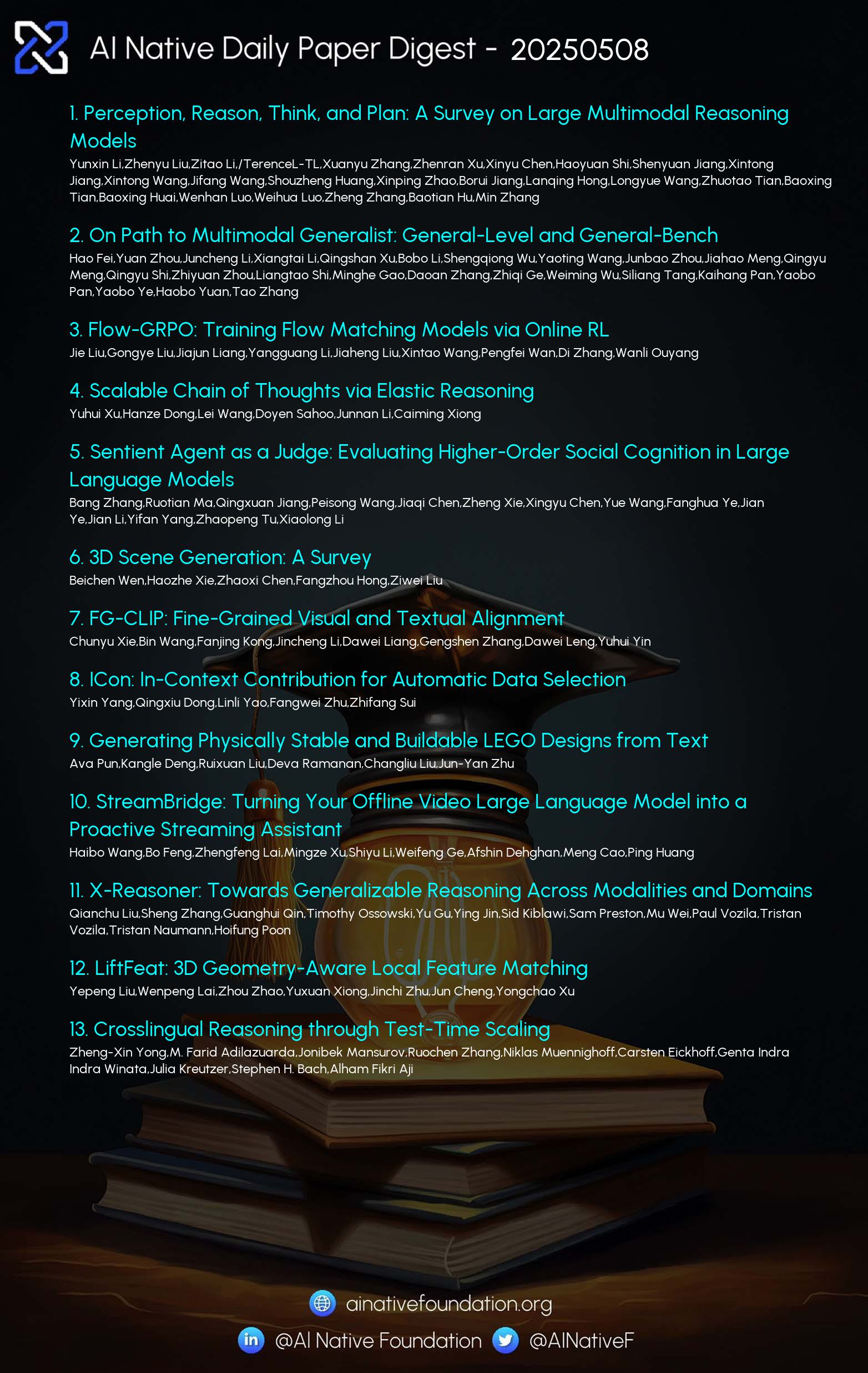